from sklearn.preprocessing import StandardScaler from transformers import pipeline #一个高级API,用于快速使用预训练的模型进行自然语言处理任务。 # 数据预处理 scaler = StandardScaler() merged_train_norm_vec = scaler.fit_transform(merged_train_norm_vec) # 参数设置 param_grid = {'alpha': [0.0001, 0.001, 0.01], 'max_iter': [100, 500, 1000], 'tol': [0.001, 0.01, 0.1]} # 模型构建 #Pipeline可以方便地对整个流程进行交叉验证和调参 #Pipeline来构建模型,使用StandardScaler进行数据预处理,使用更加全面和细致的参数设置 # model = Pipeline([('scaler', scaler), ('Perceptron', Perceptron())]) # 交叉验证 k = 10 cv = StratifiedKFold(n_splits=k, shuffle=True) # 网格搜索 grid = GridSearchCV(model, param_grid, cv=cv, scoring='accuracy') grid.fit(merged_train_norm_vec, y_merged_train) # 输出最优参数和分类准确率 print('Best params:', grid.best_params_) print('Best score:', grid.best_score_) NameError: name 'Pipeline' is not defined怎么解决
时间: 2024-03-16 18:46:06 浏览: 91
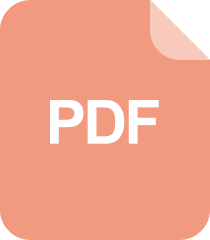
用sklearn进行数据预处理:缺失值处理、数据标准化、归一化.pdf
您需要导入 Pipeline 类,可以将以下代码添加到您的代码顶部:
```
from sklearn.pipeline import Pipeline
```
这将从 scikit-learn 库中导入 Pipeline 类。现在您可以使用它来构建模型,例如:
```
model = Pipeline([('scaler', scaler), ('Perceptron', Perceptron())])
```
请注意,这里使用了 sklearn.pipeline 中的 Pipeline 类,而不是 Hugging Face Transformers 库中的 Pipeline 类。这两个类是不同的,用于不同的用途。
阅读全文
相关推荐
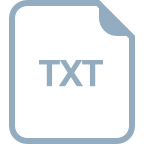
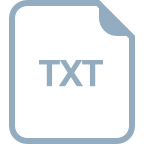
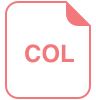
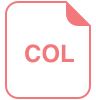
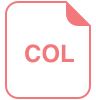
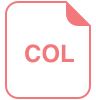
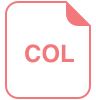
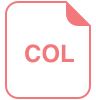
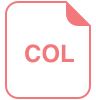
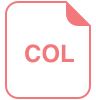
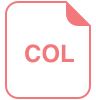
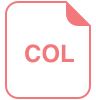
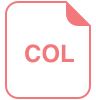
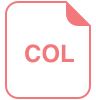
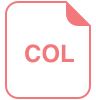
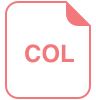

