基于图像特征的模板匹配模板匹配为基于欧式距离的模板匹配
时间: 2023-12-17 16:04:37 浏览: 71
是的,基于图像特征的模板匹配和基于欧式距离的模板匹配是两种不同的模板匹配方法。基于图像特征的模板匹配是通过提取图像中的特征,例如边缘、角点等,然后将这些特征与模板进行匹配,以确定最佳匹配位置。而基于欧式距离的模板匹配则是通过计算图像中像素值之间的欧式距离来进行匹配,最佳匹配位置是使得欧式距离最小的位置。两种方法各有优缺点,根据具体应用场景选择不同的方法。
相关问题
基于图像特征的模板匹配模板匹配为基于欧式距离的模板匹配opencv代码
以下是基于图像特征的模板匹配和基于欧式距离的模板匹配的OpenCV代码:
基于图像特征的模板匹配:
```
import cv2
# 读取原始图像和模板
img = cv2.imread('image.jpg', 0)
template = cv2.imread('template.jpg', 0)
# 提取图像特征
sift = cv2.xfeatures2d.SIFT_create()
kp1, des1 = sift.detectAndCompute(img, None)
kp2, des2 = sift.detectAndCompute(template, None)
# 创建FLANN匹配器
FLANN_INDEX_KDTREE = 0
indexParams = dict(algorithm=FLANN_INDEX_KDTREE, trees=5)
searchParams = dict(checks=50)
flann = cv2.FlannBasedMatcher(indexParams, searchParams)
# 匹配特征
matches = flann.knnMatch(des1, des2, k=2)
# 提取良好的匹配
good_matches = []
for m, n in matches:
if m.distance < 0.7 * n.distance:
good_matches.append(m)
# 绘制匹配结果
img_matches = cv2.drawMatches(img, kp1, template, kp2, good_matches, None, flags=2)
cv2.imshow('matches', img_matches)
cv2.waitKey(0)
cv2.destroyAllWindows()
```
基于欧式距离的模板匹配:
```
import cv2
# 读取原始图像和模板
img = cv2.imread('image.jpg', 0)
template = cv2.imread('template.jpg', 0)
# 获取模板大小
h, w = template.shape
# 模板匹配
res = cv2.matchTemplate(img, template, cv2.TM_SQDIFF)
# 获取最佳匹配位置
min_val, max_val, min_loc, max_loc = cv2.minMaxLoc(res)
top_left = min_loc
bottom_right = (top_left[0] + w, top_left[1] + h)
# 绘制匹配结果
img_matches = cv2.rectangle(img, top_left, bottom_right, 255, 2)
cv2.imshow('matches', img_matches)
cv2.waitKey(0)
cv2.destroyAllWindows()
```
需要注意的是,以上代码仅为示例代码,实际应用中需要根据具体情况进行调整和优化。
在灰度图像的基础上,在灰度图截取一段区域,大小nn,提取这个区域的特征值当作模板, 2. 以nn图象块的方式遍历图像,分别提取每个图像块的特征值,并通过基于模板的特征匹配来输出相似图像块的区域,模板匹配为基于欧式距离的模板匹配。c++代码
以下是一个基于OpenCV库实现的基于欧式距离模板匹配的图像匹配C++代码示例,其中包含截取区域、提取特征值、以及基于模板的特征匹配:
```c++
#include <opencv2/opencv.hpp>
using namespace cv;
int main()
{
// 读取图像
Mat img = imread("image.jpg", IMREAD_GRAYSCALE);
Mat templateImg = img(Rect(50, 50, 100, 100)); // 截取区域
int h = templateImg.rows;
int w = templateImg.cols;
// 提取模板特征值
Mat templateFeature;
reduce(templateImg, templateFeature, 1, REDUCE_AVG);
// 以nn图像块的方式遍历图像
int nn = 10;
int step = nn / 2;
for (int i = step; i < img.rows - step; i += step)
{
for (int j = step; j < img.cols - step; j += step)
{
// 提取图像块特征值
Mat imgBlock = img(Rect(j - step, i - step, nn, nn));
Mat imgBlockFeature;
reduce(imgBlock, imgBlockFeature, 1, REDUCE_AVG);
// 基于模板的特征匹配
double euclideanDist = norm(templateFeature, imgBlockFeature, NORM_L2); // 计算欧式距离
if (euclideanDist < 10) // 设置阈值
{
rectangle(img, Point(j - step, i - step), Point(j + step, i + step), Scalar(255, 255, 255), 2); // 输出相似图像块的区域
}
}
}
// 显示图像
imshow("Result", img);
waitKey(0);
destroyAllWindows();
return 0;
}
```
这段代码实现了截取区域、提取特征值、以及基于模板的特征匹配功能。其中,使用了OpenCV库的reduce函数对图像块进行特征值提取,使用了norm函数计算欧式距离,并通过设定阈值来判断是否为相似图像块。
阅读全文
相关推荐
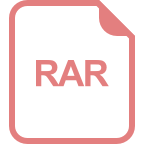
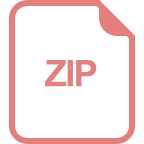
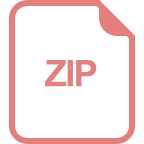
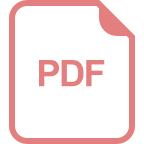
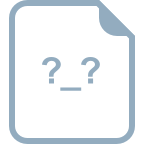
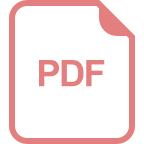
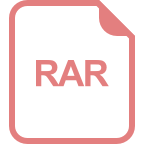
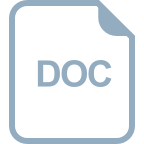
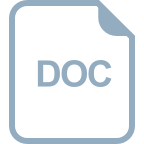
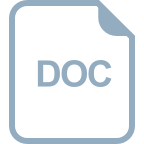
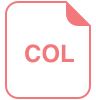
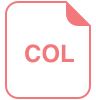

