用python写一个杂草识别代码
时间: 2024-05-30 19:12:28 浏览: 112
好的,关于杂草识别方面的问题,我可以尝试回答。但需要说明的是,AI只能提供建议和参考,最终决定还需要由人类专业人士进行判断和核实。
首先,杂草识别可以基于图像识别技术,具体的实现可以借助Python的机器学习框架,例如Tensorflow、Keras等。
以下是可能的步骤:
1. 收集标注好的杂草图片数据集,其中包括杂草的不同种类和形态。
2. 对图片进行预处理,如大小归一化、色彩空间转换等。
3. 基于卷积神经网络(CNN)模型进行训练,识别并分类图像中的各种杂草。
4. 对新的图片数据进行测试,利用模型进行预测和识别。
以上是基于机器学习的方法,当然也可以利用传统的计算机视觉技术、图像处理技术进行实现。
希望以上内容对您有帮助。
相关问题
帮我用Python写一个能识别杂草的代码
很抱歉,识别杂草是一个非常困难的任务,因为它需要对图像进行复杂的识别和分类。然而,您可以使用机器学习技术来训练一个模型,该模型可以识别杂草。
首先,您需要准备大量关于杂草的图像数据,并将它们分成训练集和测试集。然后,您可以使用一种称为卷积神经网络(CNN)的模型来训练数据。最后,您可以使用该模型对新的图像进行分类,以识别杂草。
以下是使用TensorFlow和Keras框架的示例代码:
```
import tensorflow as tf
from tensorflow import keras
# Load the data
(x_train, y_train), (x_test, y_test) = keras.datasets.cifar10.load_data()
# Normalize the data
x_train = x_train / 255.0
x_test = x_test / 255.0
# Build the model
model = keras.Sequential([
keras.layers.Conv2D(32, (3, 3), padding='same', activation='relu', input_shape=(32, 32, 3)),
keras.layers.MaxPooling2D((2, 2)),
keras.layers.Conv2D(64, (3, 3), padding='same', activation='relu'),
keras.layers.MaxPooling2D((2, 2)),
keras.layers.Flatten(),
keras.layers.Dense(128, activation='relu'),
keras.layers.Dense(10, activation='softmax')
])
# Compile the model
model.compile(optimizer='adam',
loss='sparse_categorical_crossentropy',
metrics=['accuracy'])
# Train the model
model.fit(x_train, y_train, epochs=10, validation_data=(x_test, y_test))
# Evaluate the model
test_loss, test_acc = model.evaluate(x_test, y_test, verbose=
用TensorFlow写一个杂草识别的代码
以下是一个基于 TensorFlow 的杂草识别代码:
```python
import tensorflow as tf
import numpy as np
import os
# 设置图片大小和训练批次数
IMAGE_SIZE = 128
BATCH_SIZE = 32
# 定义数据集路径
TRAIN_PATH = 'train'
TEST_PATH = 'test'
# 定义标签列表
LABELS = ['dandelion', 'grass', 'lawn', 'weed']
# 将标签转换为数字
label_dict = {label:i for i, label in enumerate(LABELS)}
# 定义读取图片函数
def read_image(file_path):
image = tf.io.read_file(file_path)
image = tf.image.decode_jpeg(image, channels=3)
image = tf.image.resize(image, [IMAGE_SIZE, IMAGE_SIZE])
image = tf.cast(image, tf.float32)
image /= 255.0
return image
# 定义读取数据集函数
def load_dataset(data_path):
images = []
labels = []
for label in LABELS:
path = os.path.join(data_path, label)
files = os.listdir(path)
for file in files:
if file.endswith('.jpg'):
image_path = os.path.join(path, file)
image = read_image(image_path)
images.append(image)
labels.append(label_dict[label])
return tf.data.Dataset.from_tensor_slices((images, labels))
# 加载训练集和测试集
train_dataset = load_dataset(TRAIN_PATH).shuffle(10000).batch(BATCH_SIZE)
test_dataset = load_dataset(TEST_PATH).batch(BATCH_SIZE)
# 定义模型
model = tf.keras.Sequential([
tf.keras.layers.Conv2D(32, (3, 3), activation='relu', input_shape=(IMAGE_SIZE, IMAGE_SIZE, 3)),
tf.keras.layers.MaxPooling2D((2, 2)),
tf.keras.layers.Conv2D(64, (3, 3), activation='relu'),
tf.keras.layers.MaxPooling2D((2, 2)),
tf.keras.layers.Conv2D(128, (3, 3), activation='relu'),
tf.keras.layers.MaxPooling2D((2, 2)),
tf.keras.layers.Flatten(),
tf.keras.layers.Dense(128, activation='relu'),
tf.keras.layers.Dense(len(LABELS))
])
# 定义损失函数和优化器
loss_fn = tf.keras.losses.SparseCategoricalCrossentropy(from_logits=True)
optimizer = tf.keras.optimizers.Adam()
# 定义评估指标
train_loss = tf.keras.metrics.Mean(name='train_loss')
train_accuracy = tf.keras.metrics.SparseCategoricalAccuracy(name='train_accuracy')
test_loss = tf.keras.metrics.Mean(name='test_loss')
test_accuracy = tf.keras.metrics.SparseCategoricalAccuracy(name='test_accuracy')
# 定义训练函数
@tf.function
def train_step(images, labels):
with tf.GradientTape() as tape:
predictions = model(images, training=True)
loss = loss_fn(labels, predictions)
gradients = tape.gradient(loss, model.trainable_variables)
optimizer.apply_gradients(zip(gradients, model.trainable_variables))
train_loss(loss)
train_accuracy(labels, predictions)
# 定义测试函数
@tf.function
def test_step(images, labels):
predictions = model(images, training=False)
t_loss = loss_fn(labels, predictions)
test_loss(t_loss)
test_accuracy(labels, predictions)
# 训练模型
epochs = 10
for epoch in range(epochs):
train_loss.reset_states()
train_accuracy.reset_states()
test_loss.reset_states()
test_accuracy.reset_states()
for images, labels in train_dataset:
train_step(images, labels)
for images, labels in test_dataset:
test_step(images, labels)
template = 'Epoch {}, Loss: {}, Accuracy: {}, Test Loss: {}, Test Accuracy: {}'
print(template.format(epoch+1,
train_loss.result(),
train_accuracy.result()*100,
test_loss.result(),
test_accuracy.result()*100))
```
该代码使用了一个简单的卷积神经网络来进行杂草识别任务。首先,定义了一个 `read_image` 函数来读取图片,并将其转换为 `tf.Tensor` 类型。然后,定义了一个 `load_dataset` 函数来将数据集加载到 `tf.data.Dataset` 类型中。接着,定义了一个简单的卷积神经网络模型,使用了 `tf.keras` 的 Sequential API 来构建。最后,定义了训练函数和测试函数,并在训练过程中输出训练集和测试集的损失和准确率。
阅读全文
相关推荐
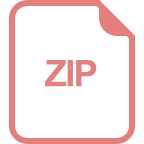
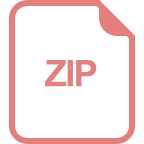
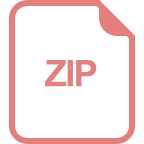

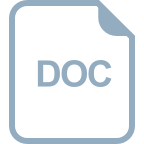
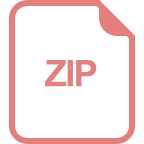



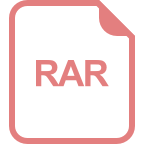
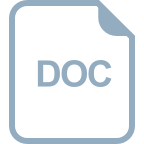




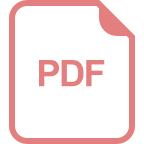