lm.fit <- lm(y~.,data = transformed_data) summary(lm.fit)
时间: 2024-04-26 16:20:28 浏览: 107
这段代码使用 R 语言中的线性回归方法,拟合经过主成分分析降维后的数据,并输出回归分析结果的摘要信息。具体来说,它使用 lm 函数对 transformed_data 数据框中的所有自变量(除了第一列外的所有列)与第一列(因变量)进行线性回归,将结果存储在 lm.fit 变量中。其中, ~ 表示左侧的变量作为因变量,右侧的变量作为自变量。参数 data = transformed_data 表示使用 transformed_data 数据框进行回归分析。最后,使用 summary 函数输出回归分析结果的摘要信息,包括回归系数、截距、标准误、置信区间、显著性水平、残差标准差、多重共线性等信息。
相关问题
lm.fit <- lm(y~.,data= transformed_data) summary(lm.fit
这段代码的功能是对经过 PCA 转换后的数据进行线性回归分析,其中 y 是因变量,其余变量为自变量。lm() 函数是用来创建线性模型的,它的第一个参数是一个公式,公式中使用 ~ 符号将因变量和自变量分隔开来,点号表示选择所有的自变量作为解释因变量的因素。summary() 函数用于展示线性模型的结果,包括每个自变量的系数、标准误差、t 值、p 值和 R-squared 等统计信息。
Create a log-linear model m2 with response log(mass_g) (using R’s natural log function log) and regressor SVL_mm.
To create a log-linear model `m2` where the response variable is the natural logarithm of `mass_g` and the regressor is `SVL_mm`, follow these steps:
1. **Transform the Response Variable**: Use R's natural log function `log` to transform the `mass_g` variable.
2. **Build the Model**: Utilize the `lm()` function to build the linear model with the transformed response variable.
Here is the corresponding R code:
```R
# Assuming 'my_lizards' is the dataframe containing the relevant data
m2 <- lm(log(mass_g) ~ SVL_mm, data = my_lizards)
summary(m2) # Display the model summary
```
This code snippet creates the model and displays its summary, providing insights into the coefficients, residuals, and overall model fit.
阅读全文
相关推荐
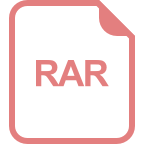
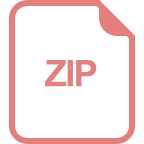
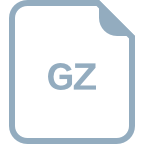
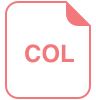
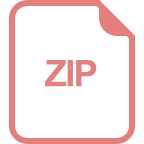