def resize_norm_img(self, img, max_wh_ratio): imgC, imgH, imgW = self.rec_image_shape assert imgC == img.shape[2] imgW = int((32 * max_wh_ratio)) h, w = img.shape[:2] ratio = w / float(h) if math.ceil(imgH * ratio) > imgW: resized_w = imgW else: resized_w = int(math.ceil(imgH * ratio)) resized_image = cv2.resize(img, (resized_w, imgH)) resized_image = resized_image.astype('float32') # [0, 255] -> [0, 1] resized_image = resized_image.transpose((2, 0, 1)) / 255 # [0, 1] -> [-0.5, 0.5] resized_image -= 0.5 # [-0.5, 0.5] -> [-1, 1] resized_image /= 0.5 padding_im = np.zeros((imgC, imgH, imgW), dtype=np.float32) padding_im[:, :, 0:resized_w] = resized_image return padding_im
时间: 2023-06-19 09:06:29 浏览: 196
这段代码是一个OCR识别模型中的图像预处理部分。它的作用是将输入的图像进行缩放、归一化和填充,以适配模型的输入要求。
具体地,它首先确定了输入图像的通道数、高度和宽度,然后根据最大宽高比限制计算出缩放后的宽度。接着,它计算图像的宽高比,并根据它来确定缩放后的高度和宽度。接着,它将缩放后的图像进行归一化,将像素值从[0, 255]映射到[0, 1],然后将像素值从[0, 1]映射到[-0.5, 0.5],最后将像素值从[-0.5, 0.5]映射到[-1, 1]。最后,它将归一化后的图像填充到指定的高度和宽度,以适配模型的输入要求。
这段代码的作用是将输入图像转换为模型可以接受的格式,并且保证图像的信息不会丢失。
相关问题
下面这段代码在做什么? def resize_norm_img(img, max_wh_ratio, rec_image_shape='3,48,320'): rec_image_shape = [int(v) for v in rec_image_shape.split(",")] imgC, imgH, imgW = rec_image_shape assert imgC == img.shape[2] max_wh_ratio = max(max_wh_ratio, imgW / imgH) imgW = int((imgH * max_wh_ratio)) imgW = max(min(imgW, 1280), 16) h, w = img.shape[:2] ratio = w / float(h) ratio_imgH = math.ceil(imgH * ratio) ratio_imgH = max(ratio_imgH, 16) if ratio_imgH > imgW: resized_w = imgW else: resized_w = int(ratio_imgH) resized_image = cv2.resize(img, (resized_w, imgH)) resized_image = resized_image.astype('float32') resized_image = resized_image.transpose((2, 0, 1)) / 255 resized_image -= 0.5 resized_image /= 0.5 padding_im = np.zeros((imgC, imgH, imgW), dtype=np.float32) padding_im[:, :, 0:resized_w] = resized_image return padding_im
这段代码是用来调整图像大小和归一化图像像素值的。函数的输入参数包括原始图片(img)、图像高宽比(max_wh_ratio)、目标图像形状(rec_image_shape)。首先,函数将输入的目标图像形状(rec_image_shape)解析为通道数(imgC)、高(imgH)和宽(imgW)。然后,函数根据图像高宽比(max_wh_ratio)重新计算图像的宽度(imgW),并将其限制在一定的范围内。接下来,函数根据比例计算调整后的图像高度(ratio_imgH)。最后,函数对调整后的图像进行归一化、转置和填充操作,并返回修改后的图像(padding_im)。
程序无法执行,修改class Processor(): def __init__(self): self._inspect_step = int(cfg.get('PROCESS', 'INSPECT_STEP')) def capture_img(self): global aco aco = aco + 1 self._cam.stream_on() raw_image = self._cam.data_stream[0].get_image() if raw_image is None: print("Getting image failed.\n") return None print("Frame ID: {} Height: {} Width: {} Count: {}\n" .format(raw_image.get_frame_id(), raw_image.get_height(), raw_image.get_width(), aco - 2)) numpy_image = raw_image.get_numpy_array() if numpy_image is None: return None img = Image.fromarray(numpy_image, 'L') if self._issave: picfile = '{}/{}.bmp'.format(self._picpath, self._piccount) self._piccount = self._piccount + 1 img.save(picfile) if self._isshow: w, h = img.size scale = min(1.0 * IMG_RESIZE_W / w, 1.0 * IMG_RESIZE_H / h) self._img = ImageTk.PhotoImage(image=img.resize((int(w * scale), int(h * scale)), Image.ANTIALIAS)) self._show_cb(self._img) self._cam.stream_off() return img def process_img(self, img): return PROC_OK def inspect(self): print("process2") time.sleep(1) def rotate(self): count = 0 aco = 0 self.threadsignal = 0 while self._running: v = self._dgl.qpin(ROT) if len(v) > 0: count = count + sum(v) if count > self._inspect_step: aco += 1 img = self.capture_img() count = count - self._inspect_step if __name__ == '__main__': task2 = multiprocessing.Process(target=self.inspect) task2.start() task1 = multiprocessing.Process(target=self.rotate) task1.start()
在这段代码中,有几个问题可以被改进。首先,类 `Processor` 中的全局变量 `aco` 可能会导致并发问题,在多进程环境下应该避免使用全局变量。其次,代码中出现了 `time.sleep()`,这会阻塞当前进程并造成性能瓶颈,应该尽量避免使用。
以下是可能的改进方案:
1. 将 `aco` 变量改为实例变量,避免多进程之间的并发问题。
2. 使用 `Queue` 或其他进程间通信机制替代全局变量,避免多进程之间的竞争。
3. 将 `time.sleep()` 改为使用定时器或者事件,避免阻塞进程并提高程序的性能。
4. 在多进程环境中,使用 `if __name__ == '__main__':` 来避免创建子进程时出现递归调用的问题。
以下是修改后的代码示例:
```python
import multiprocessing
from queue import Queue
import time
class Processor():
def __init__(self):
self._inspect_step = int(cfg.get('PROCESS', 'INSPECT_STEP'))
self._aco = 0
self._queue = Queue()
def capture_img(self):
self._aco += 1
self._cam.stream_on()
raw_image = self._cam.data_stream[0].get_image()
if raw_image is None:
print("Getting image failed.\n")
return None
print("Frame ID: {} Height: {} Width: {} Count: {}\n".format(raw_image.get_frame_id(), raw_image.get_height(), raw_image.get_width(), self._aco - 2))
numpy_image = raw_image.get_numpy_array()
if numpy_image is None:
return None
img = Image.fromarray(numpy_image, 'L')
if self._issave:
picfile = '{}/{}.bmp'.format(self._picpath, self._piccount)
self._piccount = self._piccount + 1
img.save(picfile)
if self._isshow:
w, h = img.size
scale = min(1.0 * IMG_RESIZE_W / w, 1.0 * IMG_RESIZE_H / h)
self._img = ImageTk.PhotoImage(image=img.resize((int(w * scale), int(h * scale)), Image.ANTIALIAS))
self._show_cb(self._img)
self._cam.stream_off()
return img
def process_img(self, img):
return PROC_OK
def inspect(self):
print("process2")
while self._running:
time.sleep(1)
self._queue.put("task")
def rotate(self):
count = 0
while self._running:
v = self._dgl.qpin(ROT)
if len(v) > 0:
count = count + sum(v)
if count > self._inspect_step:
self._aco += 1
img = self.capture_img()
count = count - self._inspect_step
try:
task = self._queue.get(block=False)
if task == "task":
print("processing task")
except:
pass
if __name__ == '__main__':
p = Processor()
p._running = True
task2 = multiprocessing.Process(target=p.inspect)
task2.start()
task1 = multiprocessing.Process(target=p.rotate)
task1.start()
```
请注意,这里只是对原始代码的一些修改建议,并不能保证这样的修改能够完全解决问题。在实际环境中,还需要根据具体情况进行调整和优化。
阅读全文
相关推荐
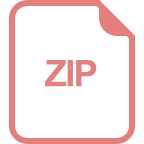
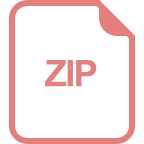
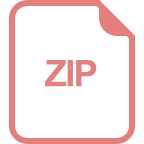
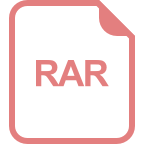
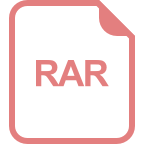
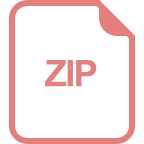
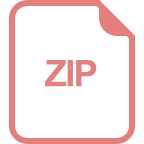
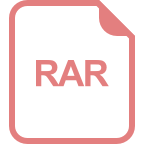
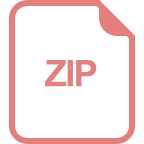
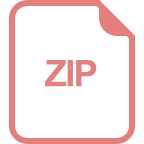
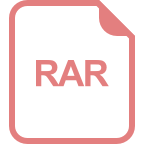
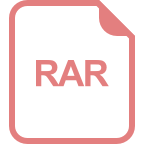
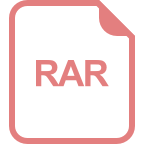
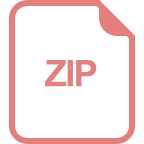
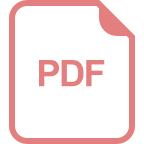
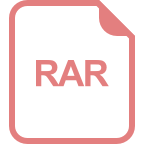