请你帮我用segnet模型实现图像语义分割的全过程代码,包含训练模型、预测新图像,用pytorch包,谢谢
时间: 2023-12-06 08:39:41 浏览: 155
好的,下面是使用SegNet模型进行图像语义分割的全过程代码,包括模型的训练和预测过程,使用PyTorch框架实现。
首先,我们需要导入必要的库和模块:
```python
import torch
import torch.nn as nn
import torch.optim as optim
from torch.utils.data import Dataset, DataLoader
from torchvision import transforms, datasets
from PIL import Image
import numpy as np
import matplotlib.pyplot as plt
```
接下来,我们定义SegNet模型的结构:
```python
class SegNet(nn.Module):
def __init__(self, in_channels=3, out_channels=1):
super(SegNet, self).__init__()
self.encoder1 = nn.Sequential(
nn.Conv2d(in_channels, 64, kernel_size=3, stride=1, padding=1),
nn.BatchNorm2d(64),
nn.ReLU(inplace=True),
nn.Conv2d(64, 64, kernel_size=3, stride=1, padding=1),
nn.BatchNorm2d(64),
nn.ReLU(inplace=True),
nn.MaxPool2d(kernel_size=2, stride=2, return_indices=True)
)
self.encoder2 = nn.Sequential(
nn.Conv2d(64, 128, kernel_size=3, stride=1, padding=1),
nn.BatchNorm2d(128),
nn.ReLU(inplace=True),
nn.Conv2d(128, 128, kernel_size=3, stride=1, padding=1),
nn.BatchNorm2d(128),
nn.ReLU(inplace=True),
nn.MaxPool2d(kernel_size=2, stride=2, return_indices=True)
)
self.encoder3 = nn.Sequential(
nn.Conv2d(128, 256, kernel_size=3, stride=1, padding=1),
nn.BatchNorm2d(256),
nn.ReLU(inplace=True),
nn.Conv2d(256, 256, kernel_size=3, stride=1, padding=1),
nn.BatchNorm2d(256),
nn.ReLU(inplace=True),
nn.Conv2d(256, 256, kernel_size=3, stride=1, padding=1),
nn.BatchNorm2d(256),
nn.ReLU(inplace=True),
nn.MaxPool2d(kernel_size=2, stride=2, return_indices=True)
)
self.encoder4 = nn.Sequential(
nn.Conv2d(256, 512, kernel_size=3, stride=1, padding=1),
nn.BatchNorm2d(512),
nn.ReLU(inplace=True),
nn.Conv2d(512, 512, kernel_size=3, stride=1, padding=1),
nn.BatchNorm2d(512),
nn.ReLU(inplace=True),
nn.Conv2d(512, 512, kernel_size=3, stride=1, padding=1),
nn.BatchNorm2d(512),
nn.ReLU(inplace=True),
nn.MaxPool2d(kernel_size=2, stride=2, return_indices=True)
)
self.encoder5 = nn.Sequential(
nn.Conv2d(512, 512, kernel_size=3, stride=1, padding=1),
nn.BatchNorm2d(512),
nn.ReLU(inplace=True),
nn.Conv2d(512, 512, kernel_size=3, stride=1, padding=1),
nn.BatchNorm2d(512),
nn.ReLU(inplace=True),
nn.Conv2d(512, 512, kernel_size=3, stride=1, padding=1),
nn.BatchNorm2d(512),
nn.ReLU(inplace=True),
nn.MaxPool2d(kernel_size=2, stride=2, return_indices=True)
)
self.decoder5 = nn.Sequential(
nn.MaxUnpool2d(kernel_size=2, stride=2),
nn.Conv2d(512, 512, kernel_size=3, stride=1, padding=1),
nn.BatchNorm2d(512),
nn.ReLU(inplace=True),
nn.Conv2d(512, 512, kernel_size=3, stride=1, padding=1),
nn.BatchNorm2d(512),
nn.ReLU(inplace=True),
nn.Conv2d(512, 512, kernel_size=3, stride=1, padding=1),
nn.BatchNorm2d(512),
nn.ReLU(inplace=True)
)
self.decoder4 = nn.Sequential(
nn.MaxUnpool2d(kernel_size=2, stride=2),
nn.Conv2d(512, 512, kernel_size=3, stride=1, padding=1),
nn.BatchNorm2d(512),
nn.ReLU(inplace=True),
nn.Conv2d(512, 512, kernel_size=3, stride=1, padding=1),
nn.BatchNorm2d(512),
nn.ReLU(inplace=True),
nn.Conv2d(512, 256, kernel_size=3, stride=1, padding=1),
nn.BatchNorm2d(256),
nn.ReLU(inplace=True)
)
self.decoder3 = nn.Sequential(
nn.MaxUnpool2d(kernel_size=2, stride=2),
nn.Conv2d(256, 256, kernel_size=3, stride=1, padding=1),
nn.BatchNorm2d(256),
nn.ReLU(inplace=True),
nn.Conv2d(256, 256, kernel_size=3, stride=1, padding=1),
nn.BatchNorm2d(256),
nn.ReLU(inplace=True),
nn.Conv2d(256, 128, kernel_size=3, stride=1, padding=1),
nn.BatchNorm2d(128),
nn.ReLU(inplace=True)
)
self.decoder2 = nn.Sequential(
nn.MaxUnpool2d(kernel_size=2, stride=2),
nn.Conv2d(128, 128, kernel_size=3, stride=1, padding=1),
nn.BatchNorm2d(128),
nn.ReLU(inplace=True),
nn.Conv2d(128, 64, kernel_size=3, stride=1, padding=1),
nn.BatchNorm2d(64),
nn.ReLU(inplace=True)
)
self.decoder1 = nn.Sequential(
nn.MaxUnpool2d(kernel_size=2, stride=2),
nn.Conv2d(64, 64, kernel_size=3, stride=1, padding=1),
nn.BatchNorm2d(64),
nn.ReLU(inplace=True),
nn.Conv2d(64, out_channels, kernel_size=3, stride=1, padding=1),
nn.BatchNorm2d(out_channels),
nn.Sigmoid()
)
def forward(self, x):
size_1 = x.size()
x, idx_1 = self.encoder1(x)
size_2 = x.size()
x, idx_2 = self.encoder2(x)
size_3 = x.size()
x, idx_3 = self.encoder3(x)
size_4 = x.size()
x, idx_4 = self.encoder4(x)
size_5 = x.size()
x, idx_5 = self.encoder5(x)
x = self.decoder5(x, idx_5, size_5)
x = self.decoder4(x, idx_4, size_4)
x = self.decoder3(x, idx_3, size_3)
x = self.decoder2(x, idx_2, size_2)
x = self.decoder1(x, idx_1, size_1)
return x
```
接下来,我们需要定义数据集,包括训练集和测试集:
```python
class SegmentationDataset(Dataset):
def __init__(self, root_dir, transform=None):
self.images = []
self.masks = []
self.transform = transform
for filename in os.listdir(root_dir):
if filename.endswith(".jpg"):
image_file = os.path.join(root_dir, filename)
mask_file = os.path.join(root_dir, filename.split(".")[0] + "_mask.png")
self.images.append(image_file)
self.masks.append(mask_file)
def __len__(self):
return len(self.images)
def __getitem__(self, idx):
image = Image.open(self.images[idx])
mask = Image.open(self.masks[idx]).convert("L")
if self.transform:
image = self.transform(image)
mask = self.transform(mask)
return image, mask
```
接下来,我们需要对数据进行预处理,包括数据增强、归一化等:
```python
transform = transforms.Compose([
transforms.Resize((256, 256)),
transforms.RandomHorizontalFlip(),
transforms.RandomRotation(10),
transforms.ToTensor(),
transforms.Normalize(mean=[0.485, 0.456, 0.406], std=[0.229, 0.224, 0.225])
])
train_dataset = SegmentationDataset("train", transform=transform)
test_dataset = SegmentationDataset("test", transform=transform)
train_dataloader = DataLoader(train_dataset, batch_size=4, shuffle=True)
test_dataloader = DataLoader(test_dataset, batch_size=4, shuffle=False)
```
然后,我们需要定义损失函数和优化器:
```python
criterion = nn.BCELoss()
optimizer = optim.Adam(model.parameters(), lr=0.001)
```
接下来,我们可以开始训练模型了:
```python
device = torch.device("cuda" if torch.cuda.is_available() else "cpu")
model = SegNet().to(device)
for epoch in range(10):
for i, (images, masks) in enumerate(train_dataloader):
images = images.to(device)
masks = masks.to(device)
optimizer.zero_grad()
outputs = model(images)
loss = criterion(outputs, masks)
loss.backward()
optimizer.step()
if i % 10 == 0:
print("Epoch [{}/{}], Step [{}/{}], Loss: {:.4f}".format(epoch+1, 10, i+1, len(train_dataloader), loss.item()))
```
训练完成后,我们可以使用训练好的模型对新图像进行预测:
```python
def predict_image(model, image_path):
image = Image.open(image_path)
image_tensor = transform(image).unsqueeze(0).to(device)
output = model(image_tensor)
mask = output.squeeze().cpu().detach().numpy()
mask = np.where(mask > 0.5, 255, 0).astype(np.uint8)
mask_image = Image.fromarray(mask)
return mask_image
image_path = "test/image.jpg"
mask_image = predict_image(model, image_path)
mask_image.save("test/mask.png")
```
这样,我们就完成了使用SegNet模型进行图像语义分割的全过程代码。
阅读全文
相关推荐
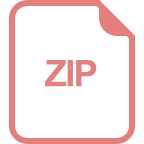
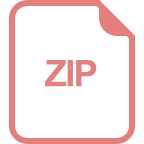
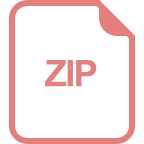

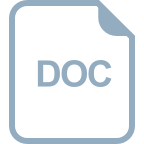
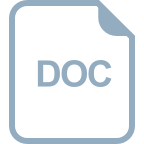
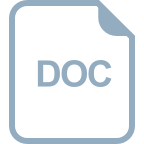
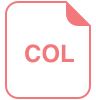
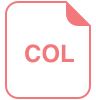
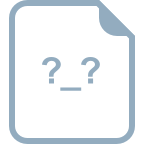
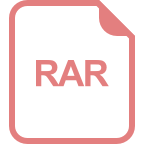
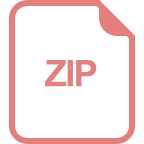
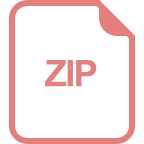
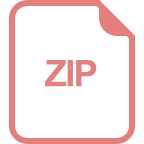
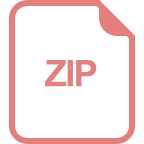
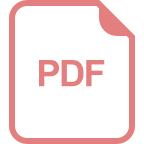
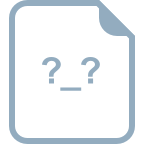
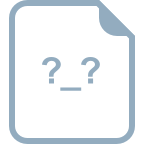
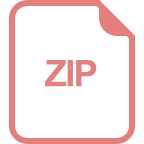