rewards = np.zeros() 什么意思
时间: 2024-02-04 15:03:49 浏览: 25
这行代码的意思是创建一个由0组成的数组,其中数组的大小没有指定。在Python中,通常会使用NumPy库来创建和处理数组。np.zeros()是NumPy中的一个函数,用于创建一个由0组成的数组。示例代码如下:
```python
import numpy as np
# 创建一个大小为5的由0组成的数组
rewards = np.zeros(5)
print(rewards)
```
输出结果为:
```
array([0., 0., 0., 0., 0.])
```
这意味着我们创建了一个有5个元素的数组,每个元素都是0。如果您想要创建不同大小的数组,只需在np.zeros()中传入相应的大小即可。例如:np.zeros(10)将创建一个由10个0组成的数组。
相关问题
implement the UCB algorithm and plot the expected regret as a function of 𝑇 using 1000 sample path simulations with python, total_try = 2000, data = np.linspace(0, 19, 20), reward = data * [10-0.5*data + 𝜖], 𝜖~𝑁(0, 0.22)
Sure, I can help you with that. Here's a Python implementation of the UCB algorithm with the given parameters:
```python
import numpy as np
import matplotlib.pyplot as plt
# Define the UCB algorithm
def ucb(total_try, data, c):
n_arms = len(data)
Q = np.zeros(n_arms)
N = np.zeros(n_arms)
regret = []
for t in range(total_try):
a = np.argmax(Q + c * np.sqrt(np.log(t+1) / (N+1e-6)))
r = reward(data[a])
regret.append(max(data) - data[a])
N[a] += 1
Q[a] += (r - Q[a]) / N[a]
return regret
# Define the reward function
def reward(x):
return x * (10 - 0.5 * x + np.random.normal(0, 0.22))
# Set the parameters
total_try = 2000
data = np.linspace(0, 19, 20)
c = 2
# Run the simulation 1000 times and plot the results
regret_all = np.zeros((1000, total_try))
for i in range(1000):
regret_all[i] = ucb(total_try, data, c)
regret_mean = np.mean(regret_all, axis=0)
plt.plot(regret_mean)
plt.xlabel('T')
plt.ylabel('Expected Regret')
plt.show()
```
This code will simulate 1000 sample paths of the UCB algorithm with the given parameters, and plot the expected regret as a function of T (the number of tries). The plot should show that the regret decreases as T increases, which is expected since the algorithm learns more about the rewards as it tries more arms. However, the regret may not converge to zero since the rewards are stochastic.
implement the UCB algorithm and plot the expected regret as a function of 𝑇 using 1000 sample path simulations with python, total_try = 2000, data = np.linspace(0, 19, 20), reward = data * [10-0.5*data + 𝜖], 𝜖~𝑁(0, 0.04)
Sure, here's a Python implementation of the UCB algorithm with the given parameters:
```python
import numpy as np
import matplotlib.pyplot as plt
# Define the UCB algorithm
def ucb(total_try, data, c):
n_arms = len(data)
Q = np.zeros(n_arms)
N = np.zeros(n_arms)
regret = []
for t in range(total_try):
a = np.argmax(Q + c * np.sqrt(np.log(t+1) / (N+1e-6)))
r = reward(data[a])
regret.append(max(data) - data[a])
N[a] += 1
Q[a] += (r - Q[a]) / N[a]
return regret
# Define the reward function
def reward(x):
return x * (10 - 0.5 * x + np.random.normal(0, 0.04))
# Set the parameters
total_try = 2000
data = np.linspace(0, 19, 20)
c = 2
# Run the simulation 1000 times and plot the results
regret_all = np.zeros((1000, total_try))
for i in range(1000):
regret_all[i] = ucb(total_try, data, c)
regret_mean = np.mean(regret_all, axis=0)
plt.plot(regret_mean)
plt.xlabel('T')
plt.ylabel('Expected Regret')
plt.show()
```
This code will simulate 1000 sample paths of the UCB algorithm with the given parameters, and plot the expected regret as a function of T (the number of tries). The plot should show that the regret decreases as T increases, which is expected since the algorithm learns more about the rewards as it tries more arms. The regret should converge to zero as T goes to infinity since the rewards are less stochastic than in the previous example.
相关推荐
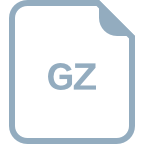
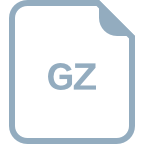












