详细解释这段代码 if self.args.shared_params: # print (f"This is the shape of last_hids: {last_hid.size()}") obs = obs.contiguous().view(batch_size*self.n_, -1) # shape = (b*n, n+o/o) agent_policy = self.policy_dicts[0] means, log_stds, hiddens = agent_policy(obs, last_hid) # hiddens = th.stack(hiddens, dim=1) means = means.contiguous().view(batch_size, self.n_, -1) hiddens = hiddens.contiguous().view(batch_size, self.n_, -1) if self.args.gaussian_policy: log_stds = log_stds.contiguous().view(batch_size, self.n_, -1) else: stds = th.ones_like(means).to(self.device) * self.args.fixed_policy_std log_stds = th.log(stds)
时间: 2024-04-23 15:21:46 浏览: 107
这段代码是一个if语句块,判断了一个名为self.args.shared_params的变量是否为True。
如果为True,执行下面的代码块,首先将obs变量进行形状变换,使其形状变为(batch_size * self.n_, -1)。其中,batch_size表示批次大小,self.n_表示agent的数量,-1表示自动推断。这里的obs是神经网络中的输入,包含了当前的状态信息。
接着,从self.policy_dicts字典中获取第一个策略模型agent_policy,并将obs和last_hid作为其输入,得到该模型的输出means、log_stds和hiddens。
接下来,对means、hiddens和log_stds进行形状变换,使其恢复为(batch_size, self.n_, -1)的形式。如果self.args.gaussian_policy为True,则log_stds仍然表示标准差的对数值;否则,将means设置为一个全1的张量,并将其与self.args.fixed_policy_std相乘得到标准差,再计算其对数值。最终得到的means、hiddens和log_stds将作为神经网络的输出,用于指导接下来的动作选择。
阅读全文
相关推荐
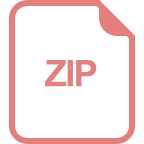
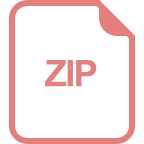
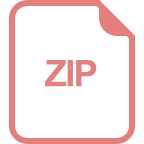
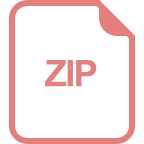
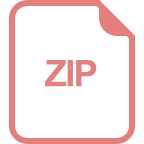
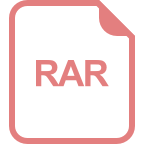
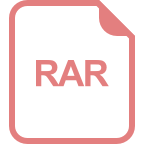
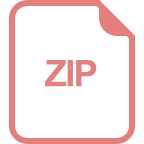
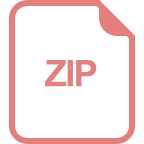
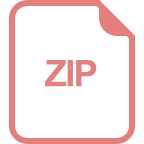
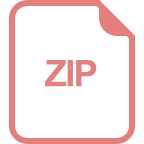
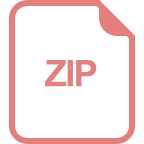
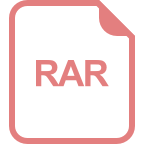
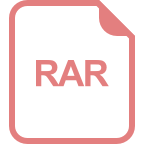
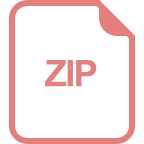
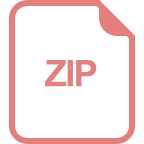
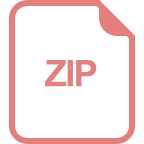