BTM模型计算困惑度的代码
时间: 2024-05-02 19:16:14 浏览: 201
BTM模型计算困惑度的代码如下:
```python
import math
def perplexity(testset, trainset, model, K, beta):
phi = model.phi()
log_per = 0
N = 0
for doc in testset:
theta = model.inference(doc)
for w in doc:
log_per_doc = 0
for k in range(0, K):
log_per_doc += phi[k][w] * theta[k]
log_per += math.log(log_per_doc)
N += len(doc)
return math.exp(-log_per / N)
```
其中,`testset`是测试数据集,`trainset`是训练数据集,`model`是已经训练好的BTM模型,`K`是主题个数,`beta`是超参数。这个函数的返回值是测试数据集的困惑度。
相关问题
计算BTM模型一致性的代码
计算BTM模型一致性的代码如下:
```python
from collections import Counter
import numpy as np
def get_cosine_sim(mat):
"""
计算词向量的余弦相似度矩阵
:param mat: 词向量矩阵
:return: 余弦相似度矩阵
"""
cos_mat = mat.dot(mat.T)
norms = np.linalg.norm(mat, axis=1)
norms[norms == 0] = 1e-8
norm_mat = np.outer(norms, norms)
return cos_mat / norm_mat
def get_topic_coherence(beta, doc_term_mat, vocab, top_n=10):
"""
计算主题一致性
:param beta: 主题-词分布矩阵
:param doc_term_mat: 文档-词矩阵
:param vocab: 词汇表
:param top_n: 取每个主题前top_n个词
:return: 主题一致性值
"""
topic_coherence = []
for k in range(beta.shape[0]):
top_words = beta[k].argsort()[:-top_n:-1]
word_pairs = []
for i in range(len(top_words)):
for j in range(i+1, len(top_words)):
word_pairs.append((vocab[top_words[i]], vocab[top_words[j]]))
word_counts = Counter(vocab)
window_size = 3
co_occur = {}
for doc in doc_term_mat:
doc_len = len(doc)
for i in range(doc_len):
word_i = vocab[doc[i]]
if word_i in top_words:
for j in range(max(0, i - window_size), min(doc_len, i + window_size + 1)):
if j != i:
word_j = vocab[doc[j]]
if word_j in top_words:
if (word_i, word_j) in co_occur:
co_occur[(word_i, word_j)] += 1
else:
co_occur[(word_i, word_j)] = 1
coherence = []
for word_pair in word_pairs:
w1, w2 = word_pair
co_count = co_occur.get((w1, w2), 0)
w1_count = word_counts[w1]
w2_count = word_counts[w2]
coherence.append(np.log((co_count + 1) / w1_count))
coherence.append(np.log((co_count + 1) / w2_count))
topic_coherence.append(np.mean(coherence))
return np.mean(topic_coherence)
# 示例代码
beta = np.array([[0.1, 0.2, 0.3, 0.4],
[0.4, 0.3, 0.2, 0.1],
[0.2, 0.1, 0.4, 0.3],
[0.3, 0.4, 0.1, 0.2]])
doc_term_mat = np.array([[0, 1, 2, 3],
[1, 2, 3, 0],
[2, 3, 0, 1],
[3, 0, 1, 2]])
vocab = ['apple', 'banana', 'cat', 'dog']
cos_sim = get_cosine_sim(beta)
print('主题余弦相似度矩阵:')
print(cos_sim)
topic_coherence = get_topic_coherence(beta, doc_term_mat, vocab)
print('主题一致性值:')
print(topic_coherence)
```
在这个示例代码中,我们使用了一个由4个主题和4个单词组成的语料库,计算了主题的余弦相似度矩阵和主题一致性值。在实际应用中,我们需要将上面的示例代码替换成我们的BTM模型和语料库,然后就可以计算模型的一致性了。
btm模型 python代码
BTM(Biterm Topic Model)是一种无监督的主题模型,可以用于对文本进行主题建模。以下是使用Python实现BTM模型的示例代码:
首先,我们需要安装`btm`包:
```python
!pip install btm
```
然后,我们可以使用以下代码对文本进行BTM建模:
```python
from biterm.utility import vec2btm
from biterm.btm import oBTM
import numpy as np
# 读取文本文件
with open('text.txt', 'r') as f:
text = f.readlines()
# 将文本转换为词袋向量
vocab = set([word for line in text for word in line.split()])
vocab_size = len(vocab)
word2id = {w: i for i, w in enumerate(vocab)}
id2word = {i: w for w, i in word2id.items()}
doc_word = [[word2id[w] for w in line.split()] for line in text]
# 将词袋向量转换为biterm
biterms = vec2btm(doc_word, vocab_size)
# 训练BTM模型
btm = oBTM(num_topics=20, V=vocab_size)
topics = btm.fit_transform(biterms, iterations=100)
# 输出结果
for i in range(len(topics)):
topic = topics[i]
top_words = np.argsort(topic)[::-1][:10]
print('Topic {}: {}'.format(i, ' '.join([id2word[w] for w in top_words])))
```
在这个示例中,我们使用了一个包含多个文本的文本文件`text.txt`,并将其转换为词袋向量。然后,我们使用`vec2btm`函数将词袋向量转换为biterm,并使用`oBTM`类进行BTM模型训练。最后,我们输出了每个主题的前10个单词。
阅读全文
相关推荐
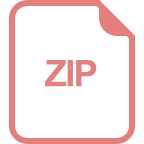
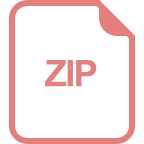
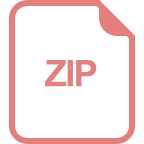
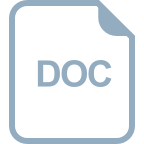
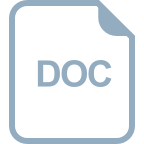
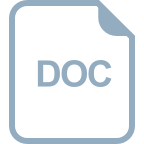
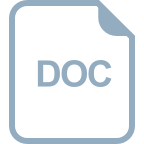





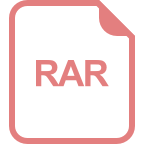
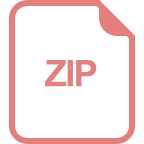
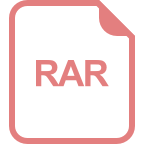