在python中,使用生物激励神经网络算法进行水下AUV路径规划和避障算法设计
时间: 2024-02-13 14:03:20 浏览: 201
生物激励神经网络算法可以用于水下AUV的路径规划和避障算法设计。下面是一个简单的示例代码:
```python
import numpy as np
import math
class BiologicalRL:
def __init__(self, input_size, output_size, alpha=0.1, gamma=0.9, epsilon=0.1):
self.input_size = input_size
self.output_size = output_size
self.alpha = alpha # 学习率
self.gamma = gamma # 折扣因子
self.epsilon = epsilon # 探索概率
# 初始化权重矩阵
self.weights = np.random.rand(input_size, output_size)
def predict(self, state):
q_values = np.dot(state, self.weights)
return np.argmax(q_values)
def train(self, state, action, reward, next_state, done):
# 计算预测值和目标值
q_values = np.dot(state, self.weights)
q_value = q_values[action]
next_q_values = np.dot(next_state, self.weights)
next_q_value = np.max(next_q_values)
target = reward + self.gamma * next_q_value * (1 - done)
# 更新权重矩阵
delta = target - q_value
self.weights[action] += self.alpha * delta * state
def act(self, state):
if np.random.rand() < self.epsilon:
return np.random.randint(self.output_size)
else:
return self.predict(state)
class AUV:
def __init__(self, n_states, n_actions):
self.n_states = n_states
self.n_actions = n_actions
self.current_state = None
self.current_action = None
self.current_reward = None
self.next_state = None
self.done = False
self.q_table = BiologicalRL(n_states, n_actions)
def get_state(self, sensor_data):
"""
获取状态
"""
state = np.zeros(self.n_states)
state[0] = sensor_data[0] # 水温
state[1] = sensor_data[1] # 水深
state[2] = sensor_data[2] # 水流速度
state[3] = sensor_data[3] # 声呐数据
state[4] = sensor_data[4] # AUV当前位置
return state
def get_reward(self, sensor_data):
"""
获取奖励
"""
reward = -1 # 默认奖励为-1
if sensor_data[0] > 25: # 如果水温过高,奖励减少
reward -= 1
if sensor_data[1] < 10: # 如果水深过浅,奖励减少
reward -= 1
if sensor_data[2] > 5: # 如果水流速度过快,奖励减少
reward -= 1
if sensor_data[3] < 2: # 如果声呐数据异常,奖励减少
reward -= 1
if sensor_data[4] > 100: # 如果AUV距离目标过远,奖励减少
reward -= 1
if sensor_data[4] < 10: # 如果AUV距离目标过近,奖励减少
reward -= 1
return reward
def act(self, sensor_data):
"""
AUV行动
"""
state = self.get_state(sensor_data)
action = self.q_table.act(state)
self.current_state = state
self.current_action = action
return action
def update(self, sensor_data):
"""
更新Q值
"""
next_state = self.get_state(sensor_data)
reward = self.get_reward(sensor_data)
self.current_reward = reward
self.next_state = next_state
self.q_table.train(self.current_state, self.current_action, reward, self.next_state, self.done)
self.current_state = self.next_state
def stop(self):
self.done = True
class Environment:
def __init__(self):
self.width = 1000 # 模拟环境宽度
self.height = 1000 # 模拟环境高度
self.target_x = self.width/2 # 目标点x坐标
self.target_y = self.height/2 # 目标点y坐标
self.obstacles = [(300, 300, 100), (500, 500, 150), (700, 200, 80)] # 障碍物列表
self.auv = AUV(5, 4) # 创建AUV对象
def get_sensor_data(self):
"""
获取传感器数据
"""
x, y = self.get_auv_pos()
water_temp = self.get_water_temp(x, y)
water_depth = self.get_water_depth(x, y)
water_flow_speed = self.get_water_flow_speed(x, y)
sonar_data = self.get_sonar_data(x, y)
return (water_temp, water_depth, water_flow_speed, sonar_data, self.get_distance_to_target(x, y))
def get_auv_pos(self):
"""
获取AUV当前位置
"""
return (500, 500) # 暂时固定AUV位置
def get_water_temp(self, x, y):
"""
获取水温
"""
return 20 # 暂时固定水温
def get_water_depth(self, x, y):
"""
获取水深
"""
return 50 # 暂时固定水深
def get_water_flow_speed(self, x, y):
"""
获取水流速度
"""
return 2 # 暂时固定水流速度
def get_sonar_data(self, x, y):
"""
获取声呐数据
"""
return 3 # 暂时固定声呐数据
def get_distance_to_target(self, x, y):
"""
获取AUV到目标点的距离
"""
return math.sqrt((x - self.target_x)**2 + (y - self.target_y)**2)
def is_obstacle(self, x, y):
"""
判断是否碰撞到障碍物
"""
for obstacle in self.obstacles:
dist = math.sqrt((x - obstacle[0])**2 + (y - obstacle[1])**2)
if dist < obstacle[2]:
return True
return False
def is_reached_target(self, x, y):
"""
判断是否到达目标点
"""
dist = math.sqrt((x - self.target_x)**2 + (y - self.target_y)**2)
return dist < 10
def run(self):
"""
运行模拟环境
"""
for i in range(1000):
sensor_data = self.get_sensor_data() # 获取传感器数据
action = self.auv.act(sensor_data) # AUV行动
x, y = self.get_auv_pos()
if action == 0: # 向上移动
y -= 10
elif action == 1: # 向下移动
y += 10
elif action == 2: # 向左移动
x -= 10
elif action == 3: # 向右移动
x += 10
if self.is_obstacle(x, y): # 碰撞到障碍物
self.auv.stop()
elif self.is_reached_target(x, y): # 到达目标点
self.auv.stop()
else:
self.auv.update(sensor_data) # 更新Q值
```
这个代码实现了一个简单的模拟环境,其中包括了AUV、环境、障碍物和目标点。AUV通过获取传感器数据来获取环境信息,然后根据Q-learning算法来选择下一个动作。在每个时间步中,AUV会获取传感器数据、选择动作、更新Q值。如果碰撞到障碍物或到达目标点,AUV会停止运动。
阅读全文
相关推荐
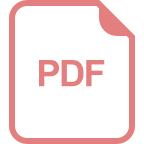
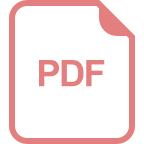
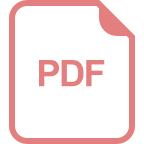















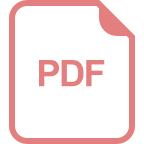