用c++写一个聚类算法
时间: 2023-03-15 11:56:38 浏览: 80
我可以给你一个用C语言写聚类算法的例子: 1.初始化数据:选择一组数据,将其分割为k个分组,每个分组的中心点作为聚类中心;2.使用距离公式计算每个数据点到每个聚类中心的距离;3.将每个数据点分配给距离它最近的聚类中心;4.重新计算每个聚类中心的位置;5.重复步骤2-4,直至聚类中心的位置不再发生变化;6.输出聚类结果。
相关问题
用c++写一个DBSCAN聚类算法
好的,以下是一个简单的C++实现DBSCAN聚类算法的示例代码:
```c++
#include <iostream>
#include <vector>
#include <cmath>
using namespace std;
struct Point {
double x, y;
bool visited;
int cluster;
};
double distance(Point a, Point b) {
return sqrt(pow(a.x - b.x, 2) + pow(a.y - b.y, 2));
}
vector<Point> regionQuery(vector<Point>& points, int index, double eps) {
vector<Point> neighbors;
for (int i = 0; i < points.size(); i++) {
if (distance(points[i], points[index]) <= eps) {
neighbors.push_back(points[i]);
}
}
return neighbors;
}
void expandCluster(vector<Point>& points, int index, vector<Point>& neighbors, int cluster, double eps, int minPts) {
points[index].cluster = cluster;
for (int i = 0; i < neighbors.size(); i++) {
int neighborIndex = &neighbors[i] - &points[0];
if (!neighbors[i].visited) {
neighbors[i].visited = true;
vector<Point> newNeighbors = regionQuery(points, neighborIndex, eps);
if (newNeighbors.size() >= minPts) {
neighbors.insert(neighbors.end(), newNeighbors.begin(), newNeighbors.end());
}
}
if (points[neighborIndex].cluster == -1) {
points[neighborIndex].cluster = cluster;
}
}
}
void DBSCAN(vector<Point>& points, double eps, int minPts) {
int cluster = 0;
for (int i = 0; i < points.size(); i++) {
if (!points[i].visited) {
points[i].visited = true;
vector<Point> neighbors = regionQuery(points, i, eps);
if (neighbors.size() < minPts) {
points[i].cluster = -1;
} else {
expandCluster(points, i, neighbors, cluster, eps, minPts);
cluster++;
}
}
}
}
int main() {
vector<Point> points = {{1, 1}, {1, 2}, {2, 1}, {10, 10}, {10, 12}, {12, 10}, {50, 50}, {50, 52}, {52, 50}};
double eps = 3;
int minPts = 3;
DBSCAN(points, eps, minPts);
for (int i = 0; i < points.size(); i++) {
cout << "(" << points[i].x << ", " << points[i].y << ") - Cluster " << points[i].cluster << endl;
}
return 0;
}
```
这个示例代码使用了一个Point结构体来存储点的坐标、是否被访问过、聚类编号等信息。`distance()`函数用来计算两个点之间的距离,`regionQuery()`函数用来查询某个点的邻居点,`expandCluster()`函数用来将某个点及其邻居点分配到同一个聚类中。最后的`DBSCAN()`函数是整个算法的主体部分,通过遍历所有点来实现聚类。在这个示例代码中,我们使用了一个简单的点集来测试算法的正确性,并输出了每个点所属的聚类编号。
写一个基于opencv c++的聚类算法
以下是一个基于OpenCV C++的聚类算法示例,使用K-means算法实现:
```
#include <opencv2/core/core.hpp>
#include <opencv2/highgui/highgui.hpp>
#include <opencv2/imgproc/imgproc.hpp>
#include <iostream>
#include <vector>
using namespace cv;
using namespace std;
int main()
{
Mat image = imread("example.jpg", IMREAD_COLOR);
if (image.empty())
{
cout << "Could not open or find the image" << endl;
return -1;
}
Mat reshaped_image = image.reshape(1, image.rows * image.cols);
reshaped_image.convertTo(reshaped_image, CV_32F);
int K = 6; // number of clusters
TermCriteria criteria(TermCriteria::EPS + TermCriteria::COUNT, 10, 1.0);
int attempts = 5;
Mat labels, centers;
kmeans(reshaped_image, K, labels, criteria, attempts, KMEANS_RANDOM_CENTERS, centers);
vector<Mat> clusters(K);
for (int i = 0; i < K; i++)
{
clusters[i] = Mat::zeros(image.rows, image.cols, CV_8UC3);
}
MatIterator_<Vec3b> it, end;
int i = 0;
for (it = image.begin<Vec3b>(), end = image.end<Vec3b>(); it != end; ++it, ++i)
{
int cluster_idx = labels.at<int>(i);
clusters[cluster_idx].at<Vec3b>(i) = *it;
}
for (int i = 0; i < K; i++)
{
imshow("Cluster " + to_string(i), clusters[i]);
}
waitKey(0);
return 0;
}
```
该示例读取一张图像,将其重塑为行向量,然后使用K-means算法将其聚类为给定数量的簇。最终,每个簇都被分配到一个颜色,并显示在单独的窗口中。
相关推荐
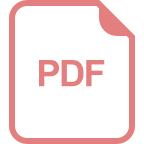
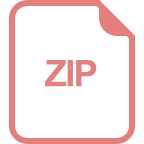












