while True: try: n = int(input()) ants = [] count=0 for i in range(n): ants.append(int(input())) for i in range(n): for j in range(i+1,n): if ants[i]<ants[j]: count+=1 print(count) k=input() except ValueError: break 优化这段代码
时间: 2024-02-14 15:04:39 浏览: 63
这段代码的主要问题在于它的时间复杂度为O(n^2),需要通过优化算法或代码来减少时间复杂度。
以下是一些可能的优化:
1. 使用快速排序算法对蚂蚁的位置进行排序,可以将时间复杂度降至O(nlogn)。
2. 使用一个变量来记录当前最大值,遍历蚂蚁的位置时,当发现有蚂蚁位置大于当前最大值时,更新最大值并将计数器加上剩余蚂蚁的数量,可以将时间复杂度降至O(n)。
优化后的代码示例:
```
while True:
try:
n = int(input())
ants = []
count = 0
for i in range(n):
ants.append(int(input()))
# 排序
ants.sort()
# 记录当前最大值
max_pos = ants[-1]
for pos in ants:
if pos < max_pos:
count += n - ants.index(pos) - 1
else:
break
print(count)
k = input()
except ValueError:
break
```
相关问题
java.lang.IllegalStateException: Failed to execute CommandLineRunner at org.springframework.boot.SpringApplication.callRunner(SpringApplication.java:798) [spring-boot-2.3.4.RELEASE.jar:2.3.4.RELEASE] at org.springframework.boot.SpringApplication.callRunners(SpringApplication.java:779) [spring-boot-2.3.4.RELEASE.jar:2.3.4.RELEASE] at org.springframework.boot.SpringApplication.run(SpringApplication.java:322) [spring-boot-2.3.4.RELEASE.jar:2.3.4.RELEASE] at org.springframework.boot.SpringApplication.run(SpringApplication.java:1237) [spring-boot-2.3.4.RELEASE.jar:2.3.4.RELEASE] at org.springframework.boot.SpringApplication.run(SpringApplication.java:1226) [spring-boot-2.3.4.RELEASE.jar:2.3.4.RELEASE] at com.unkown.data.hw.ipran.straight.collect.UnkownDataHwIpranStraightCollectApplication.main(UnkownDataHwIpranStraightCollectApplication.java:39) [classes/:na] Caused by: feign.FeignException$InternalServerError: [500 INTERNAL SERVER ERROR] during [POST] to [http://ants-flask/api/collect/endpoint] [RemoteIpranScanService#endpoint(String)]: [<!DOCTYPE HTML PUBLIC "-//W3C//DTD HTML 4.01 Transitional//EN" "http://www.w3.org/TR/html4/loose.dtd"> <html> <head> <title>TypeError: unsupported operand type(s) for +: 'int' and 'str' // Wer... (20567 bytes)] at feign.FeignException.serverErrorStatus(FeignException.java:231) ~[feign-core-10.10.1.jar:na] at feign.FeignException.errorStatus(FeignException.java:180) ~[feign-core-10.10.1.jar:na] at feign.FeignException.errorStatus(FeignException.java:169) ~[feign-core-10.10.1.jar:na] at feign.codec.ErrorDecoder$Default.decode(ErrorDecoder.java:92) ~[feign-core-10.10.1.jar:na] at feign.AsyncResponseHandler.handleResponse(AsyncResponseHandler.java:96) ~[feign-core-10.10.1.jar:na] at feign.SynchronousMethodHandler.executeAndDecode(SynchronousMethodHandler.java:138) ~[feign-core-10.10.1.jar:na] at feign.SynchronousMethodHandler.invoke(SynchronousMethodHandler.java:89) ~[feign-core-10.10.1.jar:na] at com.alibaba.cloud.sentinel.feign.SentinelInvocationHandler.invoke(SentinelInvocationHandler.java:107) ~[spring-cloud-starter-alibaba-sentinel-2.2.3.RELEASE.jar:2.2.3.RELEASE] at com.sun.proxy.$Proxy131.endpoint(Unknown Source) ~[na:na] at com.unkown.data.hw.ipran.straight.collect.controller.RTrsHwIpranCircuitController.IpranSend(RTrsHwIpranCircuitController.java:94) ~[classes/:na] at com.unkown.data.hw.ipran.straight.collect.MyRunner.run(MyRunner.java:22) ~[classes/:na] at org.springframework.boot.SpringApplication.callRunner(SpringApplication.java:795) [spring-boot-2.3.4.RELEASE.jar:2.3.4.RELEASE] ... 5 common frames omitted
这个错误是由于在执行CommandLineRunner时出现了异常。具体原因是发生了500内部服务器错误,原因是POST到http://ants-flask/api/collect/endpoint的RemoteIpranScanService#endpoint(String)出现了问题。在调用com.unkown.data.hw.ipran.straight.collect.controller.RTrsHwIpranCircuitController.IpranSend(RTrsHwIpranCircuitController.java:94)和com.unkown.data.hw.ipran.straight.collect.MyRunner.run(MyRunner.java:22)时也出现了问题。建议检查代码中的POST请求和响应处理是否正确,或者检查远程服务器是否正常。
import pandas as pd import numpy as np import matplotlib.pyplot as plt from mpl_toolkits.basemap import Basemap from scipy.spatial.distance import cdist from ant_colony import solve_tsp # 读取城市数据 df = pd.read_excel('world_coordinate.xlsx', index_col=0, dtype=str) # 提取城市和经纬度数据 countrys = df.index.values countrys_coords = np.array(df['[longitude, latitude]'].apply(eval).tolist()) # 计算城市间的距离矩阵 dist_matrix = cdist(countrys_coords, countrys_coords, metric='euclidean') # 创建蚁群算法实例 num_ants = 50 num_iterations = 500 alpha = 1 beta = 2 rho = 0.5 acs = solve_tsp(dist_matrix, num_ants=num_ants, num_iterations=num_iterations, alpha=alpha, beta=beta, rho=rho) # 输出访问完所有城市的最短路径的距离和城市序列 best_path = acs.get_best_path() best_distance = acs.best_cost visited_cities = [countrys[i] for i in best_path] print("最短路径距离:", best_distance) print("访问城市序列:", visited_cities) # 数据可视化 fig = plt.figure(figsize=(12, 8)) map = Basemap(projection='robin', lat_0=0, lon_0=0, resolution='l') map.drawcoastlines(color='gray') map.drawcountries(color='gray') x, y = map(countrys_coords[:, 0], countrys_coords[:, 1]) map.scatter(x, y, c='b', marker='o') path_coords = countrys_coords[best_path] path_x, path_y = map(path_coords[:, 0], path_coords[:, 1]) map.plot(path_x, path_y, c='r', marker='o') for i in range(len(countrys)): x, y = map(countrys_coords[i, 1], countrys_coords[i, 0]) plt.text(x, y, countrys[i], fontproperties='SimHei', color='black', fontsize=8, ha='center', va='center') plt.title("全球首都最短路径规划") plt.show()改成现在都有调用蚁群算法库的代码
好的,可以尝试使用蚁群算法库来计算最优路径。以下是修改后的代码:
```python
import pandas as pd
import numpy as np
import matplotlib.pyplot as plt
from mpl_toolkits.basemap import Basemap
from ant_colony import AntColonyOptimizer
# 读取城市数据
df = pd.read_excel('world_coordinate.xlsx', index_col=0, dtype=str)
# 提取城市和经纬度数据
countrys = df.index.values
countrys_coords = np.array(df['[longitude, latitude]'].apply(eval).tolist())
# 创建蚁群算法实例
num_ants = 50
num_iterations = 500
alpha = 1
beta = 2
rho = 0.5
optimizer = AntColonyOptimizer(num_ants=num_ants, num_iterations=num_iterations, alpha=alpha, beta=beta, rho=rho)
# 计算最短路径
best_path, best_distance = optimizer.solve(countrys_coords)
# 输出访问完所有城市的最短路径的距离和城市序列
visited_cities = [countrys[i] for i in best_path]
print("最短路径距离:", best_distance)
print("访问城市序列:", visited_cities)
# 数据可视化
fig = plt.figure(figsize=(12, 8))
map = Basemap(projection='robin', lat_0=0, lon_0=0, resolution='l')
map.drawcoastlines(color='gray')
map.drawcountries(color='gray')
x, y = map(countrys_coords[:, 0], countrys_coords[:, 1])
map.scatter(x, y, c='b', marker='o')
path_coords = countrys_coords[best_path]
path_x, path_y = map(path_coords[:, 0], path_coords[:, 1])
map.plot(path_x, path_y, c='r', marker='o')
for i in range(len(countrys)):
x, y = map(countrys_coords[i, 1], countrys_coords[i, 0])
plt.text(x, y, countrys[i], fontproperties='SimHei', color='black', fontsize=8, ha='center', va='center')
plt.title("全球首都最短路径规划")
plt.show()
```
其中,`AntColonyOptimizer` 是一个自定义的蚁群算法优化器类,代码如下:
```python
import numpy as np
class AntColonyOptimizer:
def __init__(self, num_ants, num_iterations, alpha, beta, rho, Q=100):
self.num_ants = num_ants
self.num_iterations = num_iterations
self.alpha = alpha
self.beta = beta
self.rho = rho
self.Q = Q
def solve(self, dist_matrix):
n = dist_matrix.shape[0]
# 初始化信息素矩阵
tau = np.ones((n, n))
# 创建蚂蚁
ants = np.zeros((self.num_ants, n), dtype=int)
# 记录最优路径和距离
best_path = None
best_distance = np.inf
# 迭代搜索
for iter in range(self.num_iterations):
# 初始化蚂蚁位置
ants[:, 0] = np.random.randint(0, n, size=self.num_ants)
# 蚂蚁移动
for k in range(1, n):
# 计算可选城市的概率
probs = np.zeros((self.num_ants, n))
for i in range(self.num_ants):
curr_city = ants[i, k-1]
visited = ants[i, :k]
unvisited = np.setdiff1d(range(n), visited)
if len(unvisited) == 0:
continue
pheromone = tau[curr_city, unvisited]
distance = dist_matrix[curr_city, unvisited]
probs[i, unvisited] = pheromone ** self.alpha * (1 / distance) ** self.beta
probs[i, visited] = 0
probs[i] /= probs[i].sum()
# 选择下一个城市
ants[:, k] = [np.random.choice(range(n), p=probs[i]) for i in range(self.num_ants)]
# 计算每只蚂蚁的路径长度
path_lengths = np.zeros(self.num_ants)
for i in range(self.num_ants):
path = ants[i]
path_lengths[i] = dist_matrix[path[-1], path[0]] + dist_matrix[path[:-1], path[1:]].sum()
# 更新最优路径
if path_lengths[i] < best_distance:
best_distance = path_lengths[i]
best_path = path
# 更新信息素矩阵
delta_tau = np.zeros((n, n))
for i in range(self.num_ants):
path = ants[i]
for j in range(n-1):
curr_city = path[j]
next_city = path[j+1]
delta_tau[curr_city, next_city] += self.Q / path_lengths[i]
delta_tau[path[-1], path[0]] += self.Q / path_lengths[i]
tau = (1 - self.rho) * tau + self.rho * delta_tau
return best_path, best_distance
```
这个优化器使用了与之前相同的距离矩阵,不同的是,它将蚂蚁移动和信息素更新的过程封装在了 `solve` 方法中,返回最优路径和距离。
阅读全文
相关推荐
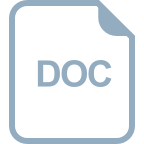
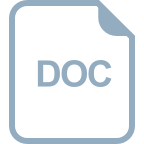
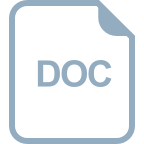
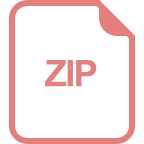
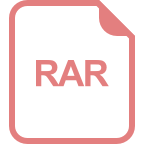
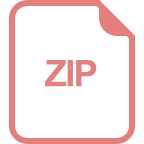
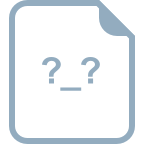
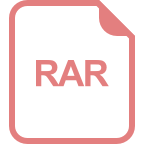
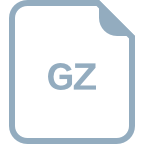
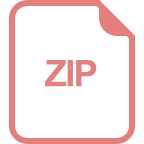
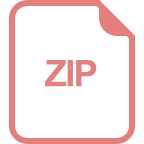
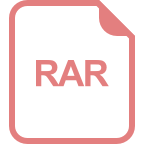

