def batch_gradientDescent(x, y, w, alpha, iters): temp = np.matrix(np.zeros(w.shape)) parameters = int(w.ravel().shape[1]) cost = np.zeros(iters) for i in range(iters): error = (x * w.T) - y for j in range(parameters): term = np.multiply(error, x[:, j]) temp[0, j] = w[0, j] - ((alpha / len(X)) * np.sum(term)) w = temp cost[i] = funcCost(x, y, w) return w, cost能给我解释一下这段代码吗
时间: 2024-04-26 13:23:13 浏览: 239
这段代码实现了一个批量梯度下降算法(batch gradient descent algorithm),用于线性回归模型的参数估计。其中:
- x:表示输入特征矩阵,它的每一行代表一个样本,每一列代表一个特征。
- y:表示样本的真实标签,它是一个向量。
- w:表示线性回归模型的参数向量,它是一个行向量。
- alpha:表示学习率(learning rate),它是一个标量,控制每次参数更新的步长。
- iters:表示迭代次数,也就是梯度下降算法要运行多少次。
在这个函数中,我们首先初始化一个临时的参数向量 temp,然后根据迭代次数 iters 进行循环。在每一次循环中,我们计算当前模型的预测值与真实值之间的误差,然后根据误差和输入特征矩阵 x 计算出每个参数的梯度。接着,我们使用梯度下降的公式更新每个参数,也就是将当前的参数值减去学习率乘以梯度。最后,我们将更新后的参数赋值给 temp,并记录下当前的代价函数值(即均方误差)。最终,我们返回更新后的参数向量 w 和每次迭代的代价函数值 cost。
相关问题
给出下面代码注释def batch_gradientDescent(X, y, w, alpha, iters): temp = np.matrix(np.zeros(w.shape)) parameters = int(w.ravel().shape[1]) cost = np.zeros(iters) for i in range(iters): error = (X * w.T) - y for j in range(parameters): term = np.multiply(error, X[:, j]) temp[0, j] = w[0, j] - ((alpha / len(X)) * np.sum(term)) w = temp cost[i] = computeCost(X, y, w) return w, cost
这是一段代码,用于实现批量梯度下降算法,对于给定的输入数据 X 和标签 y,通过不断更新参数 w,来最小化损失函数。其中 alpha 是学习率,iters 是迭代次数。函数返回最终的参数 w 和每次迭代的损失值 cost。
class TimeWindowSequence(Sequence): def __init__(self, x, y, batch_size, window_size): self.x = x self.y = y self.batch_size = batch_size self.window_size = window_size self.window_count = int(np.ceil(x.shape[0] / window_size)) def __len__(self): return int(np.ceil(self.x.shape[0] / self.batch_size)) def __getitem__(self, idx): batch_x = np.zeros((self.batch_size, self.window_size, self.x.shape[1])) batch_y = np.zeros((self.batch_size, self.y.shape[1])) for i in range(self.batch_size): j = idx * self.batch_size + i if j >= self.window_count: break window_x = self.x[j*self.window_size:(j+1)*self.window_size, :] window_y = self.y[j*self.window_size:(j+1)*self.window_size, :] batch_x[i, :window_x.shape[0], :] = window_x batch_y[i, :] = window_y[-1, :] return batch_x, batch_y出现
这段代码中似乎缺少了一个缩进,导致 `return` 语句在 `for` 循环内部。可以尝试将 `return` 语句缩进到 `for` 循环外部,如下所示:
```
class TimeWindowSequence(Sequence):
def __init__(self, x, y, batch_size, window_size):
self.x = x
self.y = y
self.batch_size = batch_size
self.window_size = window_size
self.window_count = int(np.ceil(x.shape[0] / window_size))
def __len__(self):
return int(np.ceil(self.x.shape[0] / self.batch_size))
def __getitem__(self, idx):
batch_x = np.zeros((self.batch_size, self.window_size, self.x.shape[1]))
batch_y = np.zeros((self.batch_size, self.y.shape[1]))
for i in range(self.batch_size):
j = idx * self.batch_size + i
if j >= self.window_count:
break
window_x = self.x[j*self.window_size:(j+1)*self.window_size, :]
window_y = self.y[j*self.window_size:(j+1)*self.window_size, :]
batch_x[i, :window_x.shape[0], :] = window_x
batch_y[i, :] = window_y[-1, :]
return batch_x, batch_y
```
这样应该就可以解决这个问题了。
阅读全文
相关推荐
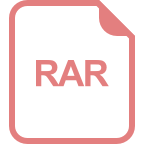
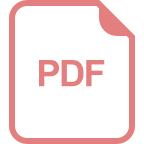
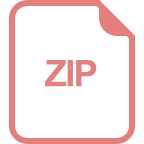
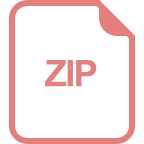
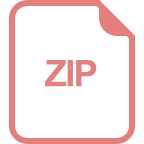
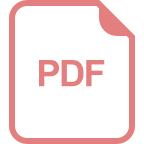
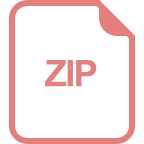
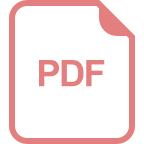
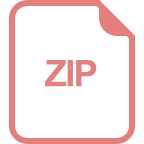
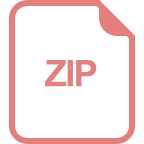
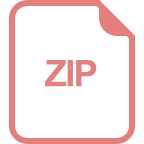
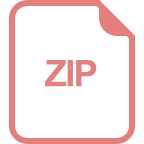
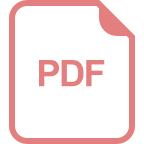
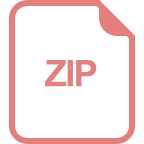
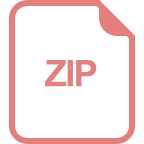