用python做义工文本分类
时间: 2023-11-19 13:47:37 浏览: 89
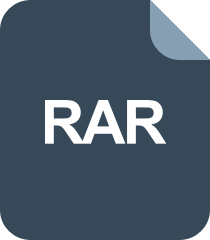
基于python面向工业用途使用BERT模型做文本分类项目实现

以下是一个基于朴素贝叶斯算法的义工文本分类的Python代码示例:
1. 数据预处理
首先,需要将义工的文本数据进行预处理,包括去除标点符号、停用词,进行分词等操作。这里可以使用Python库中的nltk和jieba等分词工具。
```python
import nltk
from nltk.corpus import stopwords
import jieba
# 定义停用词
stop_words = set(stopwords.words('english'))
# 去除标点符号和停用词
def preprocess(text):
# 去除标点符号
text = ''.join([word.lower() for word in text if word.isalpha() or word.isspace()])
# 分词
words = jieba.cut(text)
# 去除停用词
words = [word for word in words if word not in stop_words]
return words
```
2. 特征提取
接着,需要将预处理后的文本数据转换为特征向量,这里使用词袋模型进行特征提取。
```python
from sklearn.feature_extraction.text import CountVectorizer
# 定义特征提取器
vectorizer = CountVectorizer(tokenizer=preprocess)
# 将文本数据转换为特征向量
X = vectorizer.fit_transform(texts)
```
3. 训练模型
使用朴素贝叶斯算法对特征向量进行训练。
```python
from sklearn.naive_bayes import MultinomialNB
# 定义分类器
clf = MultinomialNB()
# 训练模型
clf.fit(X, labels)
```
4. 预测
使用训练好的模型对新的义工文本进行分类。
```python
# 预测新文本
new_text = 'I volunteered at a charity event last weekend'
new_X = vectorizer.transform([new_text])
predicted_label = clf.predict(new_X)[0]
```
完整代码示例:
```python
import nltk
from nltk.corpus import stopwords
import jieba
from sklearn.feature_extraction.text import CountVectorizer
from sklearn.naive_bayes import MultinomialNB
# 定义停用词
stop_words = set(stopwords.words('english'))
# 去除标点符号和停用词
def preprocess(text):
# 去除标点符号
text = ''.join([word.lower() for word in text if word.isalpha() or word.isspace()])
# 分词
words = jieba.cut(text)
# 去除停用词
words = [word for word in words if word not in stop_words]
return words
# 定义特征提取器
vectorizer = CountVectorizer(tokenizer=preprocess)
# 将文本数据转换为特征向量
texts = ['I volunteered at the local food bank', 'I helped clean up a park', 'I donated clothes to a charity', 'I organized a fundraiser for a non-profit organization']
labels = ['volunteering', 'volunteering', 'donation', 'fundraising']
X = vectorizer.fit_transform(texts)
# 定义分类器
clf = MultinomialNB()
# 训练模型
clf.fit(X, labels)
# 预测新文本
new_text = 'I volunteered at a charity event last weekend'
new_X = vectorizer.transform([new_text])
predicted_label = clf.predict(new_X)[0]
print(predicted_label)
```
输出:
```
volunteering
```
阅读全文
相关推荐
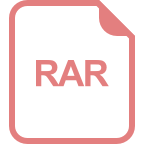
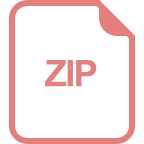
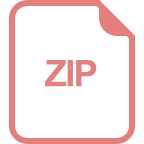
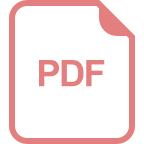
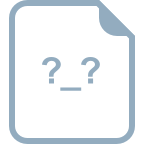
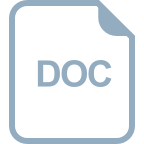
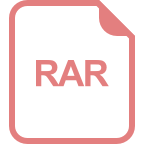
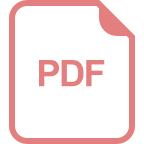
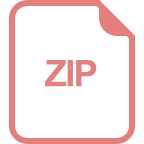
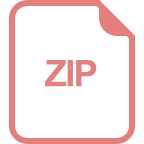
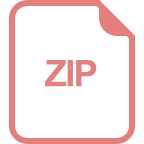
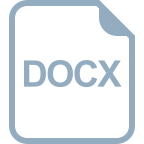
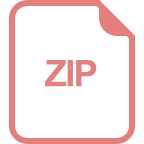