Csrnet模型加注意力机制代码
时间: 2023-06-25 08:06:12 浏览: 80
以下是使用 PyTorch 实现的 Csrnet 模型加注意力机制的代码:
```python
import torch
import torch.nn as nn
import torch.nn.functional as F
class AttentionBlock(nn.Module):
def __init__(self, in_channels, out_channels):
super(AttentionBlock, self).__init__()
self.conv1 = nn.Conv2d(in_channels, out_channels, kernel_size=1)
self.conv2 = nn.Conv2d(in_channels, out_channels, kernel_size=1)
self.conv3 = nn.Conv2d(in_channels, out_channels, kernel_size=1)
self.gamma = nn.Parameter(torch.zeros(1))
def forward(self, x):
batch_size, channels, height, width = x.size()
f = self.conv1(x).view(batch_size, -1, width * height)
g = self.conv2(x).view(batch_size, -1, width * height)
h = self.conv3(x).view(batch_size, -1, width * height)
s = torch.bmm(f.transpose(1, 2), g)
beta = F.softmax(s, dim=-1)
o = torch.bmm(h, beta.transpose(1, 2))
o = o.view(batch_size, channels, height, width)
x = self.gamma * o + x
return x
class Csrnet(nn.Module):
def __init__(self):
super(Csrnet, self).__init__()
self.conv1 = nn.Conv2d(3, 64, kernel_size=3, padding=1)
self.conv2 = nn.Conv2d(64, 64, kernel_size=3, padding=1)
self.pool1 = nn.MaxPool2d(kernel_size=2, stride=2)
self.conv3 = nn.Conv2d(64, 128, kernel_size=3, padding=1)
self.conv4 = nn.Conv2d(128, 128, kernel_size=3, padding=1)
self.pool2 = nn.MaxPool2d(kernel_size=2, stride=2)
self.conv5 = nn.Conv2d(128, 256, kernel_size=3, padding=1)
self.conv6 = nn.Conv2d(256, 256, kernel_size=3, padding=1)
self.conv7 = nn.Conv2d(256, 256, kernel_size=3, padding=1)
self.pool3 = nn.MaxPool2d(kernel_size=2, stride=2)
self.conv8 = nn.Conv2d(256, 512, kernel_size=3, padding=1)
self.conv9 = nn.Conv2d(512, 512, kernel_size=3, padding=1)
self.conv10 = nn.Conv2d(512, 512, kernel_size=3, padding=1)
self.pool4 = nn.MaxPool2d(kernel_size=2, stride=2)
self.conv11 = nn.Conv2d(512, 512, kernel_size=3, padding=1)
self.conv12 = nn.Conv2d(512, 512, kernel_size=3, padding=1)
self.conv13 = nn.Conv2d(512, 512, kernel_size=3, padding=1)
self.pool5 = nn.MaxPool2d(kernel_size=2, stride=2)
self.attention1 = AttentionBlock(64, 64)
self.attention2 = AttentionBlock(128, 128)
self.attention3 = AttentionBlock(256, 256)
self.attention4 = AttentionBlock(512, 512)
self.attention5 = AttentionBlock(512, 512)
self.fc1 = nn.Linear(512 * 7 * 7, 4096)
self.fc2 = nn.Linear(4096, 1024)
self.fc3 = nn.Linear(1024, 1)
def forward(self, x):
x = F.relu(self.conv1(x))
x = F.relu(self.conv2(x))
x = self.pool1(x)
x = self.attention1(x)
x = F.relu(self.conv3(x))
x = F.relu(self.conv4(x))
x = self.pool2(x)
x = self.attention2(x)
x = F.relu(self.conv5(x))
x = F.relu(self.conv6(x))
x = F.relu(self.conv7(x))
x = self.pool3(x)
x = self.attention3(x)
x = F.relu(self.conv8(x))
x = F.relu(self.conv9(x))
x = F.relu(self.conv10(x))
x = self.pool4(x)
x = self.attention4(x)
x = F.relu(self.conv11(x))
x = F.relu(self.conv12(x))
x = F.relu(self.conv13(x))
x = self.pool5(x)
x = self.attention5(x)
x = x.view(-1, 512 * 7 * 7)
x = F.relu(self.fc1(x))
x = F.relu(self.fc2(x))
x = self.fc3(x)
return x
```
其中,AttentionBlock 类定义了注意力机制的操作,Csrnet 类定义了 Csrnet 模型的结构,同时在模型中加入了五个 AttentionBlock,分别应用于 Csrnet 中的五个卷积块。
相关推荐
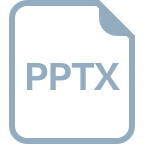













