with open(config_file, 's', encoding='utf-8') as f:
时间: 2023-12-23 17:06:13 浏览: 26
这段代码中的 's' 是一个无效的文件打开模式,应该改为 'w' 才能以写模式打开文件。因此,应该这样写:
```
with open(config_file, 'w', encoding='utf-8') as f:
```
这样才能正确地打开文件并进行写操作。同时,上下文管理器 with 会确保在写入完成后自动关闭文件,以避免文件资源泄露或损坏。
相关问题
def open_file(self): config_file = 'config/fold.json' # config = json.load(open(config_file, 'r', encoding='utf-8')) config = json.load(open(config_file, 'r', encoding='utf-8')) open_fold = config['open_fold'] if not os.path.exists(open_fold): open_fold = os.getcwd() name, _ = QFileDialog.getOpenFileName(self, 'Video/image', open_fold, "Pic File(*.mp4 *.mkv *.avi *.flv " "*.jpg *.png)") if name: self.det_thread.source = name # self.statistic_msg('Loaded file:{}'.format(os.path.basename(name))) config['open_fold'] = os.path.dirname(name) config_json = json.dumps(config, ensure_ascii=False, indent=2) with open(config_file, 'w', encoding='utf-8') as f: f.write(config_json) self.stop()
这段代码是一个 PyQt5 中的方法,它实现了打开文件对话框,并读取配置文件中保存的上一次打开的文件夹路径(如果没有,则默认为当前工作目录),用于初始化文件对话框中的路径。
如果用户选择了一个文件,则将该文件路径设置为 self.det_thread.source 的值,并将配置文件中保存的上一次打开的文件夹路径更新为该文件所在的文件夹路径。如果用户没有选择文件,则不会进行任何操作。最后,将更新后的配置文件保存回磁盘。
其中,self.det_thread 是一个 QThread 的子类,用于在后台线程中运行视频检测算法。self.det_thread.source 是用于存储视频文件路径的成员变量。
def chose_rtsp(self): self.rtsp_window = Window() config_file = 'config/ip.json' if not os.path.exists(config_file): ip = "rtsp://admin:admin888@192.168.1.67:555" new_config = {"ip": ip} new_json = json.dumps(new_config, ensure_ascii=False, indent=2) with open(config_file, 'w', encoding='utf-8') as f: f.write(new_json) else: config = json.load(open(config_file, 'r', encoding='utf-8')) ip = config['ip'] self.rtsp_window.rtspEdit.setText(ip) self.rtsp_window.show() self.rtsp_window.rtspButton.clicked.connect(lambda: self.load_rtsp(self.rtsp_window.rtspEdit.text()))
这段代码是一个函数,它的功能是让用户选择一个 RTSP 地址,然后加载这个地址的视频流。具体来说,它会先创建一个窗口对象,并将窗口显示出来。然后,它会读取一个配置文件,如果这个配置文件不存在,它会将一个默认的 RTSP 地址写入配置文件中。接着,它会从配置文件中读取 RTSP 地址,并将这个地址显示在窗口中的文本框中。最后,它会连接一个点击事件,当用户点击“确认”按钮时,会调用 `load_rtsp` 函数来加载用户选择的 RTSP 地址的视频流。
相关推荐
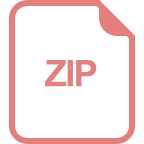
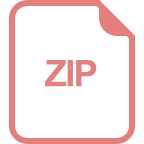
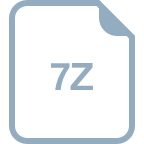












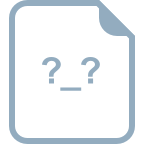