将下面的缺失值填充处理代码优化并写出代码过程 update_value = [] for key in ['pm10', '温度', '湿度', '风速', '风向']: # 插值填充缺失数据 col = e[key].copy() bool_na = col.isna().copy() for (idx, na) in enumerate(bool_na.to_list()): if na: pre_value = post_value = None pre_index = post_index = idx while pre_value is None and idx > 0: pre_index -= 1 if pre_index == 0: break if not bool_na.iloc[pre_index]: pre_value = col.iloc[pre_index] while post_value is None and idx < (len(col) - 1): post_index += 1 if post_index == len(col): break if not bool_na.iloc[post_index]: post_value = col.iloc[post_index] if pre_value is not None and post_value is not None: col.iloc[idx] = (pre_value + post_value) / 2 bool_na.iloc[idx] = False update_value.append((idx, key, col.iloc[idx])) for (idx, key, v) in update_value: e.loc[idx, key] = v
时间: 2024-02-10 21:12:08 浏览: 70
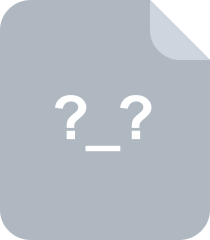
缺失值处理
这段代码可以进行如下优化:
1. 使用 pandas 内置的插值函数进行填充,可以使用 interpolate() 函数或 bfill() 和 ffill() 函数进行填充,可以根据数据的特性选择不同的插值方法。
2. 可以使用 apply() 函数对列进行操作,避免使用循环。
下面是代码优化后的过程:
1. 导入 pandas 库并读取数据
```python
import pandas as pd
e = pd.read_csv('data.csv')
```
2. 使用 interpolate() 函数进行填充
```python
e.interpolate(inplace=True)
```
3. 使用 apply() 函数对列进行操作
```python
update_value = []
def fill_na(col):
bool_na = col.isna().copy()
for (idx, na) in enumerate(bool_na.to_list()):
if na:
pre_value = post_value = None
pre_index = post_index = idx
while pre_value is None and idx > 0:
pre_index -= 1
if pre_index == 0:
break
if not bool_na.iloc[pre_index]:
pre_value = col.iloc[pre_index]
while post_value is None and idx < (len(col) - 1):
post_index += 1
if post_index == len(col):
break
if not bool_na.iloc[post_index]:
post_value = col.iloc[post_index]
if pre_value is not None and post_value is not None:
col.iloc[idx] = (pre_value + post_value) / 2
bool_na.iloc[idx] = False
update_value.append((idx, col.name, col.iloc[idx]))
for key in ['pm10', '温度', '湿度', '风速', '风向']:
e[key].apply(fill_na)
for (idx, key, v) in update_value:
e.loc[idx, key] = v
```
阅读全文
相关推荐
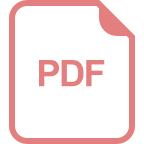
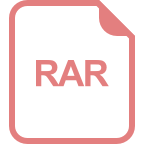
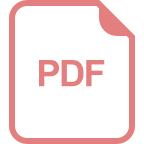
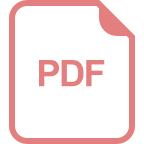
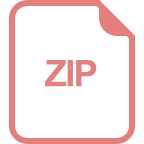
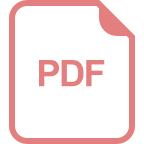
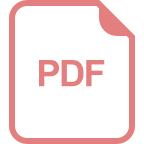
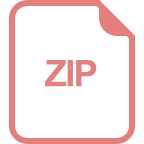
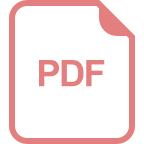
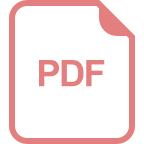
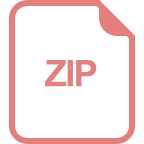
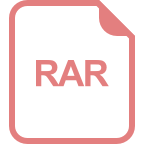
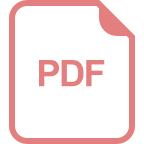
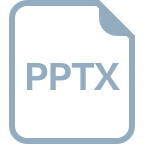
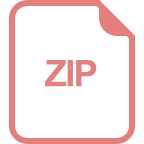
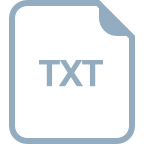