解释一下这段代码:loss = tf.reduce_mean(tf.nn.nce_loss( nce_weights, nce_biases, embed, train_labels, num_sampled, vocabulary_size))
时间: 2023-04-02 20:02:10 浏览: 78
这段代码是 TensorFlow 中用于计算 NCE loss 的函数。NCE loss 是一种用于训练神经网络的损失函数,它可以用于训练词向量模型。在这段代码中,我们需要提供一些参数,包括 NCE 权重、NCE 偏置、嵌入向量、训练标签、采样数量和词汇表大小。函数会根据这些参数计算出 NCE loss,并返回一个标量值作为损失。
相关问题
select * from SAJET.G_SN_CELL_PARAMS WHERE SERIAL_NUMBER='03NCE00NFN76CSD5J4241692';
由于我是一个AI模型,无法直接访问数据库。但是你可以使用适当的数据库客户端(如MySQL、Oracle等)连接到数据库,并执行你提供的SQL查询。
在你的查询中,你正在选择`SAJET.G_SN_CELL_PARAMS`表中`SERIAL_NUMBER`列值为'03NCE00NFN76CSD5J4241692'的所有行。请确保你有正确的数据库连接信息和适当的访问权限,以便成功执行查询。
python 实现skip-gram训练
Skip-gram 是一种常用的词向量训练方法,它通过预测一个词周围的词来学习每个词的向量表示。下面是使用 Python 实现 Skip-gram 训练的简单示例。
首先,我们需要导入必要的库:
```python
import numpy as np
import tensorflow as tf
from collections import Counter
import random
```
然后,我们需要定义一些参数:
```python
# 语料库文件路径
corpus_file = 'corpus.txt'
# 词向量维度
embedding_size = 128
# 跳跃窗口大小
window_size = 5
# 负样本数
num_neg_samples = 64
# 学习率
learning_rate = 0.1
# 迭代次数
num_iterations = 10000
# 每隔多少步输出一次日志
log_interval = 1000
```
接下来,我们需要读取语料库文件,并将其中的词转换成数字编码:
```python
with open(corpus_file, 'r', encoding='utf-8') as f:
corpus = f.read().split()
# 统计词频并按照词频从高到低排序
word_counts = Counter(corpus)
sorted_vocab = sorted(word_counts, key=word_counts.get, reverse=True)
# 生成词汇表和词汇表的反向映射表
vocab_to_int = {word: idx for idx, word in enumerate(sorted_vocab)}
int_to_vocab = {idx: word for idx, word in enumerate(sorted_vocab)}
# 将语料库中的词转换成数字编码
corpus_int = [vocab_to_int[word] for word in corpus]
```
之后,我们需要定义 Skip-gram 模型的输入和输出:
```python
inputs = tf.placeholder(tf.int32, [None], name='inputs')
labels = tf.placeholder(tf.int32, [None, 1], name='labels')
```
然后,我们需要定义词向量矩阵,这个矩阵的维度是词汇表大小 × 词向量维度:
```python
vocab_size = len(vocab_to_int)
embedding = tf.Variable(tf.random_uniform([vocab_size, embedding_size], -1.0, 1.0))
```
接下来,我们需要定义损失函数。具体来说,我们用负对数似然损失函数来最小化预测概率和真实值之间的距离,同时使用负样本来训练模型:
```python
nce_weights = tf.Variable(tf.truncated_normal([vocab_size, embedding_size], stddev=1.0 / np.sqrt(embedding_size)))
nce_biases = tf.Variable(tf.zeros([vocab_size]))
embed = tf.nn.embedding_lookup(embedding, inputs)
loss = tf.reduce_mean(tf.nn.nce_loss(nce_weights, nce_biases, labels, embed, num_neg_samples, vocab_size))
```
最后,我们需要使用梯度下降优化器来最小化损失函数,并在训练过程中输出日志:
```python
optimizer = tf.train.GradientDescentOptimizer(learning_rate).minimize(loss)
with tf.Session() as sess:
sess.run(tf.global_variables_initializer())
for i in range(num_iterations):
# 生成训练样本
batch_inputs, batch_labels = generate_batch(corpus_int, window_size, num_neg_samples)
# 训练模型
feed_dict = {inputs: batch_inputs, labels: batch_labels}
_, loss_val = sess.run([optimizer, loss], feed_dict=feed_dict)
# 输出日志
if (i + 1) % log_interval == 0:
print('Iteration {}: Loss = {:.4f}'.format(i + 1, loss_val))
```
完整代码如下:
```python
import numpy as np
import tensorflow as tf
from collections import Counter
import random
# 语料库文件路径
corpus_file = 'corpus.txt'
# 词向量维度
embedding_size = 128
# 跳跃窗口大小
window_size = 5
# 负样本数
num_neg_samples = 64
# 学习率
learning_rate = 0.1
# 迭代次数
num_iterations = 10000
# 每隔多少步输出一次日志
log_interval = 1000
def generate_batch(corpus, window_size, num_neg_samples):
# 遍历整个语料库
for i in range(window_size, len(corpus) - window_size):
# 输入词
center_word = corpus[i]
# 输出词
context_words = []
for j in range(i - window_size, i + window_size + 1):
if j != i:
context_words.append(corpus[j])
# 负样本
neg_samples = []
while len(neg_samples) < num_neg_samples:
samp = random.randint(0, len(corpus) - 1)
if samp != center_word and samp not in context_words:
neg_samples.append(samp)
yield center_word, context_words + neg_samples
with open(corpus_file, 'r', encoding='utf-8') as f:
corpus = f.read().split()
# 统计词频并按照词频从高到低排序
word_counts = Counter(corpus)
sorted_vocab = sorted(word_counts, key=word_counts.get, reverse=True)
# 生成词汇表和词汇表的反向映射表
vocab_to_int = {word: idx for idx, word in enumerate(sorted_vocab)}
int_to_vocab = {idx: word for idx, word in enumerate(sorted_vocab)}
# 将语料库中的词转换成数字编码
corpus_int = [vocab_to_int[word] for word in corpus]
inputs = tf.placeholder(tf.int32, [None], name='inputs')
labels = tf.placeholder(tf.int32, [None, 1], name='labels')
vocab_size = len(vocab_to_int)
embedding = tf.Variable(tf.random_uniform([vocab_size, embedding_size], -1.0, 1.0))
nce_weights = tf.Variable(tf.truncated_normal([vocab_size, embedding_size], stddev=1.0 / np.sqrt(embedding_size)))
nce_biases = tf.Variable(tf.zeros([vocab_size]))
embed = tf.nn.embedding_lookup(embedding, inputs)
loss = tf.reduce_mean(tf.nn.nce_loss(nce_weights, nce_biases, labels, embed, num_neg_samples, vocab_size))
optimizer = tf.train.GradientDescentOptimizer(learning_rate).minimize(loss)
with tf.Session() as sess:
sess.run(tf.global_variables_initializer())
for i in range(num_iterations):
# 生成训练样本
batch_inputs, batch_labels = generate_batch(corpus_int, window_size, num_neg_samples)
# 训练模型
feed_dict = {inputs: batch_inputs, labels: batch_labels}
_, loss_val = sess.run([optimizer, loss], feed_dict=feed_dict)
# 输出日志
if (i + 1) % log_interval == 0:
print('Iteration {}: Loss = {:.4f}'.format(i + 1, loss_val))
```
注意,这只是一个简单的示例,实际上 Skip-gram 模型中还可以加入很多其他的优化和技巧。
相关推荐
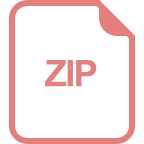












