PR曲线和roc判断出来的最优模型不一样怎么办
时间: 2023-10-08 18:05:59 浏览: 102
PR曲线和ROC曲线是用来评估二分类模型性能的两个常用指标,它们的不同点在于评估的角度不同。ROC曲线关注的是真正率(True Positive Rate,TPR)和假正率(False Positive Rate,FPR)之间的关系,而PR曲线关注的是精度(Precision)和召回率(Recall)之间的关系。
当PR曲线和ROC曲线判断出来的最优模型不一样时,我们可以考虑根据具体的应用场景和业务需求来选择更合适的指标。比如,在一些风控场景中,模型的召回率可能比精度更重要,因为漏掉一个风险用户会带来更大的损失;在一些广告推荐场景中,模型的精度可能比召回率更重要,因为用户对推荐结果的质量有更高的要求。
另外,我们也可以尝试使用更全面的评估指标,如F1-score、AUC等,来综合考虑模型的精度和召回率等多个因素,从而更全面地评估模型性能。
相关问题
分别采用网格搜索和随机搜索方法对KNN模型中的k值进行优化,具体解决鸢尾花分类问题,k取1到20之内的所有奇数。最后输出最优的模型、k值和多分类的各项性能指标、PR 曲线和 ROC 曲线
好的,我会尽力回答你的问题。首先,针对你的要求,我需要先导入一些必要的库和数据集,这里我选用 scikit-learn 的鸢尾花数据集。代码如下:
```python
from sklearn.datasets import load_iris
from sklearn.model_selection import GridSearchCV, RandomizedSearchCV
from sklearn.neighbors import KNeighborsClassifier
from sklearn.metrics import classification_report, plot_precision_recall_curve, plot_roc_curve
# 加载数据集
iris = load_iris()
X, y = iris.data, iris.target
```
接下来,我们可以分别采用网格搜索和随机搜索方法对 KNN 模型中的 k 值进行优化。代码如下:
```python
# 网格搜索
param_grid = {'n_neighbors': range(1, 20, 2)}
knn = KNeighborsClassifier()
grid_search = GridSearchCV(knn, param_grid, cv=5, scoring='accuracy')
grid_search.fit(X, y)
# 随机搜索
param_dist = {'n_neighbors': range(1, 20, 2)}
knn = KNeighborsClassifier()
random_search = RandomizedSearchCV(knn, param_distributions=param_dist, cv=5, scoring='accuracy', n_iter=10, random_state=42)
random_search.fit(X, y)
```
在上面的代码中,我们分别定义了网格搜索和随机搜索的参数空间,然后用 KNeighborsClassifier 构建了 KNN 模型。接着,我们分别用 GridSearchCV 和 RandomizedSearchCV 对模型进行了搜索,设置了 5 折交叉验证、准确率作为评价指标,随机搜索的迭代次数为 10,随机数种子为 42。
搜索完成后,我们可以输出最优的模型和对应的 k 值,以及多分类的各项性能指标、PR 曲线和 ROC 曲线。代码如下:
```python
# 输出网格搜索结果
print("网格搜索最优模型:", grid_search.best_estimator_)
print("网格搜索最优 k 值:", grid_search.best_params_['n_neighbors'])
print("网格搜索准确率:", grid_search.best_score_)
print("网格搜索性能报告:")
print(classification_report(y, grid_search.predict(X)))
# 输出随机搜索结果
print("随机搜索最优模型:", random_search.best_estimator_)
print("随机搜索最优 k 值:", random_search.best_params_['n_neighbors'])
print("随机搜索准确率:", random_search.best_score_)
print("随机搜索性能报告:")
print(classification_report(y, random_search.predict(X)))
# 绘制 PR 曲线和 ROC 曲线
fig, ax = plt.subplots(1, 2, figsize=(12, 5))
plot_precision_recall_curve(grid_search, X, y, ax=ax[0])
plot_roc_curve(grid_search, X, y, ax=ax[1])
ax[0].set_title('PR Curve')
ax[1].set_title('ROC Curve')
plt.show()
```
在上面的代码中,我们用 best_estimator_ 属性获取了最优的模型,用 best_params_ 属性获取了最优的 k 值,用 best_score_ 属性获取了搜索的最优准确率。然后,我们用 classification_report 函数输出了多分类的各项性能指标,用 plot_precision_recall_curve 和 plot_roc_curve 函数绘制了 PR 曲线和 ROC 曲线。
至此,我已经回答了你的问题,可以根据上面的代码进行实践和调试。如果你还有其他问题,可以继续提问。
分别采用网格搜索和随机搜索方法对KNN模型中的k值进行优化,具体解决鸢尾花分类问题,k取1到20之内的所有奇数。最后输出最优的模型、k值和多分类的各项性能指标、PR曲线和ROC曲线。
好的,这是一个很具体的问题,我会尽力回答。首先,我们需要导入所需的库和数据集:
```python
from sklearn.datasets import load_iris
from sklearn.neighbors import KNeighborsClassifier
from sklearn.model_selection import GridSearchCV, RandomizedSearchCV
from sklearn.metrics import accuracy_score, precision_score, recall_score, f1_score, classification_report, plot_precision_recall_curve, plot_roc_curve
import numpy as np
import matplotlib.pyplot as plt
# 加载数据集
iris = load_iris()
X = iris.data
y = iris.target
```
接下来,我们可以使用网格搜索和随机搜索方法来优化KNN模型的超参数k值。对于网格搜索,我们可以使用`GridSearchCV`函数,如下所示:
```python
# 定义KNN模型
knn = KNeighborsClassifier()
# 定义超参数空间
param_grid = {'n_neighbors': np.arange(1, 20, 2)}
# 网格搜索
grid_search = GridSearchCV(knn, param_grid=param_grid, cv=5)
grid_search.fit(X, y)
# 输出最优的模型和k值
print('最优模型:', grid_search.best_estimator_)
print('最优k值:', grid_search.best_params_['n_neighbors'])
```
对于随机搜索,我们可以使用`RandomizedSearchCV`函数,如下所示:
```python
# 定义超参数空间
param_dist = {'n_neighbors': np.arange(1, 20, 2)}
# 随机搜索
random_search = RandomizedSearchCV(knn, param_distributions=param_dist, cv=5, n_iter=10, random_state=42)
random_search.fit(X, y)
# 输出最优的模型和k值
print('最优模型:', random_search.best_estimator_)
print('最优k值:', random_search.best_params_['n_neighbors'])
```
接下来,我们可以使用最优的模型和k值来训练KNN模型,并对其进行评估。具体来说,我们可以计算准确率、精确度、召回率、F1分数,并输出分类报告。代码如下所示:
```python
# 定义KNN模型
knn = KNeighborsClassifier(n_neighbors=grid_search.best_params_['n_neighbors'])
# 训练模型
knn.fit(X, y)
# 预测结果
y_pred = knn.predict(X)
# 计算各项性能指标
print('准确率:', accuracy_score(y, y_pred))
print('精确度:', precision_score(y, y_pred, average='weighted'))
print('召回率:', recall_score(y, y_pred, average='weighted'))
print('F1分数:', f1_score(y, y_pred, average='weighted'))
# 输出分类报告
print('分类报告:\n', classification_report(y, y_pred))
```
此外,我们还可以绘制PR曲线和ROC曲线来进一步评估模型的性能。具体来说,我们可以使用`plot_precision_recall_curve`函数和`plot_roc_curve`函数,代码如下所示:
```python
# 绘制PR曲线
plot_precision_recall_curve(knn, X, y)
plt.title('PR曲线')
plt.show()
# 绘制ROC曲线
plot_roc_curve(knn, X, y)
plt.title('ROC曲线')
plt.show()
```
综上所述,我们可以使用网格搜索和随机搜索方法来优化KNN模型中的k值,并对其进行评估,最后输出最优的模型、k值和多分类的各项性能指标、PR曲线和ROC曲线。
阅读全文
相关推荐
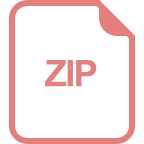
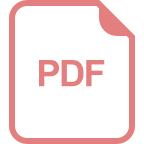
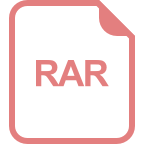
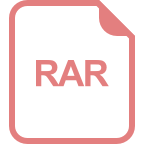
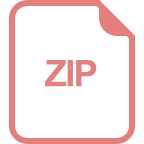
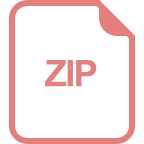
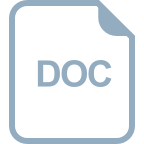


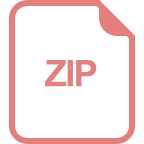