机器学习逻辑回归分类算法代码
时间: 2023-09-26 19:07:09 浏览: 138
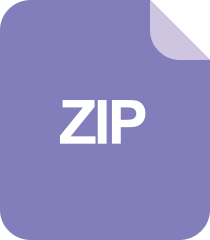
使用机器学习算法自动进行音乐流派分类,例如逻辑回归和 K-最近邻_python_代码_下载

### 回答1:
下面是一个简单的逻辑回归分类算法的 Python 代码示例:
```python
import numpy as np
class LogisticRegression:
def __init__(self, learning_rate=0.01, num_iterations=100000, fit_intercept=True):
self.learning_rate = learning_rate
self.num_iterations = num_iterations
self.fit_intercept = fit_intercept
def sigmoid(self, z):
return 1 / (1 + np.exp(-z))
def fit(self, X, y):
if self.fit_intercept:
intercept = np.ones((X.shape[0], 1))
X = np.hstack((intercept, X))
# 初始化权重
self.theta = np.zeros(X.shape[1])
for i in range(self.num_iterations):
z = np.dot(X, self.theta)
h = self.sigmoid(z)
gradient = np.dot(X.T, (h - y)) / y.size
self.theta -= self.learning_rate * gradient
def predict_prob(self, X):
if self.fit_intercept:
intercept = np.ones((X.shape[0], 1))
X = np.hstack((intercept, X))
return self.sigmoid(np.dot(X, self.theta))
def predict(self, X, threshold=0.5):
return self.predict_prob(X) >= threshold
```
这里我们定义了一个 `LogisticRegression` 类,它有以下几个方法:
- `__init__`:初始化逻辑回归模型的超参数,包括学习率、迭代次数和是否拟合截距项。
- `sigmoid`:sigmoid 函数,用于将线性函数的输出转换为概率值。
- `fit`:训练模型的方法,使用梯度下降算法来更新权重。
- `predict_prob`:给定输入数据,返回模型预测的类别概率。
- `predict`:给定输入数据和阈值,返回模型预测的类别。
在使用时,首先需要创建一个 `LogisticRegression` 类的实例,然后调用 `fit` 方法来训练模型。训练完成后,可以使用 `predict_prob` 方法来预测类别的概率,或使用 `predict` 方法来预测具体的类别。
### 回答2:
逻辑回归是一种常用的机器学习算法,用于解决二分类问题。其基本原理是利用线性回归模型的预测结果通过一个sigmoid函数转换成0或1的概率值进行分类预测。
以下是一个简单的机器学习逻辑回归分类算法代码:
1. 导入所需的库:
```python
import numpy as np
from sklearn.linear_model import LogisticRegression
```
2. 准备数据:
```python
X = np.array([[x1, x2], [x1, x2], ..., [x1, x2]]) # 特征矩阵,每行代表一个样本的特征向量
y = np.array([y1, y2, ..., yn]) # 标签向量,表示每个样本的类别
```
3. 创建逻辑回归模型对象:
```python
model = LogisticRegression()
```
4. 使用训练数据进行模型训练:
```python
model.fit(X, y)
```
5. 对新样本进行分类预测:
```python
new_sample = np.array([x1, x2]) # 待预测的新样本的特征向量
predicted_class = model.predict([new_sample]) # 预测样本的类别
```
以上是一个简单的机器学习逻辑回归分类算法的代码实现。要注意的是,在实际应用中,可能需要进行特征工程、数据预处理、模型评估等步骤来提高分类效果。此外,可以通过调整模型的参数,如正则化系数等,来优化模型的性能。
阅读全文
相关推荐
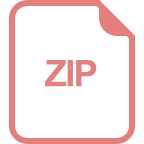
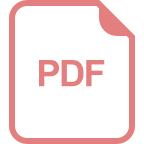
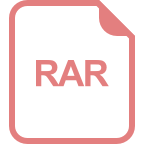

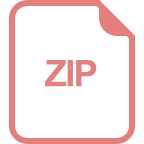
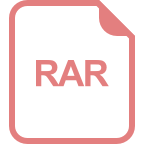