python 实现SPIMI算法
时间: 2023-10-16 15:11:32 浏览: 123
Sure, Python can be used to implement the SPIMI algorithm for information retrieval. The SPIMI algorithm stands for Single-Pass In-Memory Indexing, which is used to build an inverted index for a large corpus by processing the documents one at a time. It uses the concept of block-based indexing and is widely used in search engines.
相关问题
python实现spimi算法
SPIMI(Single Pass In-Memory Indexing)算法是一种基于内存的索引构建算法,用于处理大规模文本数据集。它的实现比较简单,可以使用Python来实现。
SPIMI算法的实现步骤如下:
1. 将文本数据集分块,每个块可以是一个文件或者一个固定大小的数据块。
2. 对于每个块,使用分词器将其分词成单词,并将每个单词与其所在文档的标识符一起存储到一个字典中。
3. 当字典大小达到一定阈值时,将字典写入磁盘。
4. 对于每个单词,维护一个有序列表,记录它出现在哪些文档中。
5. 最后,使用类似归并排序的方法合并所有块的索引。
下面是一个简单的Python实现,假设有一个名为“data.txt”的大规模文本数据集,每个数据块大小为100个单词:
```python
from collections import defaultdict
def spimi_invert(filename, block_size):
"""SPIMI algorithm implementation for inverted indexing"""
# initialize an empty dictionary for the inverted index
inverted_index = defaultdict(list)
# open the input file
with open(filename, 'r') as input_file:
block = []
block_size_bytes = 0
for line in input_file:
# tokenize the line into words
words = line.strip().split()
for word in words:
# add the word to the current block
block.append((word, input_file.tell()))
block_size_bytes += len(word)
# if the block is full, write it to disk and clear the memory
if block_size_bytes >= block_size:
inverted_index = spimi_merge(inverted_index, block)
block = []
block_size_bytes = 0
# process the last block
if block:
inverted_index = spimi_merge(inverted_index, block)
return inverted_index
def spimi_merge(inverted_index, block):
"""Merge a block into an inverted index built so far"""
# sort the block by the word
block.sort(key=lambda x: x[0])
# initialize a pointer array for each word in the block
pointers = {}
for i, (word, _) in enumerate(block):
if word not in pointers:
pointers[word] = []
pointers[word].append(i)
# merge the block with the inverted index
for word, indices in pointers.items():
postings = []
for index in indices:
_, doc_id = block[index]
postings.append(doc_id)
inverted_index[word].extend(postings)
return inverted_index
inverted_index = spimi_invert('data.txt', 100)
print(inverted_index)
```
这里的`spimi_invert`函数实现了SPIMI算法,输入参数为文本数据集的文件名和块大小,返回值是一个字典,键为单词,值为该单词出现的文档标识符列表。函数内部使用`spimi_merge`函数将每个块合并到字典中,`spimi_merge`函数接受一个已有的倒排索引和一个数据块,并返回合并后的倒排索引。
python实现SPIMI算法
SPIMI(单遍多插入)算法是一种用于处理大型文本数据的索引构建算法,通常用于信息检索系统中。在Python中,我们可以使用以下步骤实现SPIMI算法:
1. 定义一个空的索引(index)字典。
2. 从输入数据中读取每个文档(document)。
3. 对于每个文档,将其拆分为词元(term)。可以使用Python中的split()函数来实现。
4. 对于每个词元,检查它是否已经在索引字典中出现。如果词元已经存在,则更新其出现文档列表(posting list),否则将其添加到索引字典中,并将其出现文档列表初始化为空列表。
5. 继续读取文档,直到所有文档都处理完毕。
6. 将索引字典写入磁盘文件中。
以下是一个示例代码:
```
import os
import re
def spimi(inverted_idx, doc_id, token_list):
for token in token_list:
if token in inverted_idx:
if doc_id not in inverted_idx[token]:
inverted_idx[token].append(doc_id)
else:
inverted_idx[token] = [doc_id]
def tokenize(text):
token_pattern = re.compile(r'\w+')
return token_pattern.findall(text.lower())
def spimi_invert(docs_dir):
inverted_idx = {}
doc_id = 0
buffer = {}
buffer_size = 1000
for filename in os.listdir(docs_dir):
with open(os.path.join(docs_dir, filename), 'r') as file:
tokens = tokenize(file.read())
spimi(buffer, doc_id, tokens)
doc_id += 1
if len(buffer) >= buffer_size:
for term in buffer:
if term in inverted_idx:
inverted_idx[term].extend(buffer[term])
else:
inverted_idx[term] = list(buffer[term])
buffer = {}
for term in buffer:
if term in inverted_idx:
inverted_idx[term].extend(buffer[term])
else:
inverted_idx[term] = list(buffer[term])
return inverted_idx
docs_dir = './docs'
inverted_idx = spimi_invert(docs_dir)
print(inverted_idx)
```
在这个示例中,我们遍历了一个包含多个文档的文件夹,将每个文档拆分为词元并在SPIMI算法中处理它们。对于每个词元,我们使用一个字典(buffer)缓存它们的出现文档列表。当缓存字典的大小达到一定值(buffer_size)时,我们将其合并到总的倒排索引字典(inverted_idx)中。最后,我们返回完整的倒排索引字典。
阅读全文
相关推荐
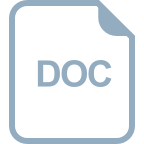
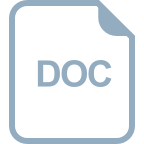
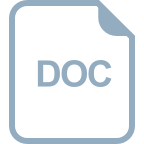




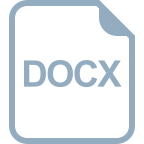
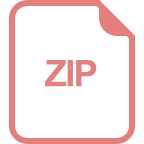
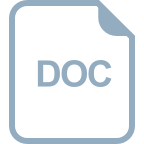


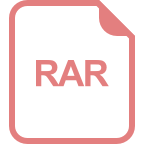
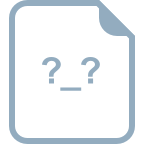
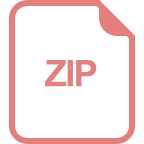

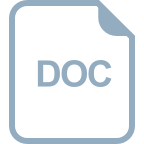