myimage = cv.cvtColor(img, cv.COLOR_BGR2GRAY) ret, img1 = cv.threshold(myimage, 100, 255, cv.THRESH_BINARY_INV) # cv.namedWindow('img1',0) # cv.resizeWindow('img1',600,600) # cv.imshow('img1',img1) # print(type(img1)) # print(img1.shape) # print(img1.size) # cv.waitKey(2) kernel1 = np.ones((10, 10), np.uint8) # 做一次膨胀 img2 = cv.dilate(img1, kernel1) # cv.namedWindow('img2', 0) # cv.resizeWindow('img2', 600, 600) # cv.imshow('img2', img2) contours, hierarchy = cv.findContours(img2, cv.RETR_EXTERNAL, cv.CHAIN_APPROX_NONE) # print(len(contours),hierarchy) for i in range(len(contours)): area = cv.contourArea(contours[i]) if area < 150: # '设定连通域最小阈值,小于该值被清理' cv.drawContours(img2, [contours[i]], 0, 0, -1) # import pdb;pdb.set_trace() # cv.imwrite('yuchuli.jpg', img2) ###########预处理 # import pdb;pdb.set_trace() not_row = img2[[not np.all(img2[i] == 0) for i in range(img2.shape[0])], :] bot_col = not_row[:, [not np.all(not_row[:, i] == 0) for i in range(not_row.shape[1])]] # import pdb;pdb.set_trace() # print(bot_col.shape) if bot_col.shape[0] > bot_col.shape[1]: if bot_col.shape[1] % 2 == 0: img_new = np.concatenate((np.zeros([bot_col.shape[0], int((bot_col.shape[0] - bot_col.shape[1]) / 2)]), bot_col, np.zeros([bot_col.shape[0], int((bot_col.shape[0] - bot_col.shape[1]) / 2)])), 1) if bot_col.shape[1] % 2 == 1: img_new = np.concatenate((np.zeros( [bot_col.shape[0], int((bot_col.shape[0] - bot_col.shape[1] - 1) / 2)]), bot_col, np.zeros( [bot_col.shape[0], int((bot_col.shape[0] - bot_col.shape[1] + 1) / 2)])), 1) cv.imwrite('fenge.jpg', img_new) ###########分割 file_path = 'fenge.jpg' return file_path这个具体以何种方法进行分割的
时间: 2024-01-19 19:02:09 浏览: 28
这段代码使用了 OpenCV 库中的图像处理函数实现了字符图片的分割。下面是代码的具体实现细节:
首先,将彩色图像转换为灰度图像,并使用二值化方法将图像转换为黑白图像。
```python
myimage = cv.cvtColor(img, cv.COLOR_BGR2GRAY)
ret, img1 = cv.threshold(myimage, 100, 255, cv.THRESH_BINARY_INV)
```
接着,使用膨胀操作将字符区域扩大。
```python
kernel1 = np.ones((10, 10), np.uint8) # 做一次膨胀
img2 = cv.dilate(img1, kernel1)
```
然后,使用 `findContours` 函数寻找图像中的轮廓,并根据轮廓的面积进行过滤,去除面积过小的连通域。
```python
contours, hierarchy = cv.findContours(img2, cv.RETR_EXTERNAL, cv.CHAIN_APPROX_NONE)
for i in range(len(contours)):
area = cv.contourArea(contours[i])
if area < 150:
cv.drawContours(img2, [contours[i]], 0, 0, -1)
```
接下来,根据字符区域的位置,将字符图片进行裁剪,得到多个字符图片。
```python
not_row = img2[[not np.all(img2[i] == 0) for i in range(img2.shape[0])], :]
bot_col = not_row[:, [not np.all(not_row[:, i] == 0) for i in range(not_row.shape[1])]]
if bot_col.shape[0] > bot_col.shape[1]:
if bot_col.shape[1] % 2 == 0:
img_new = np.concatenate((np.zeros([bot_col.shape[0], int((bot_col.shape[0] - bot_col.shape[1]) / 2)]),
bot_col,
np.zeros([bot_col.shape[0], int((bot_col.shape[0] - bot_col.shape[1]) / 2)])),
1)
if bot_col.shape[1] % 2 == 1:
img_new = np.concatenate((np.zeros(
[bot_col.shape[0], int((bot_col.shape[0] - bot_col.shape[1] - 1) / 2)]), bot_col, np.zeros(
[bot_col.shape[0], int((bot_col.shape[0] - bot_col.shape[1] + 1) / 2)])), 1)
```
最后,将裁剪后的字符图片保存到文件中,并返回文件路径。
```python
cv.imwrite('fenge.jpg', img_new)
file_path = 'fenge.jpg'
return file_path
```
整个过程中使用的方法包括二值化、膨胀、轮廓检测和裁剪等,这些方法常用于字符图片的处理和识别。
相关推荐
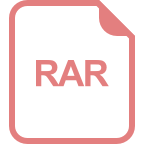














