import lightgbm as lgb from lightgbm import LGBMClassifier from sklearn.metrics import accuracy_score from sklearn.model_selection import cross_val_score from sklearn.model_selection import GridSearchCV # 定义xgboost模型 lgb_model = lgb.LGBMClassifier() # 设置参数空间 params = { 'boosting_type': 'gbdt', #'boosting_type': 'dart', 'objective': 'multiclass', 'metric': 'multi_logloss', 'min_child_weight': 1.5, 'num_leaves': 2**5, 'lambda_l2': 10, 'subsample': 0.7, 'colsample_bytree': 0.7, 'learning_rate': 0.03, 'seed': 2017, "num_class": 2, 'silent': True, } # 输出最优参数 print('Best Parameter:', params) # 使用最优参数构建新的xgboost模型 lgb_model = lgb.LGBMClassifier(**params) # 使用训练集对新模型进行拟合 # X_train = np.array(X_train) # pdb.set_trace() lgb_model.fit(X_train, y_train) # 使用新模型进行预测 y_pred = lgb_model.predict(X_test)改为svm模型
时间: 2023-06-23 15:08:39 浏览: 73
好的,那么你需要将导入的库改为sklearn中的SVM库,代码如下:
```python
from sklearn.svm import SVC
from sklearn.metrics import accuracy_score
from sklearn.model_selection import cross_val_score
from sklearn.model_selection import GridSearchCV
# 定义svm模型
svm_model = SVC()
# 设置参数空间
params = {
'kernel': ['linear', 'rbf', 'poly', 'sigmoid'],
'C': [0.1, 1, 10, 100],
'gamma': ['scale', 'auto', 0.1, 1, 10],
'decision_function_shape': ['ovo', 'ovr'],
'random_state': [2017]
}
# 输出最优参数
print('Best Parameter:', params)
# 使用GridSearchCV进行参数调优
svm_model = GridSearchCV(svm_model, params, cv=5)
# 使用训练集对新模型进行拟合
svm_model.fit(X_train, y_train)
# 使用新模型进行预测
y_pred = svm_model.predict(X_test)
```
注意,SVM模型的参数设置有很多,你需要根据你的数据集和问题场景来进行调整。在上面的代码中,我使用了GridSearchCV进行了参数调优,你可以根据需要进行修改。
相关推荐
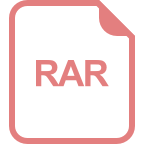
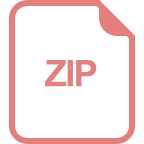
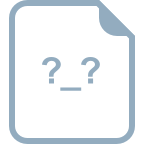














