python对红酒数据集分别用留出法、交叉验证法进行划分
时间: 2024-06-03 18:10:40 浏览: 58
对于红酒数据集的划分,可以采用留出法和交叉验证法。留出法是将数据集随机分为训练集和测试集两部分,一般将数据集的70%作为训练集,30%作为测试集。交叉验证法是将数据集划分为k个子集,然后进行k次训练和测试,每次将k-1个子集作为训练集,剩下的1个子集作为测试集,因此训练和测试均分布在了全数据集上。在Python中,我们可以利用sklearn库的train_test_split模块进行留出法划分,使用KFold模块进行交叉验证划分。
相关问题
python对红酒数据集分别用留出法、交叉验证法进行划分的代码怎么写
您好,以下是Python对红酒数据集分别用留出法、交叉验证法进行划分的示例代码。
留出法:
```
from sklearn.model_selection import train_test_split
from sklearn.datasets import load_wine
# 加载红酒数据集
wine = load_wine()
# 划分数据集,将30%作为测试集
X_train, X_test, y_train, y_test = train_test_split(wine.data, wine.target, test_size=0.3, random_state=0)
```
交叉验证法:
```
from sklearn.model_selection import KFold
from sklearn.datasets import load_wine
# 加载红酒数据集
wine = load_wine()
# 5折交叉验证
kf = KFold(n_splits=5, shuffle=True, random_state=0)
for train_index, test_index in kf.split(wine.data):
X_train, X_test = wine.data[train_index], wine.data[test_index]
y_train, y_test = wine.target[train_index], wine.target[test_index]
```
希望这些代码能够帮助到您!
python实现用SVM模型对sklearn数据库中的红酒数据集进行分类,通过五折交叉验证和网格搜索法确定最佳参数
在Python中,使用SVM(支持向量机)模型对sklearn库中的红酒数据集(Wine dataset)进行分类,可以按照以下步骤进行:
首先,确保已经安装了必要的库,包括`sklearn`, `numpy`, `pandas`, `matplotlib`以及`gridsearchcv`。可以使用`pip install`命令安装:
```bash
pip install scikit-learn numpy pandas matplotlib
```
接下来,我们进行以下操作:
1. 导入所需的模块:
```python
import numpy as np
import pandas as pd
from sklearn import datasets
from sklearn.model_selection import train_test_split, cross_val_score, GridSearchCV
from sklearn.preprocessing import StandardScaler
from sklearn.svm import SVC
from sklearn.metrics import classification_report, confusion_matrix
import matplotlib.pyplot as plt
```
2. 加载红酒数据集:
```python
wine = datasets.load_wine()
X = wine.data
y = wine.target
```
3. 数据预处理(标准化):
```python
scaler = StandardScaler()
X_scaled = scaler.fit_transform(X)
```
4. 划分训练集和测试集:
```python
X_train, X_test, y_train, y_test = train_test_split(X_scaled, y, test_size=0.2, random_state=42)
```
5. 定义GridSearchCV,设置参数网格和交叉验证:
```python
param_grid = {'C': [0.1, 1, 10, 100], 'kernel': ['linear', 'rbf'], 'gamma': ['scale', 'auto']}
grid_search = GridSearchCV(SVC(), param_grid, cv=5, scoring='accuracy')
```
6. 使用网格搜索找到最佳参数:
```python
grid_search.fit(X_train, y_train)
best_params = grid_search.best_params_
print("Best parameters found: ", best_params)
```
7. 预测并评估:
```python
y_pred = grid_search.predict(X_test)
print("Classification report:")
print(classification_report(y_test, y_pred))
conf_mat = confusion_matrix(y_test, y_pred)
plt.figure(figsize=(10, 7))
sns.heatmap(conf_mat, annot=True, cmap="Blues", fmt='d', xticklabels=wine.target_names, yticklabels=wine.target_names)
plt.xlabel('Predicted')
plt.ylabel('True')
plt.title('Confusion Matrix')
plt.show()
```
现在,你已经完成了SVM模型的训练、参数优化和评估过程。记得根据网格搜索的结果调整`SVC()`实例,然后使用`grid_search.predict()`进行预测。
阅读全文
相关推荐
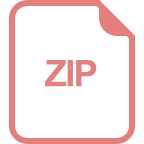


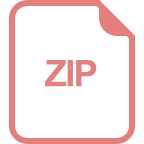
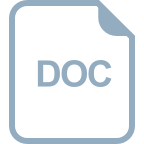





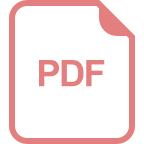
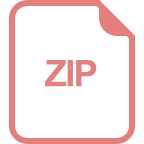


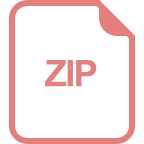