def SVG_process(self): # SVG方法 self.get_subword_vector() M = np.zeros((len(self.vocab), len(self.vocab))) df = pd.DataFrame(M, index=self.vocab, columns=self.vocab) print("Calculating the subword vector...") # 利用dataframe的字符串索引功能,使用子词向量进行计数,记录子词向量在词表中的出现频率 tbar = tqdm(total=len(self.subword_vector)) for i in self.subword_vector: try: df.at[i[0], i[1]] += 1 except: pass tbar.update(1) tbar.close() M = np.array(df) print(np.max(M)) svd = TruncatedSVD(n_components=3) self.result = svd.fit_transform(M) print(self.result.shape) def SGNS_process(self): print("Calculating the sim_sgns...") logging.basicConfig(level=logging.INFO, format='%(asctime)s - %(levelname)s - %(message)s') self.sim_sgns = [] vec_sgns = Word2Vec(LineSentence('dataset.txt'), vector_size=100, window=2, sg=1, hs=0, min_count=1, workers=multiprocessing.cpu_count()) tbar = tqdm(total=len(self.word_vector)) for word in self.word_vector: try: self.sim_sgns.append(vec_sgns.wv.similarity(word[0], word[1])) except: self.sim_sgns.append(0) tbar.update(1) tbar.close() # print(self.sim_sgns) f = open('sim_sgns', 'wb') pickle.dump(self.sim_sgns, f) f.close()
时间: 2024-02-15 17:28:50 浏览: 103
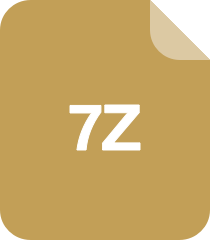
SVG_Editor_Source_Code.7z
这段代码中包含了两个方法,一个是SVG_process,另一个是SGNS_process。
SVG_process方法中,首先调用了get_subword_vector方法获取词表中的子词向量,然后使用一个二维数组M初始化一个DataFrame对象df,用于记录子词向量在词表中的出现频率。接着遍历子词向量列表,利用DataFrame的字符串索引功能将子词向量的出现次数记录在df中。最后使用TruncatedSVD对M进行奇异值分解,得到降维后的结果self.result。
SGNS_process方法中,首先使用Word2Vec读取文本数据集,构建词向量模型vec_sgns。然后遍历词向量列表,使用wv.similarity计算每个词向量之间的相似度,并将结果保存在self.sim_sgns列表中。最后将self.sim_sgns保存到文件中。
阅读全文
相关推荐
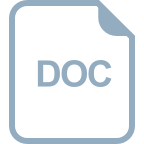
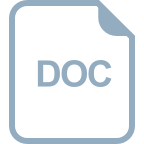
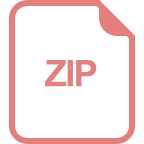
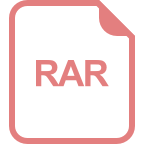
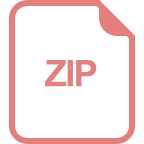
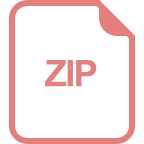
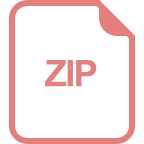
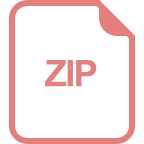
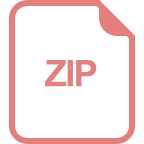
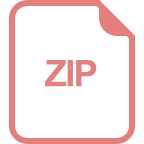
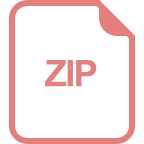
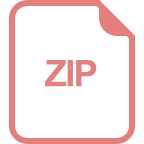
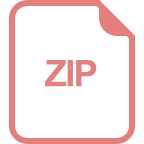
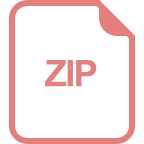
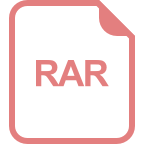
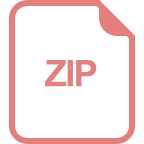