self.scale = scales if type(scales) is int else scales[0] self.sample_type = sample_type
时间: 2024-06-02 19:07:45 浏览: 121
这是一个 Python 代码中的语句,其中 self.scale 和 self.sample_type 分别赋值为 scales 和 sample_type。如果 scales 是一个整数,则 self.scale 被赋值为该整数;否则,self.scale 被赋值为 scales 列表的第一个元素。同样地,self.sample_type 被赋值为 sample_type。这段代码可能是在某个类的构造函数中出现的。需要根据上下文来确定其具体含义和作用。
相关问题
if p.scale_adaptation % Code from DSST scale_factor = 1; base_target_sz = target_sz; scale_sigma = sqrt(p.num_scales) * p.scale_sigma_factor; ss = (1:p.num_scales) - ceil(p.num_scales/2); ys = exp(-0.5 * (ss.^2) / scale_sigma^2); ysf = single(fft(ys)); if mod(p.num_scales,2) == 0 scale_window = single(hann(p.num_scales+1)); scale_window = scale_window(2:end); else scale_window = single(hann(p.num_scales)); end; ss = 1:p.num_scales; scale_factors = p.scale_step.^(ceil(p.num_scales/2) - ss); if p.scale_model_factor^2 * prod(p.norm_target_sz) > p.scale_model_max_area p.scale_model_factor = sqrt(p.scale_model_max_area/prod(p.norm_target_sz)); end scale_model_sz = floor(p.norm_target_sz * p.scale_model_factor); % find maximum and minimum scales min_scale_factor = p.scale_step ^ ceil(log(max(5 ./ bg_area)) / log(p.scale_step)); max_scale_factor = p.scale_step ^ floor(log(min([size(im,1) size(im,2)] ./ target_sz)) / log(p.scale_step)); end
这段代码实现的是目标跟踪算法中的尺度自适应部分。首先判断是否需要进行尺度自适应,然后计算尺度变换因子scale_factor、目标大小base_target_sz和尺度响应图的标准差scale_sigma。接着生成一组尺度因子scale_factors,用于对目标进行尺度变换。在此基础上,计算尺度响应图的权重系数ys,进行傅里叶变换,最后使用汉宁窗口函数来平滑尺度响应图。在计算过程中还进行了一些参数的限制和约束,例如限制尺度模型的面积不超过最大值、限制尺度变换因子的最大和最小值等。
class PrototypicalCalibrationBlock: def __init__(self, cfg): super().__init__() self.cfg = cfg self.device = torch.device(cfg.MODEL.DEVICE) self.alpha = self.cfg.TEST.PCB_ALPHA self.imagenet_model = self.build_model() self.dataloader = build_detection_test_loader(self.cfg, self.cfg.DATASETS.TRAIN[0]) self.roi_pooler = ROIPooler(output_size=(1, 1), scales=(1 / 32,), sampling_ratio=(0), pooler_type="ROIAlignV2") self.prototypes = self.build_prototypes() self.exclude_cls = self.clsid_filter() def build_model(self): logger.info("Loading ImageNet Pre-train Model from {}".format(self.cfg.TEST.PCB_MODELPATH)) if self.cfg.TEST.PCB_MODELTYPE == 'resnet': imagenet_model = resnet101() else: raise NotImplementedError state_dict = torch.load(self.cfg.TEST.PCB_MODELPATH) imagenet_model.load_state_dict(state_dict) imagenet_model = imagenet_model.to(self.device) imagenet_model.eval() return imagenet_model def build_prototypes(self): all_features, all_labels = [], [] for index in range(len(self.dataloader.dataset)): inputs = [self.dataloader.dataset[index]] assert len(inputs) == 1 # load support images and gt-boxes img = cv2.imread(inputs[0]['file_name']) # BGR img_h, img_w = img.shape[0], img.shape[1] ratio = img_h / inputs[0]['instances'].image_size[0] inputs[0]['instances'].gt_boxes.tensor = inputs[0]['instances'].gt_boxes.tensor * ratio boxes = [x["instances"].gt_boxes.to(self.device) for x in inputs] # extract roi features features = self.extract_roi_features(img, boxes) all_features.append(features.cpu().data) gt_classes = [x['instances'].gt_classes for x in inputs] all_labels.append(gt_classes[0].cpu().data)
这段代码是一个名为PrototypicalCalibrationBlock的类的定义,它包含了一些方法和属性。__init__方法接受一个cfg参数,用来初始化一些属性。其中包括设备类型、alpha值、预训练模型、数据加载器、RoI池化器和类别原型等。build_model方法用于加载ImageNet预训练模型,支持resnet101模型。build_prototypes方法用于提取RoI特征和类别标签,并将其存储为特征向量和类别原型。这个类的作用是在目标检测任务上进行模型校准。
阅读全文
相关推荐
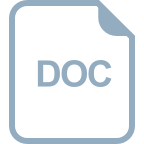
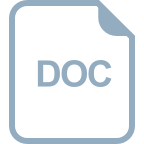
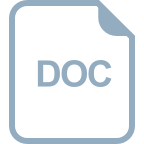












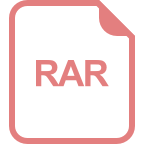