def forward(self, x): x = self.conv(x) x = self.layer1(x) x = self.layer2(x) x = self.layer3(x) x = self.layer4(x) x = self.avgpool(x) x = x.view(x.size(0),-1) # B x 128 if self.reid: x = x.div(x.norm(p=2,dim=1,keepdim=True)) return x # classifier x = self.classifier(x) return x
时间: 2023-09-20 22:08:20 浏览: 192
这是一个 PyTorch 模型中的 forward 函数,用于前向传播计算。该模型包含了卷积层、ResNet 的若干层、平均池化层和全连接层分类器。
具体来说,该函数的输入是 x,表示输入的数据。在 forward 函数中,x 首先经过卷积层 self.conv,得到一些特征图。然后,特征图通过 ResNet 的若干层 self.layer1、self.layer2、self.layer3、self.layer4,不断提取和提高特征层次,最终得到更加抽象和高层次的特征表示。接着,特征图通过平均池化层 self.avgpool 进行降维,得到一个 B x C x 1 x 1 的张量(B 表示 batch size,C 表示特征通道数)。
如果模型是用于 ReID 任务,接下来的代码将对特征向量进行归一化处理,即将特征向量除以其 L2 范数,以实现更好的特征表达。最后,如果模型是用于分类任务,特征向量将被送入全连接层 self.classifier 进行分类。函数最终返回输出的结果。
相关问题
class EnhancedResidual(nn.Module): def init(self,in_c,out_c,fm_sz,net_type = 'ta'): super(EnhancedResidual,self).init() self.net_type = net_type self.conv1 = nn.Sequential( nn.Conv2d(in_channels = in_c,out_channels = in_c,kernel_size = 3,padding = 1), nn.BatchNorm2d(in_c), nn.ReLU(), ) self.conv2 = nn.Sequential( nn.Conv2d(in_channels = in_c,out_channels = out_c,kernel_size = 3,padding = 1), nn.BatchNorm2d(out_c), nn.ReLU(), ) self.botneck = nn.Conv2d(in_channels = in_c,out_channels = out_c,kernel_size = 1) self.pool = nn.MaxPool2d(kernel_size = 2,stride = 2) if net_type == 'ta': self.spa = SpatialAttention() self.ca = ChannelAttention(in_planes = in_c,ratio = in_c) self.sa = MultiHeadSelfAttention(in_c = in_c,out_c = in_c // 4,head_n = 4,fm_sz = fm_sz) elif net_type == 'sa': self.sa = MultiHeadSelfAttention(in_c = in_c,out_c = out_c // 4,head_n = 4,fm_sz = fm_sz) elif net_type == 'cbam': self.spa = SpatialAttention() self.ca = ChannelAttention(in_planes = in_c,ratio = in_c) def forward(self,x): x0 = self.botneck(x) x = self.conv1(x) if self.net_type == 'sa': x = self.sa(x) #x = self.conv2(x) elif self.net_type == 'cbam': x = self.ca(x) * x x = self.spa(x) * x x = self.conv2(x) elif self.net_type == 'ta': x = self.ca(x) * x x = self.spa(x) * x x = self.sa(x) x = self.conv2(x) x = x + x0 x = self.pool(x) return x 改写为tensorflow形式
import tensorflow as tf
class EnhancedResidual(tf.keras.layers.Layer):
def __init__(self, in_c, out_c, fm_sz, net_type='ta', **kwargs):
super(EnhancedResidual, self).__init__(**kwargs)
self.net_type = net_type
self.conv1 = tf.keras.Sequential([
tf.keras.layers.Conv2D(filters=in_c, kernel_size=3, padding='same'),
tf.keras.layers.BatchNormalization(),
tf.keras.layers.ReLU()
])
self.conv2 = tf.keras.Sequential([
tf.keras.layers.Conv2D(filters=out_c, kernel_size=3, padding='same'),
tf.keras.layers.BatchNormalization(),
tf.keras.layers.ReLU(),
])
self.botneck = tf.keras.layers.Conv2D(filters=out_c, kernel_size=1)
self.pool = tf.keras.layers.MaxPool2D(pool_size=2, strides=2)
if net_type == 'ta':
self.spa = SpatialAttention()
self.ca = ChannelAttention(in_planes=in_c, ratio=in_c)
self.sa = MultiHeadSelfAttention(in_c=in_c, out_c=in_c // 4, head_n=4, fm_sz=fm_sz)
elif net_type == 'sa':
self.sa = MultiHeadSelfAttention(in_c=in_c, out_c=out_c // 4, head_n=4, fm_sz=fm_sz)
elif net_type == 'cbam':
self.spa = SpatialAttention()
self.ca = ChannelAttention(in_planes=in_c, ratio=in_c)
def call(self, x):
x0 = self.botneck(x)
x = self.conv1(x)
if self.net_type == 'sa':
x = self.sa(x)
# x = self.conv2(x)
elif self.net_type == 'cbam':
x = self.ca(x) * x
x = self.spa(x) * x
x = self.conv2(x)
elif self.net_type == 'ta':
x = self.ca(x) * x
x = self.spa(x) * x
x = self.sa(x)
x = self.conv2(x)
x = x + x0
x = self.pool(x)
return x
def init(self, input_nc=3, ndf=64, n_layers=3, norm_layer=nn.BatchNorm2d, use_sigmoid=False, use_parallel=True): super(NLayerDiscriminator, self).init() self.use_parallel = use_parallel if type(norm_layer) == functools.partial: use_bias = norm_layer.func == nn.InstanceNorm2d else: use_bias = norm_layer == nn.InstanceNorm2d self.conv1 = nn.Conv2d(input_nc, ndf, kernel_size=3, padding=1) self.conv_offset1 = nn.Conv2d(512, 18, kernel_size=3, stride=1, padding=1) init_offset1 = torch.Tensor(np.zeros([18, 512, 3, 3])) self.conv_offset1.weight = torch.nn.Parameter(init_offset1) # 初始化为0 self.conv_mask1 = nn.Conv2d(512, 9, kernel_size=3, stride=1, padding=1) init_mask1 = torch.Tensor(np.zeros([9, 512, 3, 3]) + np.array([0.5])) self.conv_mask1.weight = torch.nn.Parameter(init_mask1) # 初始化为0.5 def forward(self, input): input = self.conv1(input) offset1 = self.conv_offset1(input) mask1 = torch.sigmoid(self.conv_mask1(input)) sequence1 = [ torchvision.ops.deform_conv2d(input=input, offset=offset1, weight=self.conv1.weight, mask=mask1, padding=(1, 1)) ] sequence2 = sequence1 + self.sequence2 self.model = nn.Sequential(*sequence2) nn.LeakyReLU(0.2, True) return self.model(input),上述代码出现问题:RuntimeError: Given groups=1, weight of size [18, 512, 3, 3], expected input[1, 64, 512, 512] to have 512 channels, but got 64 channels instead,如何修改
这个错误是由于conv_offset1和conv_mask1的输入通道数与conv1的输出通道数不匹配所引起的。你可以尝试修改conv_offset1和conv_mask1的输入通道数以匹配conv1的输出通道数,或者将conv1的输出通道数修改为匹配conv_offset1和conv_mask1的输入通道数。你可以使用print语句检查conv1的输出通道数和conv_offset1、conv_mask1的输入通道数是否匹配。
根据报错信息,可以看到输入的张量input的shape为[1, 64, 512, 512],而conv_offset1和conv_mask1的输入通道数为512,但conv1的输出通道数为ndf,即64。因此,你需要将conv_offset1和conv_mask1的输入通道数修改为ndf,即64。修改后的代码如下:
```
def __init__(self, input_nc=3, ndf=64, n_layers=3, norm_layer=nn.BatchNorm2d, use_sigmoid=False, use_parallel=True):
super(NLayerDiscriminator, self).__init__()
self.use_parallel = use_parallel
if type(norm_layer) == functools.partial:
use_bias = norm_layer.func == nn.InstanceNorm2d
else:
use_bias = norm_layer == nn.InstanceNorm2d
self.conv1 = nn.Conv2d(input_nc, ndf, kernel_size=3, padding=1)
self.conv_offset1 = nn.Conv2d(ndf, 18, kernel_size=3, stride=1, padding=1)
init_offset1 = torch.Tensor(np.zeros([18, ndf, 3, 3]))
self.conv_offset1.weight = torch.nn.Parameter(init_offset1) # 初始化为0
self.conv_mask1 = nn.Conv2d(ndf, 9, kernel_size=3, stride=1, padding=1)
init_mask1 = torch.Tensor(np.zeros([9, ndf, 3, 3]) + np.array([0.5]))
self.conv_mask1.weight = torch.nn.Parameter(init_mask1) # 初始化为0.5
def forward(self, input):
input = self.conv1(input)
offset1 = self.conv_offset1(input)
mask1 = torch.sigmoid(self.conv_mask1(input))
sequence1 = [ torchvision.ops.deform_conv2d(input=input, offset=offset1, weight=self.conv1.weight, mask=mask1, padding=(1, 1)) ]
sequence2 = sequence1 + self.sequence2
self.model = nn.Sequential(*sequence2)
nn.LeakyReLU(0.2, True)
return self.model(input)
```
阅读全文
相关推荐
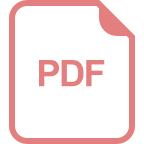
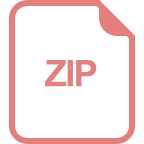
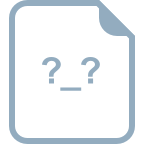














