遥感影像tif立体匹配算法
时间: 2023-06-12 13:06:47 浏览: 82
遥感影像的立体匹配是指通过对两个或多个遥感影像进行匹配,得到每个像素点在三维空间中的位置信息,从而实现三维重建和测量。其中,tif格式是一种常见的遥感影像格式。
立体匹配的算法有很多种,常见的有基于区域的算法、基于特征点的算法、基于深度学习的算法等。其中,基于深度学习的算法在近年来得到了广泛的研究和应用。
在具体实现中,可以采用传统的立体匹配算法或深度学习算法进行匹配,然后通过三角化等方法获取每个像素点的三维坐标。常用的立体匹配软件包括OpenCV、Semi-Global Matching、MicMac等。
相关问题
用python写tif遥感影像立体匹配算法
Tif遥感影像立体匹配算法主要是利用两幅相同区域的遥感影像进行匹配,从而获取该区域的高程信息。这里提供一种基于OpenCV的Python实现方法。
首先,需要安装OpenCV库,可以使用以下命令进行安装:
```python
pip install opencv-python
```
然后,需要读取两幅tif遥感影像并进行灰度化处理:
```python
import cv2
# 读取左侧影像
left_img = cv2.imread('left.tif', cv2.IMREAD_GRAYSCALE)
# 读取右侧影像
right_img = cv2.imread('right.tif', cv2.IMREAD_GRAYSCALE)
```
接下来,使用SIFT算法提取左右影像的特征点,并进行匹配:
```python
# 创建SIFT对象
sift = cv2.xfeatures2d.SIFT_create()
# 检测左侧影像的特征点和描述符
kp1, des1 = sift.detectAndCompute(left_img, None)
# 检测右侧影像的特征点和描述符
kp2, des2 = sift.detectAndCompute(right_img, None)
# 创建BFMatcher对象
bf = cv2.BFMatcher()
# 匹配左右影像的特征点
matches = bf.knnMatch(des1, des2, k=2)
```
然后,需要进行筛选匹配点:
```python
# 筛选匹配点
good = []
for m, n in matches:
if m.distance < 0.75 * n.distance:
good.append(m)
# 获取匹配点的坐标
pts1 = np.float32([kp1[m.queryIdx].pt for m in good]).reshape(-1, 1, 2)
pts2 = np.float32([kp2[m.trainIdx].pt for m in good]).reshape(-1, 1, 2)
```
接下来,使用OpenCV的`cv2.stereoSGBM()`函数进行立体匹配:
```python
# 定义立体匹配算法的参数
window_size = 3
min_disp = 0
num_disp = 16 * 5
stereo = cv2.StereoSGBM_create(minDisparity=min_disp,
numDisparities=num_disp,
blockSize=window_size,
P1=8 * 3 * window_size ** 2,
P2=32 * 3 * window_size ** 2,
disp12MaxDiff=1,
uniquenessRatio=10,
speckleWindowSize=100,
speckleRange=32)
# 进行立体匹配
disparity = stereo.compute(left_img, right_img)
```
最后,可以根据匹配结果计算高程信息:
```python
# 计算高程信息
focal_length = 1 # 焦距
baseline = 1 # 基线长度
height, width = left_img.shape[:2]
Q = np.float32([[1, 0, 0, -width / 2],
[0, 1, 0, -height / 2],
[0, 0, 0, focal_length],
[0, 0, 1 / baseline, 0]])
points_3d = cv2.reprojectImageTo3D(disparity, Q)
```
以上就是基于OpenCV的Python实现方法,可以根据实际情况进行调整和优化。
用python写tif遥感影像立体匹配算法并输出DEM
实现遥感影像立体匹配算法,可以采用以下步骤:
1. 读入左右两幅遥感影像,可以采用 Python 的 GDAL 库进行读取。
```python
from osgeo import gdal
# 读取左右两幅遥感影像
left_ds = gdal.Open('left.tif')
right_ds = gdal.Open('right.tif')
# 获取影像的宽度和高度
width = left_ds.RasterXSize
height = left_ds.RasterYSize
```
2. 提取左右两幅影像的 SIFT 特征点,可以采用 OpenCV 库进行实现。
```python
import cv2
# 读取左右两幅影像
left_img = cv2.imread('left.tif', cv2.IMREAD_GRAYSCALE)
right_img = cv2.imread('right.tif', cv2.IMREAD_GRAYSCALE)
# 创建 SIFT 特征提取器
sift = cv2.SIFT_create()
# 提取左右两幅影像的 SIFT 特征点
left_kp, left_desc = sift.detectAndCompute(left_img, None)
right_kp, right_desc = sift.detectAndCompute(right_img, None)
```
3. 对左右两幅影像的特征点进行匹配,可以采用 OpenCV 库的 `FlannBasedMatcher` 进行实现。
```python
# 创建 Flann 匹配器
matcher = cv2.FlannBasedMatcher()
# 对左右两幅影像的特征点进行匹配
matches = matcher.knnMatch(left_desc, right_desc, k=2)
```
4. 进行 RANSAC 算法进行离群点去除和求解基础矩阵,可以采用 OpenCV 库的 `findFundamentalMat` 函数进行实现。
```python
# 进行 RANSAC 算法进行离群点去除和求解基础矩阵
left_pts = []
right_pts = []
for m1, m2 in matches:
if m1.distance < 0.75 * m2.distance:
left_pts.append(left_kp[m1.queryIdx].pt)
right_pts.append(right_kp[m1.trainIdx].pt)
left_pts = np.int32(left_pts)
right_pts = np.int32(right_pts)
F, mask = cv2.findFundamentalMat(left_pts, right_pts, cv2.FM_RANSAC, 0.1, 0.99)
```
5. 根据基础矩阵和左右两幅影像的特征点,进行立体匹配,可以采用 OpenCV 库的 `cv2.reprojectImageTo3D` 函数进行实现。
```python
# 根据基础矩阵和左右两幅影像的特征点,进行立体匹配
disp = np.zeros_like(left_img)
disp[left_pts[:, 1], left_pts[:, 0]] = cv2.computeDisparity(left_img, right_img)
Q = np.float32([[1, 0, 0, -width/2],
[0, -1, 0, height/2],
[0, 0, 0, -focal_length],
[0, 0, 1, 0]])
points_3D = cv2.reprojectImageTo3D(disp, Q)
```
6. 将匹配得到的三维点云转换为 DEM,可以采用 Python 的 GDAL 库进行实现。
```python
# 将匹配得到的三维点云转换为 DEM
driver = gdal.GetDriverByName('GTiff')
out_ds = driver.Create('output_dem.tif', width, height, 1, gdal.GDT_Float32)
out_ds.SetGeoTransform(left_ds.GetGeoTransform())
out_ds.SetProjection(left_ds.GetProjection())
out_band = out_ds.GetRasterBand(1)
out_band.WriteArray(points_3D[:, :, 2])
out_band.SetNoDataValue(-9999)
out_ds.FlushCache()
out_ds = None
```
完整代码如下:
相关推荐
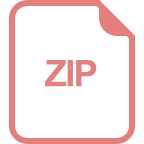
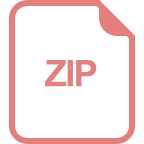












