首页python中,basic_array[i,j]^k是啥意思
python中,basic_array[i,j]^k是啥意思
时间: 2024-05-21 12:18:31 浏览: 109
在Python中,`basic_array[i,j]^k`表示取`basic_array`数组中第`i`行第`j`列的元素,然后对它进行k次按位异或操作(`^`符号表示按位异或)。具体来说,按位异或操作是将两个二进制数对应位上的数进行异或运算,即若两个数相应位上的数字相同,则该位结果为0,否则为1。因此,`basic_array[i,j]^k`的结果是一个整数值。
阅读全文
CSDN会员
开通CSDN年卡参与万元壕礼抽奖
最新推荐
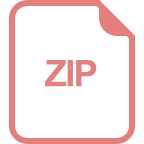
Java毕业设计项目:校园二手交易网站开发指南
资源摘要信息:"Java是一种高性能、跨平台的面向对象编程语言,由Sun Microsystems(现为Oracle Corporation)的James Gosling等人在1995年推出。其设计理念是为了实现简单性、健壮性、可移植性、多线程以及动态性。Java的核心优势包括其跨平台特性,即“一次编写,到处运行”(Write Once, Run Anywhere),这得益于Java虚拟机(JVM)的存在,它提供了一个中介,使得Java程序能够在任何安装了相应JVM的设备上运行,无论操作系统如何。
Java是一种面向对象的编程语言,这意味着它支持面向对象编程(OOP)的三大特性:封装、继承和多态。封装使得代码模块化,提高了安全性;继承允许代码复用,简化了代码的复杂性;多态则增强了代码的灵活性和扩展性。
Java还具有内置的多线程支持能力,允许程序同时处理多个任务,这对于构建服务器端应用程序、网络应用程序等需要高并发处理能力的应用程序尤为重要。
自动内存管理,特别是垃圾回收机制,是Java的另一大特性。它自动回收不再使用的对象所占用的内存资源,这样程序员就无需手动管理内存,从而减轻了编程的负担,并减少了因内存泄漏而导致的错误和性能问题。
Java广泛应用于企业级应用开发、移动应用开发(尤其是Android平台)、大型系统开发等领域,并且有大量的开源库和框架支持,例如Spring、Hibernate、Struts等,这些都极大地提高了Java开发的效率和质量。
标签中提到的Java、毕业设计、课程设计和开发,意味着文件“毕业设计---社区(校园)二手交易网站.zip”中的内容可能涉及到Java语言的编程实践,可能是针对学生的课程设计或毕业设计项目,而开发则指出了这些内容的具体活动。
在文件名称列表中,“SJT-code”可能是指该压缩包中包含的是一个特定的项目代码,即社区(校园)二手交易网站的源代码。这类网站通常需要实现用户注册、登录、商品发布、浏览、交易、评价等功能,并且需要后端服务器支持,如数据库连接和事务处理等。考虑到Java的特性,网站的开发可能使用了Java Web技术栈,如Servlet、JSP、Spring Boot等,以及数据库技术,如MySQL或MongoDB等。"

管理建模和仿真的文件
管理Boualem Benatallah引用此版本:布阿利姆·贝纳塔拉。管理建模和仿真。约瑟夫-傅立叶大学-格勒诺布尔第一大学,1996年。法语。NNT:电话:00345357HAL ID:电话:00345357https://theses.hal.science/tel-003453572008年12月9日提交HAL是一个多学科的开放存取档案馆,用于存放和传播科学研究论文,无论它们是否被公开。论文可以来自法国或国外的教学和研究机构,也可以来自公共或私人研究中心。L’archive ouverte pluridisciplinaire
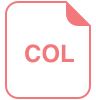
【MVC标准化:肌电信号处理的终极指南】:提升数据质量的10大关键步骤与工具
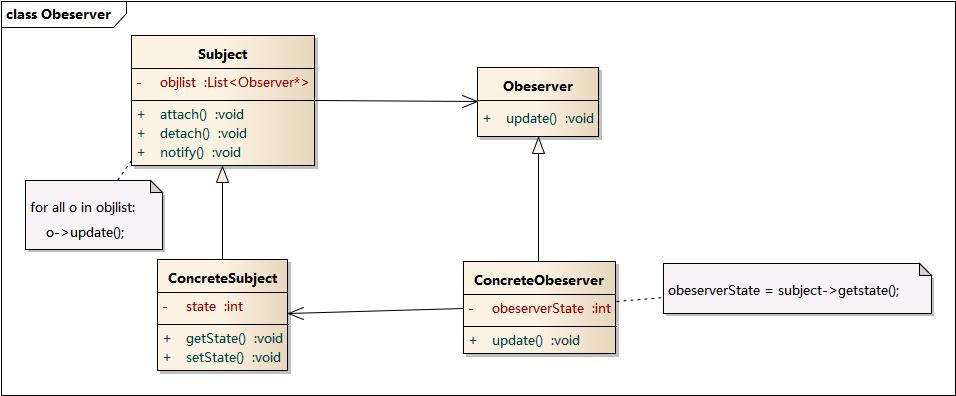
# 摘要
MVC标准化是肌电信号处理中确保数据质量的重要步骤,它对于提高测量结果的准确性和可重复性至关重要。本文首先介绍肌电信号的生理学原理和MVC标准化理论,阐述了数据质量的重要性及影响因素。随后,文章深入探讨了肌电信号预处理的各个环节,包括噪声识别与消除、信号放大与滤波技术、以及基线漂移的校正方法。在提升数据质量的关键步骤部分,本文详细描述了信号特征提取、MVC标准化的实施与评估,并讨论了数据质量评估与优化工具。最后,本文通过实验设计和案例分析,展示了MVC标准化在实践应用中的具

能否提供一个在R语言中执行Framingham数据集判别分析的详细和完整的代码示例?
当然可以。在R语言中,Framingham数据集是一个用于心血管疾病研究的经典数据集。以下是使用`ggfortify`包结合` factoextra`包进行判别分析的一个基本步骤:
首先,你需要安装所需的库,如果尚未安装,可以使用以下命令:
```r
install.packages(c("ggfortify", "factoextra"))
```
然后加载所需的数据集并做预处理。Framingham数据集通常存储在`MASS`包中,你可以通过下面的代码加载:
```r
library(MASS)
data(Framingham)
```
接下来,我们假设你已经对数据进行了适当的清洗和转换
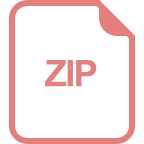
Blaseball Plus插件开发与构建教程
资源摘要信息:"Blaseball Plus"
Blaseball Plus是一个与游戏Blaseball相关的扩展项目,该项目提供了一系列扩展和改进功能,以增强Blaseball游戏体验。在这个项目中,JavaScript被用作主要开发语言,通过在package.json文件中定义的脚本来完成构建任务。项目说明中提到了开发环境的要求,即在20.09版本上进行开发,并且提供了一个flake.nix文件来复制确切的构建环境。虽然Nix薄片是一项处于工作状态(WIP)的功能且尚未完全记录,但可能需要用户自行安装系统依赖项,其中列出了Node.js和纱(Yarn)的特定版本。
### 知识点详细说明:
#### 1. Blaseball游戏:
Blaseball是一个虚构的棒球游戏,它在互联网社区中流行,其特点是独特的规则、随机事件和社区参与的元素。
#### 2. 扩展开发:
Blaseball Plus是一个扩展,它可能是为在浏览器中运行的Blaseball游戏提供额外功能和改进的软件。扩展开发通常涉及编写额外的代码来增强现有软件的功能。
#### 3. JavaScript编程语言:
JavaScript是一种高级的、解释执行的编程语言,被广泛用于网页和Web应用的客户端脚本编写,是开发Web扩展的关键技术之一。
#### 4. package.json文件:
这是Node.js项目的核心配置文件,用于声明项目的各种配置选项,包括项目名称、版本、依赖关系以及脚本命令等。
#### 5.构建脚本:
描述中提到的脚本,如`build:dev`、`build:prod:unsigned`和`build:prod:signed`,这些脚本用于自动化构建过程,可能包括编译、打包、签名等步骤。`yarn run`命令用于执行这些脚本。
#### 6. yarn包管理器:
Yarn是一个快速、可靠和安全的依赖项管理工具,类似于npm(Node.js的包管理器)。它允许开发者和项目管理依赖项,通过简单的命令行界面可以轻松地安装和更新包。
#### 7. Node.js版本管理:
项目要求Node.js的具体版本,这里是14.9.0版本。管理特定的Node.js版本是重要的,因为在不同版本间可能会存在API变化或其他不兼容问题,这可能会影响扩展的构建和运行。
#### 8. 系统依赖项的安装:
文档提到可能需要用户手动安装系统依赖项,这在使用Nix薄片时尤其常见。Nix薄片(Nix flakes)是一个实验性的Nix特性,用于提供可复现的开发环境和构建设置。
#### 9. Web扩展的工件放置:
构建后的工件放置在`addon/web-ext-artifacts/`目录中,表明这可能是一个基于WebExtension的扩展项目。WebExtension是一种跨浏览器的扩展API,用于创建浏览器扩展。
#### 10. 扩展部署:
描述中提到了两种不同类型的构建版本:开发版(dev)和生产版(prod),其中生产版又分为未签名(unsigned)和已签名(signed)版本。这些不同的构建版本用于不同阶段的开发和发布。
通过这份文档,我们能够了解到Blaseball Plus项目的开发环境配置、构建脚本的使用、依赖管理工具的运用以及Web扩展的基本概念和部署流程。这些知识点对于理解JavaScript项目开发和扩展构建具有重要意义。

"互动学习:行动中的多样性与论文攻读经历"
多样性她- 事实上SCI NCES你的时间表ECOLEDO C Tora SC和NCESPOUR l’Ingén学习互动,互动学习以行动为中心的强化学习学会互动,互动学习,以行动为中心的强化学习计算机科学博士论文于2021年9月28日在Villeneuve d'Asq公开支持马修·瑟林评审团主席法布里斯·勒菲弗尔阿维尼翁大学教授论文指导奥利维尔·皮耶昆谷歌研究教授:智囊团论文联合主任菲利普·普雷教授,大学。里尔/CRISTAL/因里亚报告员奥利维耶·西格德索邦大学报告员卢多维奇·德诺耶教授,Facebook /索邦大学审查员越南圣迈IMT Atlantic高级讲师邀请弗洛里安·斯特鲁布博士,Deepmind对于那些及时看到自己错误的人...3谢谢你首先,我要感谢我的两位博士生导师Olivier和Philippe。奥利维尔,"站在巨人的肩膀上"这句话对你来说完全有意义了。从科学上讲,你知道在这篇论文的(许多)错误中,你是我可以依
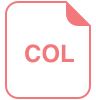
【天线性能提升密籍】:深入探究均匀线阵方向图设计原则及案例分析
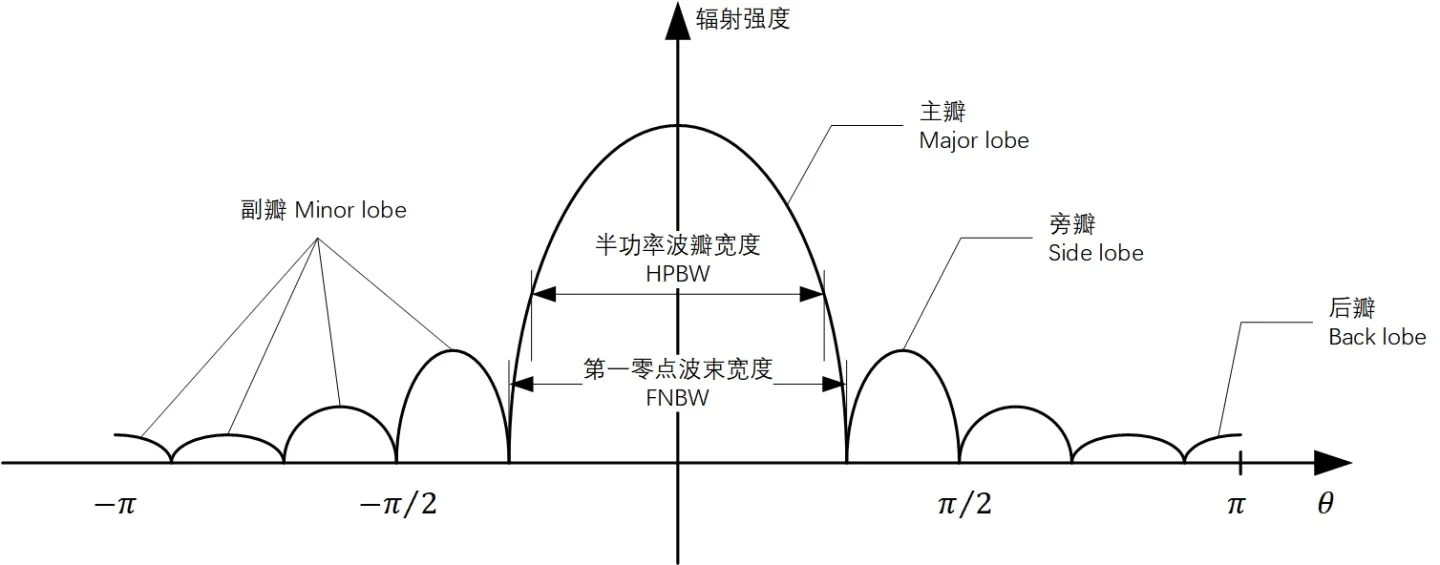
# 摘要
本文深入探讨了均匀线阵天线的基础理论及其方向图设计,旨在提升天线系统的性能和应用效能。文章首先介绍了均匀线阵及方向图的基本概念,并阐述了方向图设计的理论基础,包括波束形成与主瓣及副瓣特性的控制。随后,论文通过设计软件工具的应用和实际天线系统调试方法,展示了方向图设计的实践技巧。文中还包含了一系列案例分析,以实证研究验证理论,并探讨了均匀线阵性能

C#怎么把图片存入名为当前日期的文件夹里
在C#中,你可以通过`System.IO`命名空间下的`Directory`和`File`类来创建并存储图片到包含当前日期的文件夹里。以下是一个简单的示例:
```csharp
using System;
using System.IO;
public void SaveImageToTodayFolder(string imagePath, string imageName)
{
// 获取当前日期
DateTime currentDate = DateTime.Now;
string folderPath = Path.Combine(Environment.C
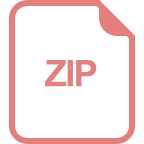
Deno Express:模仿Node.js Express的Deno Web服务器解决方案
资源摘要信息:"deno-express:该项目的灵感来自https"
知识点:
1. Deno 介绍:Deno 是一个简单、现代且安全的JavaScript和TypeScript运行时,由Node.js的原作者Ryan Dahl开发。它内置了诸如TypeScript支持、依赖模块的自动加载等功能。Deno的出现是为了解决Node.js存在的一些问题,比如全局状态污染和包管理等。
2. Express.js 概念:Express.js 是一个基于Node.js平台的极简、灵活的web应用开发框架。它提供了一系列强大的功能,用于开发单页、多页和混合web应用。Express.js的亮点在于其路由系统,对中间件的使用,以及对视图引擎的支持。
3. deno-express 项目:该项目以Node.js的Express框架为灵感,为Deno提供了一套类似于Express的Web服务器搭建方式。使用deno-express可以让开发者用熟悉的Express API在Deno环境中快速构建Web应用。
4. TypeScript 使用:TypeScript 是 JavaScript 的一个超集,添加了类型系统和对ES6+的新特性的支持。它最终会被编译成纯JavaScript代码,以便在浏览器和Node.js等JavaScript环境中运行。在deno-express项目中,通过TypeScript编写代码,不仅可以享受到静态类型检查的好处,还可以利用TypeScript的强类型系统来构建更稳定、易于维护的代码。
5. 代码示例解析:在描述中提供了一个简短的代码示例,示范了如何使用deno-express构建一个简单的web server。
- `import * as expressive from "https://raw.githubusercontent.com/NMathar/deno-express/master/mod.ts";` 这行代码通过网络导入了deno-express库的核心模块。
- `const port = 3000;` 定义了一个端口号,即web服务器将监听的端口。
- `const app = new expressive.App();` 创建了一个Express-like的App实例。
- `app.use(expressive.simpleLog());` 使用了一个简单的日志中间件,这可能会记录请求和响应的信息。
- `app.use(expressive.static_("./public"));` 使用了静态文件服务中间件,指定 "./public" 作为静态文件目录,使得该目录下的文件可以被Web服务访问。
- `app.use(expressive.bodyParser.json());` 使用了body-parser中间件,它能解析请求体中的JSON格式数据,使得在后续的请求处理中可以方便地获取这些数据。
6. Deno 与 Node.js 的对比:Deno与Node.js在设计哲学和实现上有明显差异。Deno不使用npm作为包管理器,而是通过URL导入模块。它也具备内置的TLS和网络测试工具,以及自动的依赖项管理,这都是Node.js需要外部模块来实现的功能。
7. 代码示例中的未显示部分:描述中仅展示了server.ts文件的部分内容,根据标准的Express应用结构,可能还会包括定义路由、设置视图引擎、错误处理中间件等。
8. 模块和库的使用:在deno-express项目中,开发者会接触到如何在Deno环境下使用外部模块。在JavaScript和TypeScript社区中,通过URL直接导入模块是一个新颖的方法,它使得依赖关系变得清晰,并且有助于构建安全、无包管理器污染的应用。
9. 对于TypeScript的依赖:由于deno-express项目的代码示例是用TypeScript编写的,所以它展示了TypeScript在Deno项目中如何使用。Deno对TypeScript的支持是原生的,无需额外编译器,直接运行即可。
10. Web服务器搭建实践:通过这个项目,开发者可以学习如何在Deno中搭建和管理Web服务器,包括如何处理路由、如何对请求和响应进行中间件处理等Web开发基础知识点。
通过对以上知识点的了解,可以对deno-express项目有一个全面的认识。该项目不仅为Deno提供了类似Express.js的Web开发体验,还展示了如何利用TypeScript来构建现代化、高性能的Web应用。

关系数据表示学习
关系数据卢多维奇·多斯桑托斯引用此版本:卢多维奇·多斯桑托斯。关系数据的表示学习机器学习[cs.LG]。皮埃尔和玛丽·居里大学-巴黎第六大学,2017年。英语。NNT:2017PA066480。电话:01803188HAL ID:电话:01803188https://theses.hal.science/tel-01803188提交日期:2018年HAL是一个多学科的开放存取档案馆,用于存放和传播科学研究论文,无论它们是否被公开。论文可以来自法国或国外的教学和研究机构,也可以来自公共或私人研究中心。L’archive ouverte pluridisciplinaireUNIVERSITY PIERRE和 MARIE CURIE计算机科学、电信和电子学博士学院(巴黎)巴黎6号计算机科学实验室D八角形T HESIS关系数据表示学习作者:Ludovic DOS SAntos主管:Patrick GALLINARI联合主管:本杰明·P·伊沃瓦斯基为满足计算机科学博士学位的要求而提交的论文评审团成员:先生蒂埃里·A·退休记者先生尤尼斯·B·恩