frequent_itemsets = [frequent_items] k = 2 while frequent_itemsets[-1]: candidate_itemsets = generate_candidates(frequent_itemsets[-1], k) itemset_support = calculate_support(candidate_itemsets) frequent_itemsets.append(set(itemset for itemset, support in itemset_support.items() if support >= min_support)) k += 1
时间: 2024-04-04 18:30:28 浏览: 13
这段代码实现了 Apriori 算法的核心部分,用于挖掘频繁项集。具体来说,代码的执行流程如下:
1. 初始化 frequent_itemsets 列表,将初始频繁项集 frequent_items 添加到列表中;
2. 设置 k = 2,开始循环;
3. 调用 generate_candidates 函数,根据 frequent_itemsets[-1] 生成候选项集 candidate_itemsets;
4. 调用 calculate_support 函数,计算候选项集的支持度 itemset_support;
5. 将支持度不低于 min_support 的频繁项集添加到 frequent_itemsets 列表中;
6. k += 1,继续循环,直到 frequent_itemsets[-1] 为空集。
其中,generate_candidates 函数用于生成候选项集,calculate_support 函数用于计算项集的支持度。这个算法的核心思想就是利用频繁项集的性质,从小规模的频繁项集逐步生成更大的频繁项集,最终找到所有满足支持度阈值的频繁项集。
需要注意的是,这段代码中的 frequent_itemsets 列表保存的是每个 k 对应的频繁项集,而不是所有频繁项集的集合。因此,最终的频繁项集需要通过取并集的方式得到。
相关问题
# 定义一个函数,用于生成第 k 级候选项集 def generate_candidates(prev_candidates, k): candidates = set() # 对于每一对不同的前缀,将其连接起来生成一个长度为 k 的候选项集 for i in prev_candidates: for j in prev_candidates: if len(i.union(j)) == k: candidates. (i.union(j)) return candidates # 定义 Apriori 算法主函数 def apriori(transactions, support_threshold): # 初始化候选项集 candidates = set() for in transactions: for item in transaction: candidates. (frozenset([item])) # 遍历项集长度从 1 到 N,生成所有频繁项集 freq_itemsets = [] k = 1 while candidates: # 统计候选项集在数据集中出现的次数 counts = {c: 0 for c in candidates} for transaction in transactions: for candidate in candidates: if candidate.issubset(transaction): counts[ ] += 1 # 过滤掉不满足支持度阈值要求的候选项集 freq_candidates=[c for c in candidates if counts[c] / len(transactions) >= ] freq_itemsets. (freq_candidates) # 生成下一级候选项集 k += 1 candidates = (freq_candidates, k) return freq_itemsets # 示例数据集 transactions = [ {'A', 'B', 'C'}, {'A', 'B'}, {'B', 'C'}, {'A', 'B', 'D'}, {'B', 'D'} ] # 调用 Apriori 算法函数 frequent_itemsets = # 输出频繁项集 for itemset in frequent_itemsets: print(itemset)
这是一个 Apriori 算法的 Python 实现,用于挖掘频繁项集。它包括两个函数:generate_candidates 用于生成第 k 级候选项集,apriori 用于实现 Apriori 算法主体。算法的输入是一个事务列表 transactions 和一个支持度阈值 support_threshold,输出是频繁项集列表 frequent_itemsets。
Apriori 算法的主要思想是基于频繁项集的性质,利用候选项集的子集性质进行剪枝,从而减少计算量。算法的流程如下:
1. 初始化候选项集为所有单项集。
2. 遍历项集长度从 1 到 N,生成所有频繁项集。
3. 对于每个候选项集,统计其在数据集中出现的次数。
4. 过滤掉不满足支持度阈值要求的候选项集。
5. 生成下一级候选项集,重复步骤 3-4 直到无法生成更多的频繁项集。
在实现过程中,可以使用 Python 的 set、frozenset 等数据结构来方便地处理项集的交、并、子集等操作。
apriori算法代码
当然,我可以帮你提供 Apriori 算法的示例代码。以下是一个基于 Python 的简单实现:
```python
def apriori(transactions, min_support):
itemsets = find_frequent_1_itemsets(transactions, min_support)
all_frequent_itemsets = itemsets.copy()
k = 2
while itemsets:
itemsets = generate_candidates(itemsets, k)
frequent_itemsets = find_frequent_k_itemsets(itemsets, transactions, min_support)
all_frequent_itemsets.update(frequent_itemsets)
k += 1
return all_frequent_itemsets
def find_frequent_1_itemsets(transactions, min_support):
item_counts = {}
for transaction in transactions:
for item in transaction:
if item in item_counts:
item_counts[item] += 1
else:
item_counts[item] = 1
num_transactions = len(transactions)
frequent_itemsets = {}
for item, count in item_counts.items():
support = count / num_transactions
if support >= min_support:
frequent_itemsets[frozenset([item])] = support
return frequent_itemsets
def generate_candidates(itemsets, k):
candidates = set()
for itemset1 in itemsets:
for itemset2 in itemsets:
union = itemset1.union(itemset2)
if len(union) == k:
candidates.add(union)
return candidates
def find_frequent_k_itemsets(candidates, transactions, min_support):
item_counts = {}
for transaction in transactions:
for candidate in candidates:
if candidate.issubset(transaction):
if candidate in item_counts:
item_counts[candidate] += 1
else:
item_counts[candidate] = 1
num_transactions = len(transactions)
frequent_itemsets = {}
for itemset, count in item_counts.items():
support = count / num_transactions
if support >= min_support:
frequent_itemsets[itemset] = support
return frequent_itemsets
```
使用示例:
```python
transactions = [
['A', 'B', 'D'],
['B', 'C', 'E'],
['A', 'B', 'C', 'E'],
['B', 'E']
]
min_support = 0.5
frequent_itemsets = apriori(transactions, min_support)
print(frequent_itemsets)
```
这段代码实现了 Apriori 算法的关键部分,包括找到频繁 1 项集、生成候选项集、找到频繁 k 项集等步骤。你可以根据自己的数据和需求进行相应的调整和扩展。希望对你有所帮助!
相关推荐
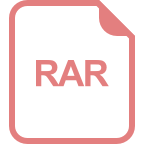












