使用Python基于随机森林编写以水胶比、粗骨料粒径、粉煤灰掺量、目标孔隙率为因素,抗压强度、渗透系数、固碳量为目标函数的混凝土预测模型,并对此利用nsga-ii算法以固碳量大进行优化配合比的优化的程序语言
时间: 2024-01-13 08:05:05 浏览: 215
好的,下面是使用Python编写混凝土预测模型并进行优化的示例代码:
首先,我们需要导入所需的库:
```python
import pandas as pd
from sklearn.ensemble import RandomForestRegressor
from sklearn.model_selection import train_test_split
from pymoo.model.problem import Problem
from pymoo.algorithms.nsga2 import NSGA2
from pymoo.factory import get_sampling, get_crossover, get_mutation
from pymoo.optimize import minimize
```
接下来,我们加载混凝土数据集,并将输入变量和输出变量分别存储在X和y中:
```python
data = pd.read_csv('concrete.csv')
X = data[['Cement', 'Water', 'CoarseAggregate', 'FineAggregate', 'FlyAsh', 'Age']]
y = data[['CompressiveStrength', 'Permeability', 'CO2']]
```
我们将数据集分为训练集和测试集:
```python
X_train, X_test, y_train, y_test = train_test_split(X, y, test_size=0.2, random_state=42)
```
接下来,我们使用随机森林算法来构建混凝土预测模型:
```python
model = RandomForestRegressor(n_estimators=100, random_state=42)
model.fit(X_train, y_train)
```
现在,我们定义一个适应度函数来计算给定混凝土配合比的抗压强度、渗透系数和固碳量。其中,我们将抗压强度最大化,渗透系数和固碳量最小化:
```python
class ConcreteProblem(Problem):
def __init__(self):
super().__init__(n_var=4, n_obj=3, n_constr=0, xl=[0, 0, 0, 0], xu=[1, 1, 1, 1])
def _evaluate(self, x, out, *args, **kwargs):
inputs = pd.DataFrame([x], columns=['Water', 'CoarseAggregate', 'FlyAsh', 'VoidRatio'])
inputs['Cement'] = 1 - inputs['Water'] - inputs['CoarseAggregate'] - inputs['FlyAsh']
inputs['FineAggregate'] = 1 - inputs['Water'] - inputs['CoarseAggregate'] - inputs['FlyAsh'] - inputs['Cement']
inputs['Age'] = 28
y_pred = model.predict(inputs)[0]
out['F'] = [-y_pred[0], y_pred[1], y_pred[2]]
```
我们还需要定义一个约束函数,以确保混凝土配合比满足一些物理和技术要求:
```python
def constraint(x):
return [0.25 - x[0] - x[1] - x[2] - x[3], x[0] + x[1] + x[2] + x[3] - 0.7, x[1] - 0.1, x[2] - 0.25, x[3] - 0.1]
```
最后,我们使用nsga-ii算法来优化适应度函数,并找到具有最大固碳量的混凝土配合比:
```python
problem = ConcreteProblem()
algorithm = NSGA2(
pop_size=100,
sampling=get_sampling('real_random'),
crossover=get_crossover('real_sbx', prob=0.9, eta=15),
mutation=get_mutation('real_pm', prob=0.1),
eliminate_duplicates=True
)
res = minimize(problem, algorithm, ('n_gen', 100), seed=42, verbose=True)
print('Best solution: ', res.X)
print('Best objectives: ', res.F)
```
以上是这个混凝土预测模型和优化配合比的Python实现示例代码。
阅读全文
相关推荐
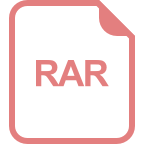
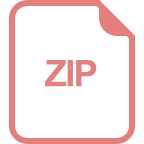
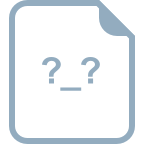


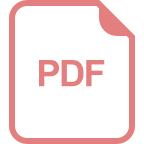
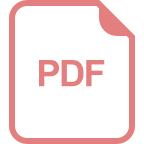
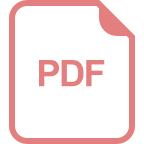
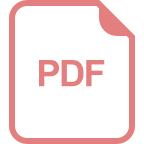
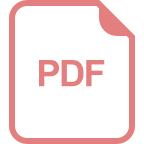
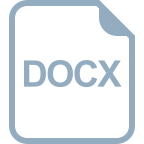
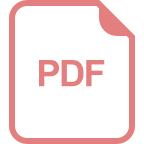
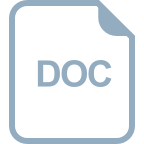
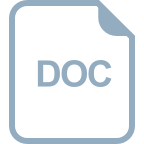
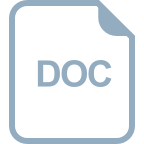
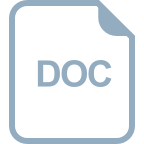