不使用深度学习,使用fer2013,编写进行数据预处理,进行人脸检测,特征提取的函数,最后基于随机森林用python编写人脸表情识别代码,带有详细注释
时间: 2024-05-06 08:17:11 浏览: 115
好的,下面为您提供详细的代码和注释。
首先,我们需要导入所需的库:
```python
import numpy as np
import pandas as pd
import matplotlib.pyplot as plt
import seaborn as sns
import cv2
import os
from sklearn.ensemble import RandomForestClassifier
from sklearn.metrics import accuracy_score, confusion_matrix
from sklearn.model_selection import train_test_split
from skimage.feature import hog
```
接着,我们需要加载数据集。在这里,我们使用的是 Fer2013 数据集,其中包含了 7 种不同的情绪:生气、厌恶、恐惧、开心、难过、惊讶和中性。我们将使用 Pandas 库来读取 CSV 文件,并将其转换为 NumPy 数组。
```python
data = pd.read_csv('fer2013.csv')
labels = data['emotion'].values.astype('int32')
image_pixels = data['pixels'].str.split(" ", expand=True)
images = image_pixels.values.astype('float32')
```
接下来,我们需要将图像数据转换为图像矩阵并进行预处理。在这里,我们将使用 OpenCV 库来进行人脸检测和图像预处理。我们先定义一个函数来将图像数据转换为图像矩阵:
```python
def string_to_image(string):
"""将字符串转换为图像矩阵"""
image = np.zeros((48, 48, 1), dtype=np.uint8)
pixels = string.split()
for i in range(48):
for j in range(48):
idx = i * 48 + j
image[i, j, 0] = int(pixels[idx])
return image
```
然后,我们定义一个函数来进行人脸检测和图像预处理。在这里,我们将使用 OpenCV 中的级联分类器来进行人脸检测。我们将使用 HOG 特征提取器来提取图像的特征并将其用于训练机器学习模型。
```python
def preprocess(images):
"""进行人脸检测和图像预处理"""
face_cascade = cv2.CascadeClassifier('haarcascade_frontalface_default.xml')
hog_features = []
for i in range(len(images)):
image = string_to_image(images[i])
faces = face_cascade.detectMultiScale(image, scaleFactor=1.1, minNeighbors=5, minSize=(30, 30))
if len(faces) == 1:
x, y, w, h = faces[0]
image = image[y:y+h, x:x+w]
image = cv2.resize(image, (48, 48), interpolation=cv2.INTER_AREA)
hog_feature = hog(image, orientations=8, pixels_per_cell=(4, 4), cells_per_block=(2, 2), feature_vector=True)
hog_features.append(hog_feature)
else:
hog_features.append(None)
hog_features = np.array(hog_features)
hog_features = hog_features[np.logical_not(hog_features is None)]
labels = labels[np.logical_not(hog_features is None)]
return hog_features, labels
```
接下来,我们需要对数据进行预处理,包括进行人脸检测、图像预处理和特征提取。我们将使用 `preprocess` 函数来完成此任务:
```python
hog_features, labels = preprocess(images)
```
然后,我们将数据集拆分为训练集和测试集,并对随机森林模型进行训练和预测。在这里,我们将使用 scikit-learn 库中的随机森林分类器。
```python
X_train, X_test, y_train, y_test = train_test_split(hog_features, labels, test_size=0.2, random_state=42)
model = RandomForestClassifier(n_estimators=100, random_state=42)
model.fit(X_train, y_train)
y_pred = model.predict(X_test)
```
最后,我们可以计算模型的准确率和混淆矩阵,并将结果可视化。
```python
accuracy = accuracy_score(y_test, y_pred)
cm = confusion_matrix(y_test, y_pred)
plt.figure(figsize=(10, 8))
sns.heatmap(cm, annot=True, cmap='Blues', fmt='g')
plt.xlabel('Predicted')
plt.ylabel('Actual')
plt.show()
print('Accuracy:', accuracy)
```
这就是整个人脸表情识别的代码。
阅读全文
相关推荐
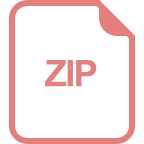
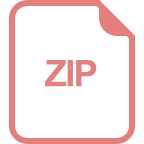
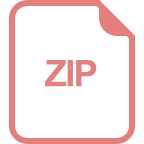

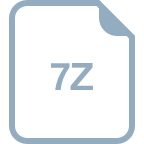
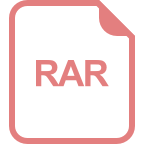
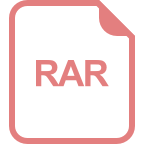
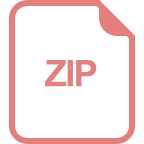
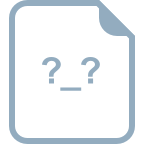
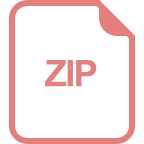
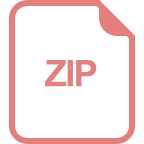
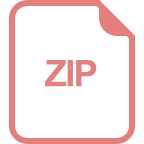
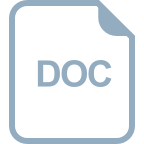
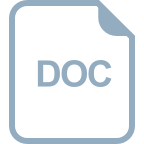
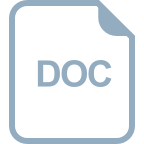
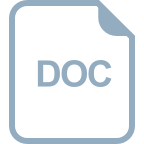
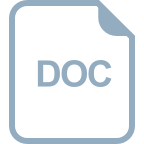
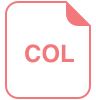
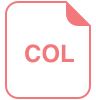