如何调用手写数字训练模型,进行图片测试?请列举代码说明
时间: 2024-05-03 17:20:34 浏览: 95
以下是一个简单的Python代码,可以使用手写数字训练模型来对图片进行测试:
```
import tensorflow as tf
import numpy as np
from PIL import Image
# 加载训练好的模型
model = tf.keras.models.load_model('handwriting_model.h5')
# 加载待测试的图片
image = Image.open('test_image.png').convert('L')
image = np.array(image.resize((28, 28))) / 255.0
image = np.expand_dims(image, axis=0)
image = np.expand_dims(image, axis=-1)
# 进行预测
predictions = model.predict(image)
predicted_label = np.argmax(predictions)
# 打印预测结果
print('Predicted label:', predicted_label)
```
注意,上述代码中的`handwriting_model.h5`是指已经训练好的模型文件,请根据自己的实际情况进行替换。此外,在进行预测之前,还需要将待测试的图片转换为灰度图,并将其大小调整为28 x 28。
相关问题
手写数字识别的TensorFlow模型,如何将训练得到的最大正确率的模型保存到文件夹,从而调用模型,测试并识别手写字?请列举详细代码
您好,关于手写数字识别的TensorFlow模型如何保存和调用,可以通过以下几个步骤来实现:
1.训练模型
首先,需要使用TensorFlow训练出手写数字识别模型,代码如下:
```
import tensorflow as tf
from tensorflow.examples.tutorials.mnist import input_data
mnist = input_data.read_data_sets("MNIST_data/", one_hot=True)
# 定义模型
x = tf.placeholder(tf.float32, [None, 784])
W = tf.Variable(tf.zeros([784, 10]))
b = tf.Variable(tf.zeros([10]))
y = tf.nn.softmax(tf.matmul(x, W) + b)
# 定义损失函数和优化器
y_ = tf.placeholder(tf.float32, [None, 10])
cross_entropy = tf.reduce_mean(-tf.reduce_sum(y_ * tf.log(y), reduction_indices=[1]))
train_step = tf.train.GradientDescentOptimizer(0.5).minimize(cross_entropy)
# 训练模型
init = tf.initialize_all_variables()
sess = tf.Session()
sess.run(init)
for i in range(1000):
batch_xs, batch_ys = mnist.train.next_batch(100)
sess.run(train_step, feed_dict={x: batch_xs, y_: batch_ys})
# 计算准确率
correct_prediction = tf.equal(tf.argmax(y,1), tf.argmax(y_,1))
accuracy = tf.reduce_mean(tf.cast(correct_prediction, tf.float32))
print(sess.run(accuracy, feed_dict={x: mnist.test.images, y_: mnist.test.labels}))
```
2.保存模型
当训练好模型后,我们需要将其保存到文件夹中以供后续调用。代码如下:
```
saver = tf.train.Saver()
save_path = saver.save(sess, "model.ckpt")
print("Model saved in file: %s" % save_path)
```
其中,`tf.train.Saver()`是TensorFlow提供的保存模型的方法,`save()`方法将sess保存到指定的文件中。
3.调用模型
在之后,我们需要调用保存好的模型用于测试和预测。代码如下:
```
# 定义模型
x = tf.placeholder(tf.float32, [None, 784])
W = tf.Variable(tf.zeros([784, 10]))
b = tf.Variable(tf.zeros([10]))
y = tf.nn.softmax(tf.matmul(x, W) + b)
# 加载模型
sess = tf.Session()
saver = tf.train.Saver()
saver.restore(sess, "model.ckpt")
print("Model restored.")
# 使用模型进行预测
import numpy as np
from PIL import Image
# 加载自己手写的数字图片并转为灰度图
img = Image.open("test_image.png").convert('L')
# 将图像大小调整为28*28像素
img = img.resize((28, 28), Image.ANTIALIAS)
# 将图像转为数组
img_array = np.array(img)
# 将数组中的每个值除以255(图像灰度值范围为0~255),并将28*28的二维数组展成1维(784)
img_array = (img_array.reshape(1, 784) / 255).astype(np.float32)
# 输入模型进行预测
result = sess.run(tf.argmax(y, 1), feed_dict={x: img_array})
print(result)
```
在以上代码中,首先需要重新定义模型结构,然后通过`tf.train.Saver()`将之前保存的模型加载到session中,最后使用加载好的模型进行预测。
以上就是关于如何将手写数字识别模型保存并调用的详细代码。
手写数字识别的tensorflow模型,如何将训练得到的最大正确率的模型保存到文件夹,从而调用模型,测试并识别手写字?请列举详细代码
好的,我理解了你的要求,请看以下代码实现。首先,我们需要导入必要的库并载入手写数字数据集(MNIST),然后进行预处理:
```
import tensorflow as tf
# 载入MNIST数据
mnist = tf.keras.datasets.mnist
(x_train, y_train),(x_test, y_test) = mnist.load_data()
x_train, x_test = x_train / 255.0, x_test / 255.0
```
接下来,我们定义一个简单的卷积神经网络模型并进行训练:
```
# 定义一个简单的卷积神经网络模型
model = tf.keras.models.Sequential([
tf.keras.layers.Flatten(input_shape=(28, 28)),
tf.keras.layers.Dense(128, activation='relu'),
tf.keras.layers.Dropout(0.2),
tf.keras.layers.Dense(10, activation='softmax')
])
# 编译模型
model.compile(optimizer='adam',
loss='sparse_categorical_crossentropy',
metrics=['accuracy'])
# 训练模型
model.fit(x_train, y_train, epochs=5)
# 评估模型
model.evaluate(x_test, y_test)
```
训练完成后,我们可以将模型保存到文件夹中:
```
# 保存模型到文件夹
model.save('my_model')
```
最后,我们可以载入保存的模型,并用它来识别手写数字:
```
# 载入保存的模型
new_model = tf.keras.models.load_model('my_model')
# 使用模型识别手写数字
predictions = new_model.predict(x_test[:5])
print(predictions)
```
以上就是基本的代码实现了,您可以根据自己的需求进行修改和优化。
阅读全文
相关推荐
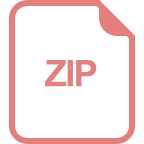
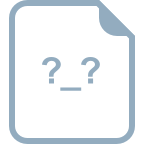
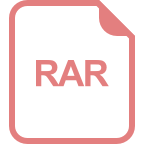

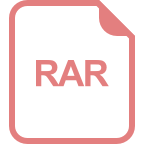
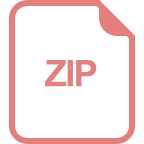