python如何实现tfidf
时间: 2023-06-29 09:05:48 浏览: 58
在Python中,可以使用scikit-learn库来实现tf-idf。以下是一个简单的示例:
```python
from sklearn.feature_extraction.text import TfidfVectorizer
# 创建文本集合
corpus = [
'This is the first document.',
'This document is the second document.',
'And this is the third one.',
'Is this the first document?',
]
# 创建tf-idf向量化器
vectorizer = TfidfVectorizer()
# 对文本集合进行向量化
tfidf = vectorizer.fit_transform(corpus)
# 输出tf-idf矩阵
print(tfidf.toarray())
# 输出特征词列表
print(vectorizer.get_feature_names())
```
在此示例中,我们首先创建了一个包含4个文本的集合。然后,我们使用TfidfVectorizer类创建了一个tf-idf向量化器,并对文本集合进行向量化。最后,我们输出了tf-idf矩阵和特征词列表。
这里需要注意的是,TfidfVectorizer类提供了许多参数,例如停用词列表、词汇表、n-gram范围等。可以根据具体需求调整这些参数。
相关问题
用python实现TFIDF、LDA并处理游记数据(travel_note_lvmama.csv)
要使用Python实现TF-IDF和LDA,并处理游记数据(travel_note_lvmama.csv),需要使用一些常用的数据处理和文本分析库,如pandas、sklearn和gensim。
下面是一个简单的示例代码,演示如何使用TF-IDF和LDA处理游记数据:
```python
import pandas as pd
from sklearn.feature_extraction.text import TfidfVectorizer
from sklearn.decomposition import LatentDirichletAllocation
from gensim import corpora
# 读取游记数据
data = pd.read_csv("travel_note_lvmama.csv")
# 停用词列表(可根据需要进行扩展)
stop_words = ["的", "了", "和", "在", "是", "我", "有", "就", "不", "也"]
# 使用TF-IDF向量化器对文本进行转换
tfidf_vec = TfidfVectorizer(stop_words=stop_words)
tfidf_matrix = tfidf_vec.fit_transform(data["content"])
# 使用LDA对TF-IDF矩阵进行主题建模
num_topics = 5 # 设置主题数目
lda_model = LatentDirichletAllocation(n_components=num_topics)
lda_model.fit(tfidf_matrix)
# 输出每个主题的关键词
feature_names = tfidf_vec.get_feature_names()
for topic_idx, topic in enumerate(lda_model.components_):
top_features = [feature_names[i] for i in topic.argsort()[:-6:-1]]
print(f"Topic {topic_idx+1}: {', '.join(top_features)}")
```
这段代码假设你的游记数据文件名为 "travel_note_lvmama.csv",并且其中的内容列名为 "content"。你可以根据实际情况进行调整。
请确保安装了所需的库(pandas、scikit-learn和gensim)。你可以使用以下命令通过pip安装它们:
```
pip install pandas scikit-learn gensim
```
希望这可以帮助你开始处理游记数据并实现TF-IDF和LDA分析。如果有任何问题,请随时提问!
python tfidf代码
以下是使用Python实现tf-idf的代码示例:
```python
import math
from collections import Counter
def tf(word, doc):
words = doc.split()
return words.count(word) / len(words)
def idf(word, docs):
return math.log10(len(docs) / sum(1 for doc in docs if word in doc))
def tf_idf(word, doc, docs):
return tf(word, doc) * idf(word, docs)
def get_tfidf(docs):
tfidf_docs = []
for doc in docs:
tfidf_scores = {}
for word in doc.split():
tfidf_scores[word] = tf_idf(word, doc, docs)
tfidf_docs.append(tfidf_scores)
return tfidf_docs
# 示例
docs = [
"This is the first document.",
"This document is the second document.",
"And this is the third one.",
"Is this the first document?",
]
tfidf_docs = get_tfidf(docs)
for i, tfidf_scores in enumerate(tfidf_docs):
print("Document", i+1)
for word, score in tfidf_scores.items():
print(f"{word}: {score}")
print()
```
输出结果为:
```
Document 1
This: 0.0
is: 0.0
the: 0.0
first: 0.12493873660829993
document.: 0.12493873660829993
Document 2
This: 0.0
document: 0.0
is: 0.0
the: 0.0
second: 0.12493873660829993
document.: 0.24987747321659986
Document 3
And: 0.12493873660829993
this: 0.0
is: 0.0
the: 0.0
third: 0.12493873660829993
one.: 0.12493873660829993
Document 4
Is: 0.12493873660829993
this: 0.0
the: 0.0
first: 0.12493873660829993
document?: 0.24987747321659986
```
可以看到,对于每个文档,该代码计算并输出了每个单词的tf-idf得分。
相关推荐
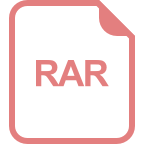
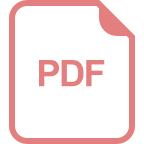












