unction [R] = Muller(f, ~,x0, x1, x2, iter, tol) %MULLER Solving a nonlinear equation with one variable. % Using interpolation with three points to approximate the root. % INPUTS: % x0, x1, x2: initial values (x2 is the best, x0 is the worst) % iter: number of iteration % tol: error tolerance for reltive error % f: left-hand side of the nonlinear equation % OUTPUTS: % x2: the approximate root k = 0; R = {1, 1}; while k<iter && abs((x2-x1)/x1)>tol omega = (f(x2)-f(x1))/(x2-x1); d = f(x0)/((x0-x1)*(x0-x2))+f(x1)/((x1-x0)*(x1-x2))+f(x2)/((x2-x1)*(x2-x0)); omega = omega+d*(x2-x1); x0 = x1; x1 = x2; x2 = x2-2*f(x2)/(omega+sign(omega)*sqrt(omega^2-4*f(x2)*d)); k = k+1; R{1, k} = x2; end R = cell2mat(R); end
时间: 2024-02-03 19:02:02 浏览: 264
这是一个求解非线性方程的 Muller 方法的 MATLAB 函数,它使用三个初始点进行插值来逼近根。
输入参数包括:
- f: 代表非线性方程左侧的函数句柄
- x0, x1, x2: 三个初始点
- iter: 迭代次数
- tol: 相对误差容忍度
输出参数为:
- R: 迭代过程中的所有近似根,以向量形式返回
函数的具体实现过程如下:
1. 初始化迭代参数和近似根:
```
k = 0;
R = {1, 1};
```
2. 在迭代次数和相对误差容忍度内进行循环,更新近似根:
```
while k<iter && abs((x2-x1)/x1)>tol
omega = (f(x2)-f(x1))/(x2-x1);
d = f(x0)/((x0-x1)*(x0-x2))+f(x1)/((x1-x0)*(x1-x2))+f(x2)/((x2-x1)*(x2-x0));
omega = omega+d*(x2-x1);
x0 = x1;
x1 = x2;
x2 = x2-2*f(x2)/(omega+sign(omega)*sqrt(omega^2-4*f(x2)*d));
k = k+1;
R{1, k} = x2;
end
```
3. 将近似根转换为向量并返回:
```
R = cell2mat(R);
```
希望这能帮助您理解该函数的实现过程。
相关问题
unction res = Query(obj,message) % write the query WriteWithoutChecks(obj,message); % read the response res = char(ReadRaw(obj)); % throw away the last byte because it is a \n res = res(1:end-1); end
这段代码是一个 MATLAB 类中的一个方法,其作用是向某个设备发送一个查询指令,然后读取并返回设备的响应结果。
具体来说,它的输入参数是 `obj` 和 `message`,其中 `obj` 是一个 MATLAB 中的对象,`message` 是一个字符串,表示要发送的查询指令。
函数首先调用 `WriteWithoutChecks` 方法向设备写入查询指令,然后调用 `ReadRaw` 方法从设备中读取响应结果。`ReadRaw` 方法返回的是一个 uint8 类型的数组,因此需要将其转换成 char 类型的字符串。
最后,函数将字符串的最后一个字符(通常是一个换行符)去掉,然后将结果返回。
unction Ypred = test_V(V, Sim, X, Y) labelSet = unique(Y); W = construct_W(V, Sim); XW = X * W'; [~, Ypred] = max(XW, [], 2); Ypred = labelSet(Ypred); end
这段代码是一个测试函数 test_V 的实现。它接受参数 V、Sim、X 和 Y,并返回预测结果 Ypred。
首先,通过调用 unique(Y),获取标签集合 labelSet,其中包含 Y 中的唯一标签。
然后,调用 construct_W(V, Sim) 构建权重矩阵 W。构建 W 的具体实现可能在代码的其他部分。
接下来,计算 X 与 W 的乘积,即 XW = X * W',其中 X 是输入数据矩阵,W' 是 W 的转置。
通过调用 max(XW, [], 2),找到每行中的最大值,并返回最大值的索引。即 Ypred = max(XW, [], 2)。
最后,将 Ypred 映射到标签集合 labelSet 中,得到最终的预测结果 Ypred。
请注意,这是对给定代码片段的解释,如果有其他函数或变量定义,请提供更多上下文。
阅读全文
相关推荐
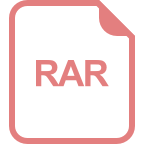
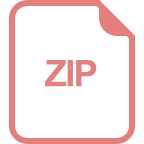
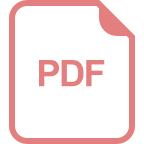













