sklearnroc和auc代码
时间: 2023-09-23 19:06:58 浏览: 80
以下是使用sklearn计算ROC和AUC的示例代码:
```python
from sklearn.metrics import roc_curve, auc
# y_true是真实标签,y_score是预测标签的置信度或概率
fpr, tpr, thresholds = roc_curve(y_true, y_score)
roc_auc = auc(fpr, tpr)
# 绘制ROC曲线
plt.plot(fpr, tpr, label='ROC curve (area = %0.2f)' % roc_auc)
plt.plot([0, 1], [0, 1], 'k--')
plt.xlim([0.0, 1.0])
plt.ylim([0.0, 1.05])
plt.xlabel('False Positive Rate')
plt.ylabel('True Positive Rate')
plt.title('Receiver operating characteristic')
plt.legend(loc="lower right")
plt.show()
```
其中,`y_true`是真实标签,`y_score`是预测标签的置信度或概率。`roc_curve`函数计算出ROC曲线上的点,`auc`函数计算出曲线下的面积(AUC)。最后,使用matplotlib库绘制ROC曲线。
相关问题
matlab AUC代码
以下是一个MATLAB代码示例,用于计算AUC(Area Under the Curve)指标:
```matlab
% 真实标签
trueLabels = [1; 1; 0; 0; 1; 0; 1; 0; 0; 1];
% 预测得分(分类器输出的概率或信号强度)
scores = [0.9; 0.8; 0.7; 0.6; 0.5; 0.4; 0.3; 0.2; 0.1; 0.05];
% 计算排序的索引
[~, sortedIdx] = sort(scores, 'descend');
% 对排序后的标签进行重新排序
sortedLabels = trueLabels(sortedIdx);
% 统计正样本数量和负样本数量
nPositive = sum(trueLabels == 1);
nNegative = sum(trueLabels == 0);
% 计算累积正样本比例和累积负样本比例
cumPositive = cumsum(sortedLabels == 1) / nPositive;
cumNegative = cumsum(sortedLabels == 0) / nNegative;
% 计算AUC
auc = trapz(cumNegative, cumPositive);
disp(auc);
```
在这个示例中,我们首先定义了真实标签(trueLabels)和预测得分(scores),分别表示样本的真实类别和分类器的输出结果。然后,我们根据预测得分对标签进行排序,并计算累积正样本比例和累积负样本比例。最后,使用trapz函数计算AUC(曲线下面积)指标。
请注意,这只是一个简单的示例代码,实际应用中可能需要根据具体情况进行适当的修改和调整。
sklearn 计算auc代码
下面是使用sklearn计算AUC的代码示例:
```python
from sklearn.metrics import roc_auc_score
# 构造样本数据
y_true = [0, 0, 1, 1]
y_scores = [0.1, 0.4, 0.35, 0.8]
# 计算AUC
auc = roc_auc_score(y_true, y_scores)
print('AUC:', auc)
```
其中,`y_true`为真实标签,`y_scores`为预测得分。`roc_auc_score`函数返回的是计算得到的AUC值。
阅读全文
相关推荐
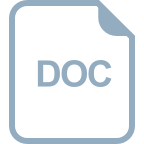
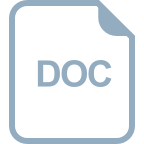
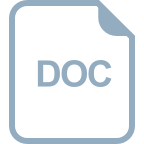













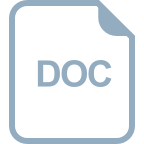