基于tensorflow的交通标志识别
时间: 2023-04-26 09:05:11 浏览: 78
基于TensorFlow的交通标志识别是一种利用TensorFlow深度学习框架进行交通标志分类和识别的技术。通过使用深度学习算法,可以训练神经网络对不同类型的交通标志进行分类和识别,从而实现自动驾驶、智能交通等领域的应用。该技术可以提高交通安全性和交通效率,是未来智能交通领域的重要发展方向之一。
相关问题
基于tensorflow的交通标志识别代码
以下是基于 TensorFlow 的交通标志识别代码示例:
首先,你需要下载交通标志数据集。可以在这里下载:
http://benchmark.ini.rub.de/?section=gtsrb&subsection=dataset
然后,你需要使用 TensorFlow 进行数据预处理和模型训练。下面是一个简单的代码示例:
```python
import tensorflow as tf
# 加载数据集
data = tf.keras.preprocessing.image_dataset_from_directory(
'path/to/dataset',
validation_split=0.2,
subset='training',
seed=123,
image_size=(32, 32),
batch_size=32)
# 数据预处理
normalization_layer = tf.keras.layers.experimental.preprocessing.Rescaling(1./255)
normalized_data = data.map(lambda x, y: (normalization_layer(x), y))
# 构建模型
model = tf.keras.Sequential([
tf.keras.layers.Conv2D(32, kernel_size=(3, 3), activation='relu', input_shape=(32, 32, 3)),
tf.keras.layers.MaxPooling2D(pool_size=(2, 2)),
tf.keras.layers.Conv2D(64, kernel_size=(3, 3), activation='relu'),
tf.keras.layers.MaxPooling2D(pool_size=(2, 2)),
tf.keras.layers.Flatten(),
tf.keras.layers.Dense(128, activation='relu'),
tf.keras.layers.Dropout(0.5),
tf.keras.layers.Dense(43, activation='softmax')
])
# 编译模型
model.compile(optimizer='adam',
loss='sparse_categorical_crossentropy',
metrics=['accuracy'])
# 训练模型
model.fit(normalized_data, epochs=10)
```
上面的代码中,我们使用了卷积神经网络(CNN)来训练交通标志识别模型。模型的输入是一个 32x32 的彩色图像,输出是一个长度为 43 的向量,表示 43 种不同的交通标志类别。我们使用了 ReLU 激活函数和 Dropout 正则化来提高模型的性能。
最后,我们使用 Adam 优化器和交叉熵损失函数来训练模型。在训练过程中,我们使用了 80% 的数据作为训练集,20% 的数据作为验证集,并将数据归一化到 0 到 1 的范围内。
基于tensorflow数据集的交通标志识别实验
很高兴回答您的问题。基于TensorFlow数据集的交通标志识别实验是一项非常有趣的实验。在这个实验中,我们可以使用TensorFlow深度学习框架预处理多个数据集来训练交通标志分类器。这可以帮助我们在交通标志的分类和识别方面取得更好的结果。如果您需要更多关于TensorFlow数据集的信息,请随时向我提问。
相关推荐
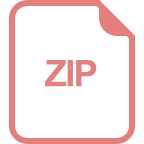
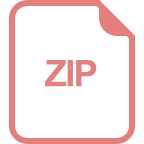
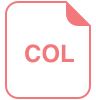
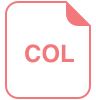
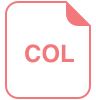









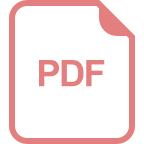