new_careplans_df1 = new_careplans.groupby('PATIENT')['Id'].apply(lambda x:x.str.cat(sep=',')).reset_index() new_careplans_df1['Id'] = new_careplans_df1['Id'].apply(lambda x: x.split(','))
时间: 2023-08-06 19:02:21 浏览: 39
这段代码的作用是将 `new_careplans` 数据集按照 `PATIENT` 进行分组,然后对于每个分组内的 `Id` 列进行处理,将同一分组内的多个 `Id` 以逗号分隔的形式连接起来,形成一个字符串。最后将得到的字符串转化为列表,并将其赋值给新的 `Id` 列。换句话说,这段代码是将同一患者的多个 `Id` 合并为一个列表,并将其保存在新的数据框 `new_careplans_df1` 中的 `Id` 列中。
相关问题
train_x=torch.tensor(df1.values, dtype=torch.float32)
这段代码将一个名为`df1`的Pandas数据框转换为一个PyTorch张量`train_x`。与之前不同的是,这里使用了`.values`方法获取数据框中的数据,并指定了数据类型为`torch.float32`。这样做的好处是可以避免上一个错误,因为`.values`返回的是一个NumPy数组,与PyTorch张量的数据类型兼容。
需要注意的是,这种转换方式只适用于数据框中所有的列都是数值型数据。如果数据框中包含非数值型数据,例如字符串或分类变量,需要先将它们转换为数值型数据,才能将整个数据框转换为张量。
df1 = df1.groupby(keyword_column1, as_index=False)
这是一个对 Pandas DataFrame 进行分组操作的语句,其中 `df1` 是需要进行分组的 DataFrame,`keyword_column1` 是指定的关键列名。这行代码会按照关键列的值对 DataFrame 进行分组,并返回一个分组后的 DataFrame。`as_index=False` 表示返回的 DataFrame 中不将关键列设置为索引。
相关推荐
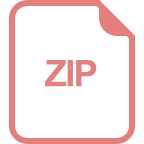












