GCN和注意力结合的代码
时间: 2024-04-08 10:30:51 浏览: 22
下面是一个简单示例的代码,演示了如何将GCN与注意力机制结合起来:
```python
import torch
import torch.nn as nn
class GraphConvolution(nn.Module):
def __init__(self, in_features, out_features):
super(GraphConvolution, self).__init__()
self.linear = nn.Linear(in_features, out_features)
def forward(self, x, adj):
x = self.linear(x)
x = torch.matmul(adj, x) # 邻居节点特征聚合
return x
class GraphAttention(nn.Module):
def __init__(self, in_features, out_features):
super(GraphAttention, self).__init__()
self.linear = nn.Linear(in_features, out_features)
self.attention = nn.Linear(out_features * 2, 1)
def forward(self, x, adj):
x = self.linear(x)
x = torch.matmul(adj, x) # 邻居节点特征聚合
# 注意力权重计算
attention_weights = self.attention(torch.cat([x.repeat(1, adj.size(1), 1), x], dim=2))
attention_weights = torch.softmax(attention_weights, dim=1)
# 加权特征融合
x = torch.matmul(adj.transpose(1, 2), attention_weights * x)
return x
class GCNWithAttention(nn.Module):
def __init__(self, in_features, hidden_features, out_features):
super(GCNWithAttention, self).__init__()
self.gcn1 = GraphConvolution(in_features, hidden_features)
self.gcn2 = GraphAttention(hidden_features, out_features)
def forward(self, x, adj):
x = self.gcn1(x, adj)
x = torch.relu(x)
x = self.gcn2(x, adj)
return x
# 示例数据
x = torch.randn(10, 32) # 节点特征
adj = torch.randn(10, 10) # 邻接矩阵
# 创建模型并进行前向传播
model = GCNWithAttention(32, 64, 128)
output = model(x, adj)
```
以上代码定义了三个模块:GraphConvolution、GraphAttention和GCNWithAttention。GraphConvolution和GraphAttention分别实现了GCN的节点特征更新过程和注意力机制的计算过程。GCNWithAttention则将两者结合起来,构建了一个包含注意力机制的GCN模型。
在forward方法中,首先使用GraphConvolution或GraphAttention对节点特征进行更新和聚合。然后,通过激活函数(如ReLU)处理特征。最后,再次使用GraphAttention对特征进行更新和聚合。最终的输出即为经过GCN和注意力机制处理后的节点特征。
请注意,以上代码只是一个简单示例,实际应用中可能需要根据具体任务和数据的特点进行适当的修改和调整。
相关推荐
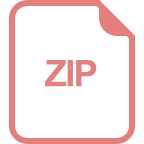














