keras实现prob attention
时间: 2023-07-09 19:21:31 浏览: 48
在Keras中实现Prob Attention可以通过自定义层(Custom Layer)的方式来实现。以下是一个基于Keras的Prob Attention实现代码示例:
```python
import tensorflow as tf
from tensorflow.keras.layers import Layer
class ProbAttention(Layer):
def __init__(self, **kwargs):
super(ProbAttention, self).__init__(**kwargs)
def build(self, input_shape):
self.w = self.add_weight(name='kernel',
shape=(input_shape[2], 1),
initializer='uniform',
trainable=True)
super(ProbAttention, self).build(input_shape)
def call(self, inputs, mask=None):
u = tf.matmul(inputs, self.w)
if mask is not None:
mask = tf.cast(mask, tf.float32)
u -= (1.0 - mask) * 1e12
u = tf.exp(u - tf.reduce_max(u, axis=1, keepdims=True))
s = tf.reduce_sum(u, axis=1, keepdims=True)
alpha = u / s
output = tf.reduce_sum(inputs * alpha, axis=1)
return output
def compute_output_shape(self, input_shape):
return (input_shape[0], input_shape[2])
```
在这个自定义层里,我们将输入张量 `inputs` 与权重张量 `self.w` 做矩阵乘法运算得到一个分数张量 `u`,然后通过对分数张量 `u` 做 Softmax 激活得到一个注意力权重张量 `alpha`,最后将输入张量 `inputs` 和注意力权重张量 `alpha` 做加权求和得到输出张量 `output`。在这个实现中,我们还考虑了一个输入遮盖张量 `mask`,用于将注意力权重张量中遮盖的位置的分数设置为一个极小值,以便在 Softmax 激活后得到一个为零的注意力权重张量。
使用这个自定义层的方式与使用 Keras 常规层相同,只需要将其实例化并将其作为一个层添加到模型中即可。
```python
from tensorflow.keras.layers import Input, Embedding, Masking, Dense, Concatenate
from tensorflow.keras.models import Model
inputs = Input(shape=(maxlen,))
x = Embedding(vocab_size, embedding_dim, mask_zero=True)(inputs)
x = Masking()(x)
x1 = ProbAttention()(x)
x2 = Dense(128, activation='relu')(x1)
outputs = Dense(n_classes, activation='softmax')(x2)
model = Model(inputs=inputs, outputs=outputs)
model.compile(optimizer='adam', loss='categorical_crossentropy', metrics=['accuracy'])
model.summary()
```
相关推荐
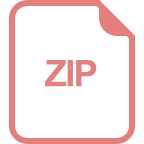
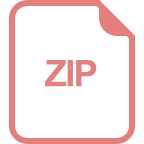
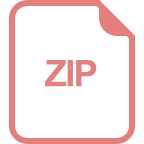














