生成代码 他们首先将图像序列分成若干个时间窗口,对于每个时间窗口,使用YOLO目标检测算法检测出其中的动态物体,并提取出物体的特征向量。然后,对于每个时间窗口中的所有物体特征向量,使用K-means聚类算法对其进行聚类,得到K个聚类中心。最后,对于每个聚类中心,将其视为一类动态物体,并使用静态概率更新策略对其进行跟踪和处理。
时间: 2024-03-17 14:40:48 浏览: 21
以下是一个简单的示例代码,用于实现上述过程:
```python
import cv2
import numpy as np
from yolo import YOLO # 导入YOLO目标检测算法
from sklearn.cluster import KMeans # 导入K-means聚类算法
# 将图像序列分成若干个时间窗口
def split_image_sequence(image_sequence, window_size):
windowed_images = []
for i in range(0, len(image_sequence), window_size):
windowed_images.append(image_sequence[i:i+window_size])
return windowed_images
# 对于每个时间窗口,使用YOLO目标检测算法检测出其中的动态物体,并提取出物体的特征向量
def extract_features_from_window(window, yolo):
features = []
for image in window:
# 使用YOLO目标检测算法检测出其中的动态物体
objects = yolo.detect_objects(image)
for obj in objects:
# 提取出物体的特征向量
feature = extract_feature_from_object(obj)
features.append(feature)
return features
# 提取出物体的特征向量
def extract_feature_from_object(obj):
# TODO: 提取出物体的特征向量
return feature
# 对于每个时间窗口中的所有物体特征向量,使用K-means聚类算法对其进行聚类,得到K个聚类中心
def cluster_features(features, k):
kmeans = KMeans(n_clusters=k)
kmeans.fit(features)
return kmeans.cluster_centers_
# 对于每个聚类中心,将其视为一类动态物体,并使用静态概率更新策略对其进行跟踪和处理
def track_and_process_objects(cluster_centers):
for center in cluster_centers:
# TODO: 对于每个聚类中心,将其视为一类动态物体,并使用静态概率更新策略对其进行跟踪和处理
pass
# 主函数
def main():
# 初始化YOLO目标检测算法
yolo = YOLO()
# 读取图像序列
image_sequence = []
# TODO: 读取图像序列
# 将图像序列分成若干个时间窗口
windowed_images = split_image_sequence(image_sequence, window_size=10)
# 对于每个时间窗口,使用YOLO目标检测算法检测出其中的动态物体,并提取出物体的特征向量
features = []
for window in windowed_images:
features += extract_features_from_window(window, yolo)
# 对于所有物体特征向量,使用K-means聚类算法对其进行聚类,得到K个聚类中心
k = 5
cluster_centers = cluster_features(features, k)
# 对于每个聚类中心,将其视为一类动态物体,并使用静态概率更新策略对其进行跟踪和处理
track_and_process_objects(cluster_centers)
# 释放资源
yolo.close_session()
if __name__ == '__main__':
main()
```
需要注意的是,上述代码仅为示例代码,实际实现中还需要根据具体情况进行修改和完善。
相关推荐
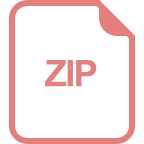
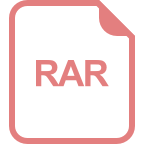
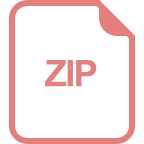














