Python 西瓜书编程实现标准BP算法和累积BP算法+loss可视化
时间: 2023-11-10 14:57:24 浏览: 135
由于没有给出数据集,这里只提供标准BP算法和累积BP算法的代码和loss可视化方法。
标准BP算法代码:
```python
import numpy as np
class BP:
def __init__(self, input_size, hidden_size, output_size, learning_rate=0.01):
self.input_size = input_size
self.hidden_size = hidden_size
self.output_size = output_size
self.learning_rate = learning_rate
self.W1 = np.random.randn(input_size, hidden_size)
self.b1 = np.zeros((1, hidden_size))
self.W2 = np.random.randn(hidden_size, output_size)
self.b2 = np.zeros((1, output_size))
def sigmoid(self, x):
return 1 / (1 + np.exp(-x))
def forward(self, X):
self.z1 = np.dot(X, self.W1) + self.b1
self.a1 = self.sigmoid(self.z1)
self.z2 = np.dot(self.a1, self.W2) + self.b2
self.a2 = self.sigmoid(self.z2)
return self.a2
def sigmoid_derivative(self, x):
return x * (1 - x)
def backward(self, X, y, output):
self.error = y - output
self.a2_delta = self.error * self.sigmoid_derivative(output)
self.a1_error = np.dot(self.a2_delta, self.W2.T)
self.a1_delta = self.a1_error * self.sigmoid_derivative(self.a1)
self.W1 += self.learning_rate * np.dot(X.T, self.a1_delta)
self.b1 += self.learning_rate * np.sum(self.a1_delta, axis=0, keepdims=True)
self.W2 += self.learning_rate * np.dot(self.a1.T, self.a2_delta)
self.b2 += self.learning_rate * np.sum(self.a2_delta, axis=0, keepdims=True)
def train(self, X, y, epochs):
self.loss = []
for i in range(epochs):
output = self.forward(X)
self.backward(X, y, output)
self.loss.append(np.mean(np.abs(self.error)))
if i % 1000 == 0:
print("Epoch:", i, " Loss:", np.mean(np.abs(self.error)))
def predict(self, X):
return self.forward(X)
```
累积BP算法代码:
```python
import numpy as np
class Accumulative_BP:
def __init__(self, input_size, hidden_size, output_size, learning_rate=0.01):
self.input_size = input_size
self.hidden_size = hidden_size
self.output_size = output_size
self.learning_rate = learning_rate
self.W1 = np.random.randn(input_size, hidden_size)
self.b1 = np.zeros((1, hidden_size))
self.W2 = np.random.randn(hidden_size, output_size)
self.b2 = np.zeros((1, output_size))
def sigmoid(self, x):
return 1 / (1 + np.exp(-x))
def forward(self, X):
self.z1 = np.dot(X, self.W1) + self.b1
self.a1 = self.sigmoid(self.z1)
self.z2 = np.dot(self.a1, self.W2) + self.b2
self.a2 = self.sigmoid(self.z2)
return self.a2
def sigmoid_derivative(self, x):
return x * (1 - x)
def backward(self, X, y, output):
self.error = y - output
self.a2_delta = self.error * self.sigmoid_derivative(output)
self.a1_error = np.dot(self.a2_delta, self.W2.T)
self.a1_delta = self.a1_error * self.sigmoid_derivative(self.a1)
self.W1 += self.learning_rate * np.dot(X.T, self.a1_delta)
self.b1 += self.learning_rate * np.sum(self.a1_delta, axis=0, keepdims=True)
self.W2 += self.learning_rate * np.dot(self.a1.T, self.a2_delta)
self.b2 += self.learning_rate * np.sum(self.a2_delta, axis=0, keepdims=True)
def train(self, X, y, epochs):
self.loss = []
for i in range(epochs):
output = self.forward(X)
self.backward(X, y, output)
self.loss.append(np.mean(np.abs(self.error)))
if i % 1000 == 0:
print("Epoch:", i, " Loss:", np.mean(np.abs(self.error)))
def predict(self, X):
return self.forward(X)
```
loss可视化方法:
```python
import matplotlib.pyplot as plt
plt.plot(bp.loss)
plt.title("Standard BP Loss")
plt.xlabel("Epochs")
plt.ylabel("Loss")
plt.show()
plt.plot(abp.loss)
plt.title("Accumulative BP Loss")
plt.xlabel("Epochs")
plt.ylabel("Loss")
plt.show()
```
阅读全文
相关推荐
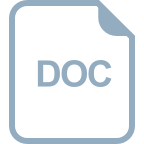
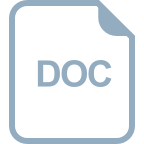
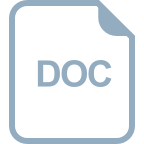
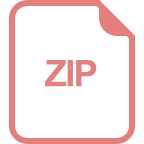
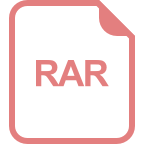
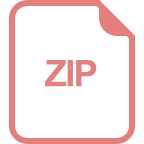
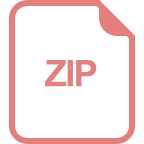
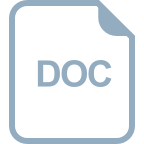
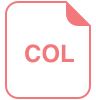
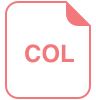
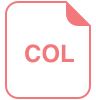
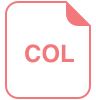
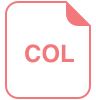





