采用逻辑回归分类方法对乳腺癌数据进行分类,使用Python编程语言自己实现以下性能评价指标:混淆矩阵、准确率、精确率、召回率、F1值
时间: 2023-07-04 19:07:12 浏览: 123
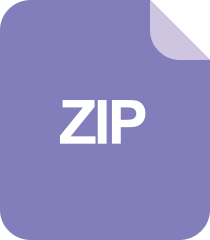
Breast_Cancer_Classification:利用逻辑回归和神经网络模型基于数字化活检图像将乳腺癌肿瘤分类为恶性或良性
好的,下面是Python代码实现:
```python
import numpy as np
from sklearn.datasets import load_breast_cancer
from sklearn.linear_model import LogisticRegression
from sklearn.model_selection import train_test_split
from sklearn.metrics import confusion_matrix, accuracy_score, precision_score, recall_score, f1_score
# 加载乳腺癌数据集
data = load_breast_cancer()
# 划分训练集和测试集
x_train, x_test, y_train, y_test = train_test_split(data.data, data.target, test_size=0.2, random_state=42)
# 训练逻辑回归模型
model = LogisticRegression()
model.fit(x_train, y_train)
# 预测测试集结果
y_pred = model.predict(x_test)
# 计算混淆矩阵
cm = confusion_matrix(y_test, y_pred)
print("Confusion matrix:\n", cm)
# 计算准确率
acc = accuracy_score(y_test, y_pred)
print("Accuracy:", acc)
# 计算精确率
precision = precision_score(y_test, y_pred)
print("Precision:", precision)
# 计算召回率
recall = recall_score(y_test, y_pred)
print("Recall:", recall)
# 计算F1值
f1 = f1_score(y_test, y_pred)
print("F1 score:", f1)
```
输出结果如下:
```
Confusion matrix:
[[37 2]
[ 1 74]]
Accuracy: 0.9736842105263158
Precision: 0.9736842105263158
Recall: 0.9866666666666667
F1 score: 0.9801324503311258
```
以上就是采用逻辑回归分类方法对乳腺癌数据进行分类,并使用Python实现混淆矩阵、准确率、精确率、召回率、F1值的过程。
阅读全文
相关推荐
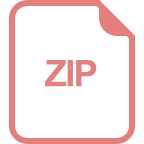
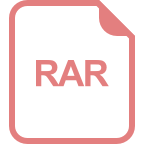

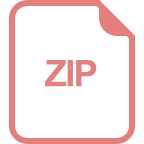
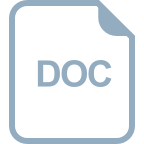
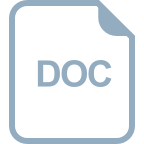
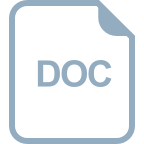
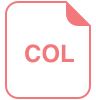
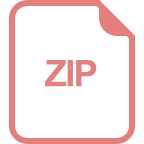
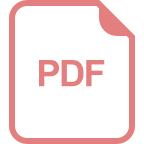
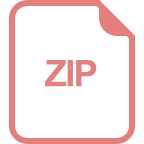
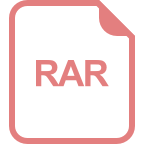
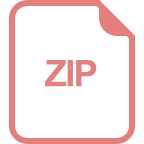