link prediction
时间: 2023-11-07 08:52:14 浏览: 41
链接预测是一种应用于知识图谱中的技术,它将实体和关系映射到连续向量空间中,用于对知识图谱中的实体和关系进行预测。链接预测任务主要包括实体之间的关系预测和实体类型的预测。在实际应用中,链接预测可以用于社交网络中的用户推荐、生物领域中的蛋白质相互作用预测以及对未标记节点类型的预测等多个领域。
在链接预测中,常用的评估指标包括平均等级(Mean Rank)、平均倒数等级(Mean Reciprocal Rank)和命中率前n(Hits@n)。这些指标可以用来评估嵌入模型在链接预测任务上的性能。
链接预测方法多种多样,包括基于相似度的方法、概率统计方法、预处理方法、SVM或KNN等算法。此外,链接预测还可以使用表示学习、神经网络和规则等方法进行推理,以及各种方法的混合使用。
相关问题
link prediction dataset
根据引用,目前主要使用【data/yeast/yeast.edgelist】下的蛋白质数据进行link prediction。根据引用和,在预测link prediction时,可以使用两个不同的预测模型,即GATModelGAN和GATModel。这些模型会返回原始的图邻接矩阵和经过模型编码后的hidden embedding经过内积解码的邻接矩阵。然后可以对这两个矩阵进行比对,得出link prediction结果。因此,link prediction的dataset可以是蛋白质数据集。<span class="em">1</span><span class="em">2</span><span class="em">3</span>
#### 引用[.reference_title]
- *1* *2* *3* [Python实现图神经网络进行link prediction of protein interactions](https://blog.csdn.net/newlw/article/details/122512954)[target="_blank" data-report-click={"spm":"1018.2226.3001.9630","extra":{"utm_source":"vip_chatgpt_common_search_pc_result","utm_medium":"distribute.pc_search_result.none-task-cask-2~all~insert_cask~default-1-null.142^v92^chatsearchT0_1"}}] [.reference_item style="max-width: 100%"]
[ .reference_list ]
seal link prediction
Seal link prediction is a task in machine learning where the goal is to predict the presence or absence of links between two nodes in a graph. Specifically, it involves predicting whether a seal will travel from one location to another, based on various factors such as ocean currents, water temperature, and prey availability. This task has applications in marine conservation and management, as understanding seal movement patterns can help inform decision-making around conservation efforts. Machine learning algorithms such as deep learning and graph neural networks can be used to tackle this task.
相关推荐
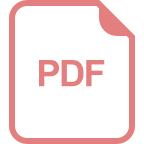












