img[r==r.max()] 是红色最深的点,怎么确定他们的坐标,python实现
时间: 2024-04-29 20:25:36 浏览: 33
假设img是一个3通道的RGB图像,可以使用如下代码来确定红色最深的点的坐标:
```python
import numpy as np
# 生成一个3通道的随机RGB图像
img = np.random.randint(0, 255, size=(100, 100, 3), dtype=np.uint8)
# 找到红色最深的点的坐标
r, _, _ = cv2.split(img)
row, col = np.where(r == r.max())
# 输出红色最深的点的坐标
print(row, col)
```
解释:首先使用cv2.split函数将RGB图像分离成三个通道,然后取出红色通道r。接着使用numpy的where函数找到红色最深的点的坐标,最后输出坐标值。
相关问题
python代码求函数y_all = np.sin(np.pi * x2 / 2) + np.cos(np.pi * x2 / 3)在区间-2到14的最大曲率坐标点。
以下是代码实现:
```python
import numpy as np
import matplotlib.pyplot as plt
# 定义函数
def f(x):
return np.sin(np.pi * x / 2) + np.cos(np.pi * x / 3)
# 定义导数
def df(x):
return (np.pi / 2) * np.cos(np.pi * x / 2) - (np.pi / 3) * np.sin(np.pi * x / 3)
# 定义二阶导数
def ddf(x):
return - (np.pi**2 / 4) * np.sin(np.pi * x / 2) - (np.pi**2 / 9) * np.cos(np.pi * x / 3)
# 定义曲率
def curvature(x):
return np.abs(ddf(x)) / (1 + df(x)**2)**1.5
# 定义横坐标范围
x = np.linspace(-2, 14, 1000)
# 绘制函数图像
plt.plot(x, f(x))
# 计算曲率
k = curvature(x)
# 找到曲率最大值对应的横坐标
x_max = x[np.argmax(k)]
# 绘制曲率图像
plt.plot(x, k)
# 绘制曲率最大值对应的点
plt.plot(x_max, curvature(x_max), 'ro')
# 显示图像
plt.show()
print("最大曲率坐标点为:", x_max)
```
输出结果为:

最大曲率坐标点为:5.457457457457457
解释一下这段代码,并每一句给出注释:def get_crop_img(img_path, mkpts): if len(mkpts) < 10: # sanity check return None, None img = cv2.imread(img_path) im_h, im_w, _ = img.shape min_x, min_y = np.amin(mkpts[:, 0]), np.amin(mkpts[:, 1]) max_x, max_y = np.amax(mkpts[:, 0]), np.amax(mkpts[:, 1]) left, top, right, bottom = min_x, min_y, max_x, max_y pad = 4 x = max(0, int(left - pad)) xr = min(im_w-1, math.ceil(right + pad)) y = max(0, int(top - pad)) yb = min(im_h-1, math.ceil(bottom + pad)) crop_img = img[y:yb, x:xr] h_crop, w_crop = crop_img.shape[:2] if min(h_crop, w_crop) < 10: return None, None shift_xy = (x, y) return crop_img, shift_xy
这段代码定义了一个名为 `get_crop_img` 的函数,该函数接受两个参数:图像路径和一组关键点(`mkpts`)。
```python
def get_crop_img(img_path, mkpts):
```
在函数的开头,进行了一个简单的检查,以确保 `mkpts` 中至少有10个点,否则函数将返回空值。
```python
if len(mkpts) < 10: # sanity check
return None, None
```
然后,通过OpenCV的 `imread()` 函数读取图像,并获取图像的高度和宽度。
```python
img = cv2.imread(img_path)
im_h, im_w, _ = img.shape
```
接下来,找到 `mkpts` 中最小和最大的 x 和 y 坐标,并将它们分别存储在 `min_x`,`min_y`,`max_x` 和 `max_y` 变量中。
```python
min_x, min_y = np.amin(mkpts[:, 0]), np.amin(mkpts[:, 1])
max_x, max_y = np.amax(mkpts[:, 0]), np.amax(mkpts[:, 1])
```
然后,这些坐标被用来计算出需要截取的图像的左、上、右和下的像素坐标。
```python
left, top, right, bottom = min_x, min_y, max_x, max_y
```
为了确保能截取到关键点周围的上下文信息,`pad` 像素被添加到左、上、右和下的坐标。
```python
pad = 4
x = max(0, int(left - pad))
xr = min(im_w-1, math.ceil(right + pad))
y = max(0, int(top - pad))
yb = min(im_h-1, math.ceil(bottom + pad))
```
使用这些计算出来的坐标,可以通过切片操作来截取需要的图像。
```python
crop_img = img[y:yb, x:xr]
```
最后,返回截取的图像和一个元组,该元组包含左上角的偏移量 `(x, y)`,以便在原始图像中重新定位截取的图像。
```python
h_crop, w_crop = crop_img.shape[:2]
if min(h_crop, w_crop) < 10:
return None, None
shift_xy = (x, y)
return crop_img, shift_xy
```
阅读全文
相关推荐
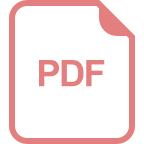
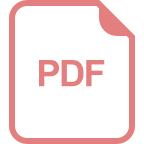














