for _ in range(generate_max_len): outputs = model(input_ids=curr_input_tensor) next_token_logits = outputs[0][-1, :] #size:[vocab size] # 对于已生成的结果generated中的每个token添加一个重复惩罚项,降低其生成概率 for id_ in set(generated): next_token_logits[id_] /= repetition_penalty # 对于[UNK]的概率设为无穷小,也就是说模型的预测结果不可能是[UNK]这个token next_token_logits[tokenizer.convert_tokens_to_ids('[UNK]')] = -float('Inf') filtered_logits = top_k_top_p_filtering(next_token_logits, top_k=topk, top_p=topp) # torch.multinomial表示从候选集合中无放回地进行抽取num_samples个元素,权重越高,抽到的几率越高,返回元素的下标 next_token = torch.multinomial(F.softmax(filtered_logits, dim=-1), num_samples=1) if next_token.item() == tokenizer.sep_token_id: # 遇到[SEP]则表明response生成结束 break generated.append(next_token.item()) curr_input_tensor = torch.cat((curr_input_tensor, next_token), dim=0) text = tokenizer.convert_ids_to_tokens(generated) print("summary:" + "".join(text))这段什么意思
时间: 2024-04-28 21:26:56 浏览: 19
这段代码是用于基于预训练的语言模型生成文本的过程。它使用了top-k和top-p采样的技术,以及一个重复惩罚项,来生成一个在给定前缀下的文本摘要。其中,top-k采样是指从预测的概率分布中选择前k个最高概率的token,top-p采样是指从预测的概率分布中选择累计概率超过一个阈值p的所有token。这样可以增加生成文本的多样性。重复惩罚项是为了防止模型重复生成相同的token。具体来说,对于已经生成的token,它们在下一次生成时的概率会进行一定的惩罚,以降低它们被重复生成的概率。另外,对于[UNK]这个token,模型的预测结果不可能是它,因此它的概率被设为无穷小。最终,生成的文本在遇到[SEP]这个token时结束,输出生成的文本摘要。
相关问题
generate_and_save_image(model=Gen, test_input=test_seed)
这个问题是关于机器学习的,我可以回答。generate_and_save_image(model=Gen, test_input=test_seed) 是一个函数,它的作用是使用生成器模型 (Gen) 和测试输入 (test_seed) 生成一张图片并保存。
def generate_text(self, max_length): text = [] for i in range(max_length): print(self.topK_sampling(5))
这是一个生成文本的函数,它使用了 topK sampling 技术来生成文本。在每次循环中,它会调用 topK_sampling 函数,并将生成的文本添加到一个列表中。循环次数由 max_length 决定。具体实现可以参考下面的代码:
```
def generate_text(self, max_length):
text = []
for i in range(max_length):
generated = self.topK_sampling(5)
text.append(generated)
return " ".join(text)
```
这个函数将生成的文本列表转换成一个字符串,并用空格将其连接起来,最后返回生成的文本。
相关推荐
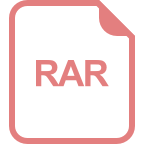
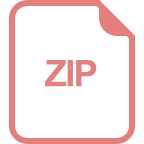












