class Detect(nn.Module): stride = None # strides computed during build onnx_dynamic = False # ONNX export parameter def __init__(self, nc=80, anchors=(), ch=(), inplace=True): # detection layer super().__init__() self.nc = nc # number of classes self.no = nc + 5 # number of outputs per anchor self.nl = len(anchors) # number of detection layers self.na = len(anchors[0]) // 2 # number of anchors self.grid = [torch.zeros(1)] * self.nl # init grid a = torch.tensor(anchors).float().view(self.nl, -1, 2) self.register_buffer('anchors', a) # shape(nl,na,2) self.register_buffer('anchor_grid', a.clone().view(self.nl, 1, -1, 1, 1, 2)) # shape(nl,1,na,1,1,2) self.m = nn.ModuleList(nn.Conv2d(x, self.no * self.na, 1) for x in ch) # output conv self.inplace = inplace # use in-place ops (e.g. slice assignment) def forward(self, x): z = [] # inference output for i in range(self.nl): x[i] = self.m[i](x[i]) # conv bs, _, ny, nx = x[i].shape # x(bs,255,20,20) to x(bs,3,20,20,85) x[i] = x[i].view(bs, self.na, self.no, ny, nx).permute(0, 1, 3, 4, 2).contiguous() if not self.training: # inference if self.grid[i].shape[2:4] != x[i].shape[2:4] or self.onnx_dynamic: self.grid[i] = self._make_grid(nx, ny).to(x[i].device) y = x[i].sigmoid() if self.inplace: y[..., 0:2] = (y[..., 0:2] * 2. - 0.5 + self.grid[i]) * self.stride[i] # xy y[..., 2:4] = (y[..., 2:4] * 2) ** 2 * self.anchor_grid[i] # wh else: # for YOLOv5 on AWS Inferentia https://github.com/ultralytics/yolov5/pull/2953 xy = (y[..., 0:2] * 2. - 0.5 + self.grid[i]) * self.stride[i] # xy wh = (y[..., 2:4] * 2) ** 2 * self.anchor_grid[i].view(1, self.na, 1, 1, 2) # wh y = torch.cat((xy, wh, y[..., 4:]), -1) z.append(y.view(bs, -1, self.no)) return x if self.training else (torch.cat(z, 1), x) @staticmethod def _make_grid(nx=20, ny=20): yv, xv = torch.meshgrid([torch.arange(ny), torch.arange(nx)]) return torch.stack((xv, yv), 2).view((1, 1, ny, nx, 2)).float()
时间: 2023-11-29 15:06:19 浏览: 86
这是一个 PyTorch 中的 Detect 模块的定义。它是 YOLOv5 模型的核心组成部分之一,用于检测图像中的目标,并输出相应的边界框、置信度和类别信息。
具体来说,这个模块包含以下几个主要组成部分:
1. 初始化函数:定义了模块的一些基本参数,如目标的类别数、锚框的数量、输出通道数等。
2. 前向传播函数:接收输入张量 x,经过一系列卷积操作后,输出检测结果。其中,这个函数包含以下几个主要步骤:
a. 对于每个 detection 层,将输入张量 x 经过一个 1x1 的卷积层,得到输出张量;
b. 将输出张量的形状转换为 (batch_size, num_anchors, num_outputs, H, W) 的形式;
c. 对于每个锚框,计算其对应的边界框的位置和置信度等信息;
d. 将不同 detection 层的检测结果拼接起来,得到最终的检测结果。
3. 辅助函数 _make_grid:用于生成网格坐标,用于计算锚框的位置信息。
总的来说,这个 Detect 模块实现了 YOLOv5 检测算法的核心逻辑,是 YOLOv5 模型的重要组成部分之一。
相关问题
class Detect(nn.Module): stride = None # strides computed during build export = False # onnx export #初始化模型的各个属性,并构建模型的卷积层 def __init__(self, nc=80, anchors=(), ch=()): # detection layer super(Detect, self).__init__() self.nc = nc # 标签的数量 self.no = nc + 5 # 计算输出层的节点数 self.nl = len(anchors) #检测层数 self.na = len(anchors[0]) // 2 #每个检测层的锚点数量 self.grid = [torch.zeros(1)] * self.nl # init grid a = torch.tensor(anchors).float().view(self.nl, -1, 2) self.register_buffer('anchors', a) self.register_buffer('anchor_grid', a.clone().view(self.nl, 1, -1, 1, 1, 2)) self.m = nn.ModuleList(nn.Conv2d(x, self.no * self.na, 1) for x in ch)
这段代码定义了 YOLOv5 目标检测模型中的一个子模块——`Detect`。`Detect` 继承了 PyTorch 中的 `nn.Module` 类,并定义了一些属性,如 `stride`、`export` 以及 `nc`、`no`、`nl`、`na`、`grid`、`anchors`、`anchor_grid` 和 `m`。其中,`nc` 表示标签的数量,`anchors` 是一个元组,表示不同检测层的锚点信息,`ch` 表示输入特征图的通道数。在模块初始化时,该代码会计算出每个检测层的锚点数量(`self.na`)和检测层数(`self.nl`),并将锚点信息转换为 PyTorch 的 `Tensor` 格式(`a`)。此外,该代码还会构造一个 `ModuleList` 对象 `self.m`,其中包含了多个 `nn.Conv2d` 模块,用于对输入特征图进行卷积操作,提取目标检测信息。最终,该模块会输出一个包含目标检测信息的张量,用于后续的目标检测任务。
解释代码: def detect_cma(self): # pass model = self.model output_size = self.output_size # source = self.img2predict # file/dir/URL/glob, 0 for webcam imgsz = [640, 640] # inference size (pixels) conf_thres = 0.25 # confidence threshold iou_thres = 0.45 # NMS IOU threshold max_det = 1000 # maximum detections per image # device = self.device # cuda device, i.e. 0 or 0,1,2,3 or cpu view_img = False # show results save_txt = False # save results to *.txt save_conf = False # save confidences in --save-txt labels save_crop = False # save cropped prediction boxes nosave = False # do not save images/videos classes = None # filter by class: --class 0, or --class 0 2 3 agnostic_nms = False # class-agnostic NMS augment = False # ugmented inference visualize = False # visualize features line_thickness = 3 # bounding box thickness (pixels) hide_labels = False # hide labels hide_conf = False # hide confidences half = False # use FP16 half-precision inference dnn = False # use OpenCV DNN for ONNX inference source = str(self.vid_source) device = select_device(self.device) stride, names, pt, jit, onnx = model.stride, model.names, model.pt, model.jit, model.onnx imgsz = check_img_size(imgsz, s=stride) # check image size save_img = not nosave and not source.endswith('.txt') # save inference images
这段代码用于使用 YOLOv5 模型对图像或视频进行目标检测。它首先从类的属性中获取模型、输出大小、视频源等信息。然后设置了一些参数,如推理尺寸、置信度阈值、NMS IOU 阈值等。接着根据设备类型选择使用 CPU 还是 GPU 进行推理。最后,它检查图像的大小是否符合模型要求,设置是否保存推理结果图片,然后返回检测结果。
阅读全文
相关推荐
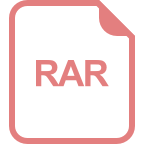
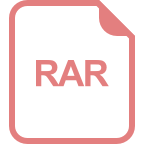
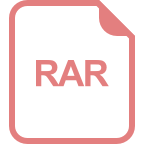

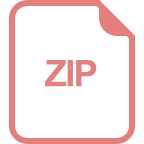
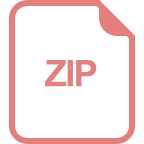
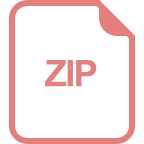
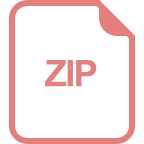
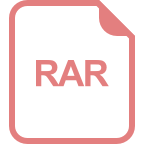
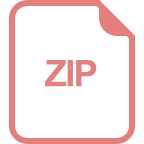
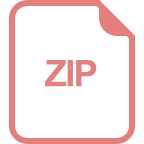
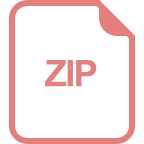
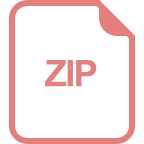
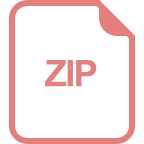
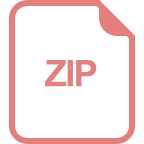
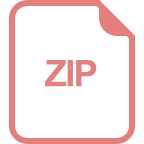
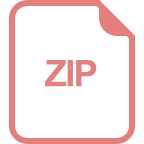
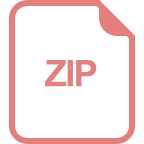