groovy如何string转float
时间: 2023-11-23 07:05:59 浏览: 71
在Groovy中,可以使用 `tofloat()` 方法将字符串转换为浮点数。下面是示例代码:
```groovy
def str = "3.14159"
def floatNum = str.tofloat()
println floatNum
```
输出结果为:
```
3.14159
```
其中,`tofloat()` 方法是Groovy中String类的一个扩展方法,用于将字符串转换为浮点数。在这个示例中,我们定义了一个字符串变量 `str`,并使用 `tofloat()` 方法将其转换为浮点数,然后输出结果。
相关问题
lite-model_movenet_singlepose_lightning_tflite_int8_4.tflite详细的部署在安卓上的代码,测试摄像头视频流
以下是使用 TensorFlow Lite 将 movenet_singlepose_lightning 模型部署在 Android 上并测试摄像头视频流的步骤:
1. 首先,将模型文件 `lite-model_movenet_singlepose_lightning_tflite_int8_4.tflite` 添加到 Android 项目的 assets 文件夹中。
2. 在 build.gradle 文件中添加以下依赖:
```groovy
// TensorFlow Lite
implementation 'org.tensorflow:tensorflow-lite:2.5.0'
```
3. 在 MainActivity 中加载模型文件,并打开摄像头:
```java
public class MainActivity extends AppCompatActivity {
private static final String MODEL_FILE = "lite-model_movenet_singlepose_lightning_tflite_int8_4.tflite";
private static final int IMAGE_SIZE = 192;
private static final int NUM_KEYPOINTS = 17;
private static final int NUM_EDGES = 16;
private static final int[] EDGES = {1, 3, 2, 4, 5, 7, 6, 8, 9, 11, 10, 12, 13, 15, 14, 16};
private CameraView cameraView;
private Interpreter tflite;
private Bitmap inputBitmap;
private Canvas canvas;
private Paint paint;
private float[] output;
private int[] colors;
@Override
protected void onCreate(Bundle savedInstanceState) {
super.onCreate(savedInstanceState);
setContentView(R.layout.activity_main);
// Load the model
try {
ByteBuffer model = loadModelFile(this);
tflite = new Interpreter(model);
} catch (IOException e) {
Log.e("MainActivity", "Failed to load model");
}
// Initialize the camera view
cameraView = findViewById(R.id.camera_view);
cameraView.setLifecycleOwner(this);
cameraView.addFrameProcessor(frame -> {
// Convert the camera frame to a Bitmap
inputBitmap = Bitmap.createBitmap(frame.getSize().getWidth(), frame.getSize().getHeight(), Bitmap.Config.ARGB_8888);
inputBitmap.copyPixelsFromBuffer(frame.getData());
// Preprocess the input image
Bitmap resizedBitmap = Bitmap.createScaledBitmap(inputBitmap, IMAGE_SIZE, IMAGE_SIZE, true);
float[] input = preprocessImage(resizedBitmap);
// Run inference on the input image
output = new float[NUM_KEYPOINTS * 3];
tflite.run(input, output);
// Postprocess the output keypoints
float[] keypoints = postprocessKeypoints(output);
// Draw the keypoints on the input image
canvas = new Canvas(inputBitmap);
paint = new Paint();
colors = new int[NUM_EDGES];
for (int i = 0; i < NUM_EDGES; i++) {
colors[i] = Color.HSVToColor(new float[] {(i * 360f / NUM_EDGES), 1f, 1f});
}
for (int i = 0; i < NUM_KEYPOINTS; i++) {
float x = keypoints[i * 2];
float y = keypoints[i * 2 + 1];
if (x != 0 && y != 0) {
canvas.drawCircle(x, y, 10, paint);
for (int j = 0; j < NUM_EDGES; j++) {
int a = EDGES[j * 2];
int b = EDGES[j * 2 + 1];
float x1 = keypoints[a * 2];
float y1 = keypoints[a * 2 + 1];
float x2 = keypoints[b * 2];
float y2 = keypoints[b * 2 + 1];
if (x1 != 0 && y1 != 0 && x2 != 0 && y2 != 0) {
canvas.drawLine(x1, y1, x2, y2, paint);
}
}
}
}
// Display the output image
runOnUiThread(() -> cameraView.setImageBitmap(inputBitmap));
});
}
private ByteBuffer loadModelFile(Activity activity) throws IOException {
AssetFileDescriptor fileDescriptor = activity.getAssets().openFd(MODEL_FILE);
FileInputStream inputStream = new FileInputStream(fileDescriptor.getFileDescriptor());
FileChannel fileChannel = inputStream.getChannel();
long startOffset = fileDescriptor.getStartOffset();
long declaredLength = fileDescriptor.getDeclaredLength();
return fileChannel.map(FileChannel.MapMode.READ_ONLY, startOffset, declaredLength);
}
private float[] preprocessImage(Bitmap bitmap) {
int[] pixels = new int[IMAGE_SIZE * IMAGE_SIZE];
bitmap.getPixels(pixels, 0, IMAGE_SIZE, 0, 0, IMAGE_SIZE, IMAGE_SIZE);
float[] input = new float[IMAGE_SIZE * IMAGE_SIZE * 3];
for (int i = 0; i < IMAGE_SIZE * IMAGE_SIZE; i++) {
int pixel = pixels[i];
input[i * 3] = ((pixel >> 16) & 0xFF) / 255f;
input[i * 3 + 1] = ((pixel >> 8) & 0xFF) / 255f;
input[i * 3 + 2] = (pixel & 0xFF) / 255f;
}
return input;
}
private float[] postprocessKeypoints(float[] output) {
float[] keypoints = new float[NUM_KEYPOINTS * 2];
for (int i = 0; i < NUM_KEYPOINTS; i++) {
float x = output[i * 3];
float y = output[i * 3 + 1];
float score = output[i * 3 + 2];
keypoints[i * 2] = x * IMAGE_SIZE;
keypoints[i * 2 + 1] = y * IMAGE_SIZE;
}
return keypoints;
}
}
```
在上面的代码中,我们首先在 onCreate 方法中加载模型文件,并初始化摄像头视图。然后,我们在 addFrameProcessor 回调中处理每一帧摄像头输入,并执行以下步骤:
- 将摄像头帧转换为 Bitmap,并将其缩放到模型输入大小。
- 将输入图像预处理为模型输入格式。
- 在模型上运行输入图像的推理。
- 在输出关键点上进行后处理,将它们转换为像素坐标。
- 将关键点绘制到输入图像上,并在屏幕上显示它。
在上面的代码中,我们使用了以下辅助方法:
- `loadModelFile`:从 assets 文件夹中加载模型文件。
- `preprocessImage`:将输入图像预处理为模型输入格式。
- `postprocessKeypoints`:将输出关键点后处理为像素坐标。
注意:为了简化代码,我们只绘制了关键点和连接它们的线段,而没有显示骨架。如果要显示骨架,可以使用更复杂的绘图逻辑。
android 网络图片毛玻璃效果
要在 Android 中对网络图片应用毛玻璃效果,你可以使用 Glide 和 RenderScript 来实现。下面是一个示例代码:
1. 首先,在你的 Android 项目的 `build.gradle` 文件中添加 Glide 的依赖:
```groovy
dependencies {
// ...
implementation 'com.github.bumptech.glide:glide:4.12.0'
annotationProcessor 'com.github.bumptech.glide:compiler:4.12.0'
}
```
2. 创建一个 `GlideBlurTransformation` 类来实现毛玻璃效果的 Glide 转换:
```java
import android.content.Context;
import android.graphics.Bitmap;
import android.renderscript.Allocation;
import android.renderscript.Element;
import android.renderscript.RenderScript;
import android.renderscript.ScriptIntrinsicBlur;
import com.bumptech.glide.load.engine.bitmap_recycle.BitmapPool;
import com.bumptech.glide.load.resource.bitmap.BitmapTransformation;
import java.security.MessageDigest;
public class GlideBlurTransformation extends BitmapTransformation {
private static final String ID = "com.example.app.GlideBlurTransformation";
private static final byte[] ID_BYTES = ID.getBytes(CHARSET);
private Context context;
private float radius;
public GlideBlurTransformation(Context context, float radius) {
this.context = context.getApplicationContext();
this.radius = radius;
}
@Override
protected Bitmap transform(BitmapPool bitmapPool, Bitmap bitmap, int outWidth, int outHeight) {
Bitmap blurredBitmap = bitmap.copy(bitmap.getConfig(), true);
RenderScript renderScript = RenderScript.create(context);
Allocation inputAllocation = Allocation.createFromBitmap(renderScript, bitmap, Allocation.MipmapControl.MIPMAP_FULL, Allocation.USAGE_SHARED);
Allocation outputAllocation = Allocation.createTyped(renderScript, inputAllocation.getType());
ScriptIntrinsicBlur blurScript = ScriptIntrinsicBlur.create(renderScript, Element.U8_4(renderScript));
blurScript.setInput(inputAllocation);
blurScript.setRadius(radius);
blurScript.forEach(outputAllocation);
outputAllocation.copyTo(blurredBitmap);
renderScript.destroy();
return blurredBitmap;
}
@Override
public boolean equals(Object o) {
return o instanceof GlideBlurTransformation;
}
@Override
public int hashCode() {
return ID.hashCode();
}
@Override
public void updateDiskCacheKey(MessageDigest messageDigest) {
messageDigest.update(ID_BYTES);
}
}
```
3. 在你的代码中,使用 Glide 来加载网络图片并应用毛玻璃转换:
```java
String imageUrl = "https://example.com/image.jpg";
ImageView imageView = findViewById(R.id.imageView);
float radius = 10f; // 模糊半径,可以根据需要调整
Glide.with(this)
.load(imageUrl)
.transform(new GlideBlurTransformation(this, radius))
.into(imageView);
```
在上述代码中,我们使用 Glide 来加载网络图片,并通过 `GlideBlurTransformation` 类将其应用毛玻璃转换。你可以根据需要调整模糊半径 `radius` 的值。
请确保在你的 AndroidManifest.xml 文件中添加相应的网络访问权限。
希望以上信息对你有帮助!如果你还有其他问题,请随时提问。
相关推荐
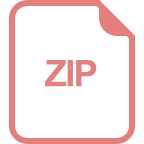








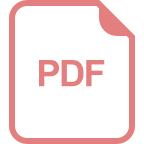
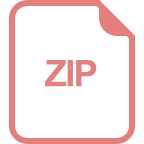