for i, params in enumerate(grid_search.param_grid): print(f"Testing combination {i+1}/{len(grid_search.param_grid)}: {params}") grid_search.fit(X_train, y_train) print(f"Score: {grid_search.best_score_:.3f}\n")
时间: 2024-02-16 15:03:19 浏览: 76
这段代码的作用是进行网格搜索,并在每个参数组合完成验证之后输出评分。具体来说,这段代码的执行过程如下:
1. 使用 `enumerate()` 函数遍历 `grid_search.param_grid` 中的每一个参数组合。`i` 表示当前参数组合的索引,`params` 表示当前参数组合的取值。
2. 输出当前正在测试的参数组合的信息,包括当前参数组合的索引、总共需要测试的参数组合数量,以及当前参数组合的取值。
```
print(f"Testing combination {i+1}/{len(grid_search.param_grid)}: {params}")
```
3. 使用当前参数组合对 `grid_search` 进行拟合,并在训练集和验证集上进行交叉验证。
```
grid_search.fit(X_train, y_train)
```
4. 输出当前参数组合在训练集上的交叉验证分数。
```
print(f"Score: {grid_search.best_score_:.3f}\n")
```
其中,`grid_search.best_score_` 表示当前最优的交叉验证分数。在每个参数组合完成验证之后,我们都会输出当前参数组合的交叉验证分数,以便我们及时了解当前参数组合的效果。
相关问题
x_train, t_train, x_test, t_test = load_data('F:\\2023\\archive\\train') network = DeepConvNet() network.load_params("deep_convnet_params.pkl") print("calculating test accuracy ... ") sampled = 1000 x_test = x_test[:sampled] t_test = t_test[:sampled] prediect_result = [] for i in x_test: i = np.expand_dims(i, 0) y = network.predict(i) _result = network.predict(i) _result = softmax(_result) result = np.argmax(_result) prediect_result.append(int(result)) acc_number = 0 err_number = 0 for i in range(len(prediect_result)): if prediect_result[i] == t_test[i]: acc_number += 1 else: err_number += 1 print("预测正确数:", acc_number) print("预测错误数:", err_number) print("预测总数:", x_test.shape[0]) print("预测正确率:", acc_number / x_test.shape[0]) classified_ids = [] acc = 0.0 batch_size = 100 for i in range(int(x_test.shape[0] / batch_size)): tx = x_test[i * batch_size:(i + 1) * batch_size] tt = t_test[i * batch_size:(i + 1) * batch_size] y = network.predict(tx, train_flg=False) y = np.argmax(y, axis=1) classified_ids.append(y) acc += np.sum(y == tt) acc = acc / x_test.shape[0] classified_ids = np.array(classified_ids) classified_ids = classified_ids.flatten() max_view = 20 current_view = 1 fig = plt.figure() fig.subplots_adjust(left=0, right=1, bottom=0, top=1, hspace=0.2, wspace=0.2) mis_pairs = {} for i, val in enumerate(classified_ids == t_test): if not val: ax = fig.add_subplot(4, 5, current_view, xticks=[], yticks=[]) ax.imshow(x_test[i].reshape(28, 28), cmap=plt.cm.gray_r, interpolation='nearest') mis_pairs[current_view] = (t_test[i], classified_ids[i]) current_view += 1 if current_view > max_view: break print("======= 错误预测结果展示 =======") print("{view index: (label, inference), ...}") print(mis_pairs) plt.show()
这段代码是一个深度卷积神经网络用于对手写数字图像进行分类的代码。首先,通过load_data函数加载训练数据和测试数据集,然后使用DeepConvNet()创建了一个深度卷积神经网络,并通过load_params函数加载了预训练的参数。接着,从测试集中选取了1000个样本进行测试,并用predict函数对每个样本进行预测,将预测结果存储在predict_result列表中。然后,计算预测的正确率,并将错误预测结果展示出来。最后,调用show函数展示错误预测结果。
def get_data_eval(array_folder,raster_file, reference_file): df = TransformCoordonates(raster_file,reference_file) array_folder_list = sorted(os.listdir(array_folder)) array_files = [] array_file_name = [] for n,arr_file in enumerate(array_folder_list): if arr_file.endswith('.npy'): array_files.append(np.load(array_folder+'/'+arr_file,allow_pickle=True)) array_file_name.append(arr_file[:-4]) else: print(arr_file, 'Not Supported') for j,class_ in enumerate(array_files): class_ = np.array(class_, dtype =float) array_val = [] for i in df['position']: try: val = class_[i[0], i[1]] array_val.append(val) except: val = np.nan array_val.append(val) df[array_file_name[j]] = array_val label_list = df['label'].unique() label_class = [] for i in range(0,len(label_list)): label_class.append(i) df['type'] = df['label'].replace(label_list, label_class) df_train = df.drop(['longitude','latitude','label','coordinates','position','row','col'], axis = 1) return df_train, [array_files, array_file_name]
这段代码看起来像是一个函数,函数名为 get_data_eval,有三个参数:array_folder,raster_file,和 reference_file。函数的作用看起来是从一个文件夹中读取Numpy数组数据,然后利用TransformCoordonates函数对这些数组进行坐标变换,并且根据坐标变换后的位置信息从数组中提取对应的值,最终将这些值和相应的标签信息整理成一个DataFrame对象,并返回该对象和原始的数组数据。具体实现过程中,函数使用了os模块对文件夹中的文件进行遍历和筛选,使用了numpy模块对数组数据进行读取和处理,使用了pandas模块对数据进行整合和组织。
阅读全文
相关推荐
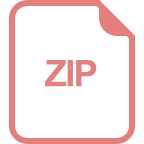
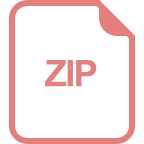
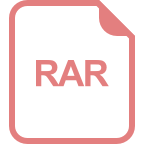













