The human visual cortex is biased towards shape components while CNNs produce texture biased features. This fact may explain why the performance of CNN significantly degrades with low-labeled input data scenarios. In this paper, we propose a frequency re-calibration U-Net (FRCU-Net) for medical image segmentation. Representing an object in terms of frequency may reduce the effect of texture bias, resulting in better generalization for a low data regime. To do so, we apply the Laplacian pyramid in the bottleneck layer of the U-shaped structure. The Laplacian pyramid represents the object proposal in different frequency domains, where the high frequencies are responsible for the texture information and lower frequencies might be related to the shape. Adaptively re-calibrating these frequency representations can produce a more discriminative representation for describing the object of interest. To this end, we first propose to use a channel-wise attention mechanism to capture the relationship between the channels of a set of feature maps in one layer of the frequency pyramid. Second, the extracted features of each level of the pyramid are then combined through a non-linear function based on their impact on the final segmentation output. The proposed FRCU-Net is evaluated on five datasets ISIC 2017, ISIC 2018, the PH2, lung segmentation, and SegPC 2021 challenge datasets and compared to existing alternatives, achieving state-of-the-art results.请详细介绍这段话中的技术点和实现方式
时间: 2024-02-16 14:02:01 浏览: 38
这段话主要介绍了一种针对医学图像分割的新型网络结构——频率重新校准U-Net(FRCU-Net)。该网络结构的核心思想是将物体表示为频率,在低数据情况下降低纹理偏差的影响,从而获得更好的泛化性能。具体实现方式包括以下技术点:
1. 使用Laplacian金字塔将物体表示在不同的频率域中,其中高频率负责纹理信息,而较低的频率可能与物体的形状相关。
2. 通过自适应重新校准这些频率表示,以产生更具有区分性的物体表示。为此,首先提出使用通道注意力机制来捕捉一个特征图集合中各通道之间的关系,然后通过一个非线性函数将金字塔各层的特征提取结果组合起来,以得到最终的分割输出。
3. 在ISIC 2017、ISIC 2018、PH2、肺分割和SegPC 2021数据集上对FRCU-Net进行了评估,并与现有的替代方案进行了比较,取得了最先进的结果。
综上所述,FRCU-Net通过将物体表示为频率,以及使用自适应的频率重新校准和通道注意力机制,实现了对低数据情况下医学图像分割的有效处理。
相关问题
解释这句话If a series contains a stochastic trend, then in finite samples estimated AR coefficients are biased towards 0. The problem for testing for unit root behaviour is that the inferential statistics (e.g. t-ratio) are not normally distributed.
这句话主要是在讨论时间序列的性质。其中,"stochastic trend"指的是一个随机漂移的过程,即时间序列数据的均值随时间的推移而发生变化,但是这种变化是随机的。"AR coefficients"指的是自回归模型中的自回归系数。这句话的意思是,如果一个时间序列包含一个随机漂移的过程,那么在有限的样本中,估计的自回归系数会向0偏移。而对于测试单位根行为(即时间序列是否具有随机漂移的特性)的问题,存在的问题是推断统计量(如t比率)不服从正态分布。简单来说,就是在有随机漂移过程的情况下,时间序列分析中的自回归系数估计会存在偏差,而对于检验随机漂移特性的问题,统计量的分布不服从正态分布,会对推断结果产生影响。
For a given PNP transistorExplain briefly what voltages need to be applied so that this transistor will work in the active (linear) region. Sketch a circuit showing the polarity of your power supplies.
To operate a PNP transistor in the active (linear) region, the following voltages need to be applied:
1. The base-emitter junction should be forward-biased, which means the voltage at the base should be more negative than the voltage at the emitter.
2. The collector-base junction should be reverse-biased, which means the voltage at the collector should be more positive than the voltage at the base.
A simple circuit to demonstrate this would be:
```
+Vcc
|
/ \
/ \ R1
/ \
\ /
\ /
\ /
|/ \| PNP
|
_
|
-
|
0V
```
In this circuit, the positive power supply is connected to the collector of the PNP transistor, and the negative power supply is connected to the emitter. The base is biased with a resistor R1 and a voltage source can be used to set the base voltage. The resistor R1 limits the current flowing into the base to protect the transistor from damage.
By applying the correct voltages to the base, collector, and emitter, the transistor will operate in the active (linear) region and can be used for amplification or switching purposes.
相关推荐
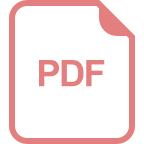
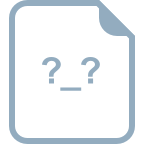
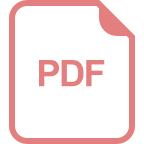












